Publications
Our teams aspire to make discoveries that impact everyone, and core to our approach is sharing our research and tools to fuel progress in the field.
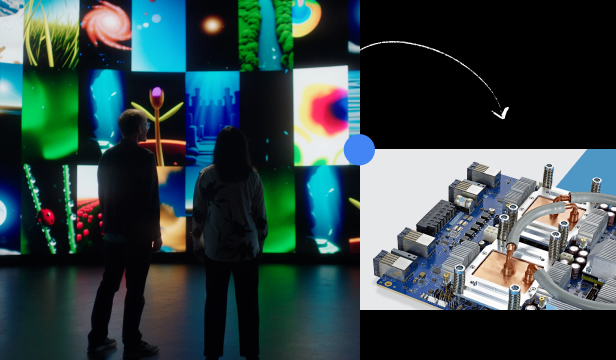
Our teams aspire to make discoveries that impact everyone, and core to our approach is sharing our research and tools to fuel progress in the field.
Sort By
1 - 15 of 10265 publications
Preview abstract
https://www.overleaf.com/project/65ba7d45dae2bce751dba252
Hashing is a fundamental operation in various computer sci-
ence applications. Despite the prevalence of specific key
formats like social security numbers, MAC addresses, plate
numbers, and URLs, hashing libraries typically treat them as
general byte sequences. This paper introduces a technique
for synthesizing specialized hash functions tailored to par-
ticular byte formats. The proposed code generation method
leverages three prevalent patterns: (i) fixed-length keys, (ii)
keys with common subsequences, and (iii) keys ranging on
predetermined sequences of bytes. The code generation pro-
cess involves two algorithms: one identifies relevant regular
expressions within key examples, and the other generates
specialized hash functions based on these expressions. This
approach, straightforward to implement, showcases improve-
ments over highly optimized hash function implementations.
Comparative analysis demonstrates that our synthetic func-
tions outperform counterparts in the C++ Standard Template
Library and the Google Abseil Library, achieving speedups
ranging from 2% to 11%, depending on the key format.
View details
Fast electronic structure quantum simulation by spectrum amplification
Qiushi Han
Dominic Berry
Alec White
Albert Eugene DePrince III
Guang Hao Low
Robbie King
Rolando Somma
arXiv:2502.15882 (2025)
Preview abstract
The most advanced techniques using fault-tolerant quantum computers to estimate the ground-state energy of a chemical Hamiltonian involve compression of the Coulomb operator through tensor factorizations, enabling efficient block-encodings of the Hamiltonian. A natural challenge of these methods is the degree to which block-encoding costs can be reduced. We address this challenge through the technique of spectrum amplification, which magnifies the spectrum of the low-energy states of Hamiltonians that can be expressed as sums of squares. Spectrum amplification enables estimating ground-state energies with significantly improved cost scaling in the block encoding normalization factor $\Lambda$ to just $\sqrt{2\Lambda E_{\text{gap}}}$, where $E_{\text{gap}} \ll \Lambda$ is the lowest energy of the sum-of-squares Hamiltonian. To achieve this, we show that sum-of-squares representations of the electronic structure Hamiltonian are efficiently computable by a family of classical simulation techniques that approximate the ground-state energy from below. In order to further optimize, we also develop a novel factorization that provides a trade-off between the two leading Coulomb integral factorization schemes-- namely, double factorization and tensor hypercontraction-- that when combined with spectrum amplification yields a factor of 4 to 195 speedup over the state of the art in ground-state energy estimation for models of Iron-Sulfur complexes and a CO$_{2}$-fixation catalyst.
View details
Preview abstract
We study the existence of almost fair and near-optimal solutions to a routing problem as defined in the seminal work of Rosenthal. We focus on the setting where multiple alternative routes are available for each potential request (which corresponds to a potential user of the network). This model captures a collection of diverse applications such as packet routing in communication networks, routing in road networks with multiple alternative routes, and the economics of transportation of goods.
Our recommended routes have provable guarantees in terms of both the total cost and fairness concepts such as approximate envy-freeness. We employ and appropriately combine tools from algorithmic game theory and fair division. Our results apply on two distinct models: the splittable case where the request is split among the selected paths (e.g., routing a fleet of trucks) and the unsplittable case where the request is assigned to one of its designated paths (e.g., a single user request). Finally, we conduct an empirical analysis to test the performance of our approach against simpler baselines using the real world road network of New York City.
View details
Mufu: Multilingual Fused Learning for Low- Resource Translation with LLM
Trevor Cohn
Zheng Lim
Honglin Yu
International Conference on Learning Representations (ICLR) 2025
Preview abstract
Multilingual large language models (LLMs) are great translators, but this is largely limited to high-resource languages. For many LLMs, translating in and out of low-resource languages remains a challenging task. To maximize data efficiency in this low-resource setting, we introduce Mufu, which includes a selection of automatically generated multilingual candidates and an instruction to correct inaccurate translations in the prompt. Mufu prompts turn a translation task into a postediting one, and seek to harness the LLM's reasoning capability with auxiliary translation candidates, from which the model is required to assess the input quality, align the semantics cross-lingually, copy from relevant inputs and override instances that are incorrect. Our experiments on En-XX translations over the Flores-200 dataset show LLMs finetuned against Mufu-style prompts are robust to poor quality auxiliary translation candidates, achieving performance superior to NLLB 1.3B distilled model in 64% of low- and very-low-resource language pairs. We then distill these models to reduce inference cost, while maintaining on average 3.1 chrF improvement over finetune-only baseline in low-resource translations.
View details
SMaCk: Efficient Instruction Cache Attacks via Self-Modifying Code Conflicts
Seonghun Son
Berk Gulmezoglu
ACM International Conference on Architectural Support for Programming Languages and Operating Systems (ASPLOS) (2025) (to appear)
Preview abstract
Self-modifying code (SMC) allows programs to alter their own instructions, optimizing performance and functionality on x86 processors. Despite its benefits, SMC introduces unique microarchitectural behaviors that can be exploited for malicious purposes. In this paper, we explore the security implications of SMC by examining how specific x86 instructions affecting instruction cache lines lead to measurable timing discrepancies between cache hits and misses. These discrepancies facilitate refined cache attacks, making them less noisy and more effective. We introduce novel attack techniques that leverage these timing variations to enhance existing methods such as Prime+Probe and Flush+Reload. Our advanced techniques allow adversaries to more precisely attack cryptographic keys and create covert channels akin
to Spectre across various x86 platforms. Finally, we propose a dynamic detection methodology utilizing hardware performance counters to mitigate these enhanced threats.
View details
Preview abstract
The problem of contract design addresses the challenge of moral hazard in principle-agent setups. The agent exerts costly efforts that produce a random outcome with an associated reward for the principal. Moral hazard refers to the tension that the principal cannot observe the agent’s effort level hence needs to incentivize the agent only through rewarding the realized effort outcome, i.e., the contract. Bayesian contract design studies the principal’s design problem of an optimal contract when facing an unknown agent characterized by a private Bayesian type. In its most general form, the agent’s type is inherently “multi-parameter” and can arbitrarily affect both the agent’s productivity and effort costs. In contrast, a natural single-parameter setting of much recent interest simplifies the agent’s type to a single value that describes the agent’s cost per unit of effort, whereas agents’ efforts are assumed to be equally
productive.
The main result of this paper is an almost approximation-preserving polynomial-time reduction from the most general multi-parameter Bayesian contract design (BCD) to single-parameter BCD. That is, for any multi-parameter BCD instance I^M, we construct a single-parameter instance I^S such that any β-approximate contract (resp. menu of contracts) of I^S can in turn be converted to a (β − ϵ)-approximate contract (resp. menu of contracts) of I^M. The reduction is in time polynomial in the input size and log(1/ϵ); moreover, when β = 1 (i.e., the given single-parameter solution is exactly optimal), the dependence on 1/ϵ can be removed, leading to a polynomial-time exact reduction. This efficient reduction is somewhat surprising because in the closely related problem of Bayesian mechanism design, a polynomial-time reduction from multi-parameter to single-parameter setting is believed to not exist. Our result demonstrates the intrinsic difficulty of addressing moral hazard in Bayesian contract design, regardless of being single-parameter or multi-parameter.
As byproducts, our reduction answers two open questions in recent literature of algorithmic contract design: (a) it implies that optimal contract design in single-parameter BCD is not in APX unless P=NP even when the agent’s type distribution is regular, answering the open question of [3] in the negative; (b) it implies that the principal’s (order-wise) tight utility gap between using a menu of contracts and a single contract is Θ(n) where n is the number of actions, answering the major open question of [27] for the single-parameter case.
View details
Gemini & Physical World: Large Language Models Can Estimate the Intensity of Earthquake Shaking from Multi-Modal Social Media Posts
Aman Raj
Marc Stogaitis
Youngmin Cho
Richard Allen
Patrick Robertson
Robert Bosch
Nivetha Thiruverahan
Alexei Barski
Tajinder Gadh
Geophysical Journal International (2025), ggae436
Preview abstract
This paper presents a novel approach for estimating the ground shaking intensity using real-time social media data and CCTV footage. Employing the Gemini 1.5 Pro’s (Reid et al. 2024) model, a multi-modal language model, we demonstrate the ability to extract relevant information from unstructured data utilizing generative AI and natural language processing. The model’s output, in the form of Modified Mercalli Intensity (MMI) values, align well with independent observational data. Furthermore, our results suggest that beyond its advanced visual and auditory understanding abilities, Gemini appears to utilize additional sources of knowledge, including a simplified understanding of the general relationship between earthquake magnitude, distance, and MMI intensity, which it presumably acquired during its training, in its reasoning and decision-making processes. These findings raise intriguing questions about the extent of Gemini's general understanding of the physical world and its phenomena. Gemini’s ability to generate results consistent with established scientific knowledge highlights the potential of LLMs like Gemini in augmenting our understanding of complex physical phenomena such as earthquakes. More specifically, the results of this study highlight the potential of LLMs like Gemini to revolutionize citizen seismology by enabling rapid, effective, and flexible analysis of crowdsourced data from eyewitness accounts for assessing earthquake impact and providing crisis situational awareness. This approach holds a great promise for improving early warning systems, disaster response, and overall resilience in earthquake-prone regions. This study provides a significant step toward harnessing the power of social media and AI for earthquake disaster mitigation.
View details
Beyond Touchscreens: Designing for Co-Occurring Accessibility Needs
Melissa Barnhart Wantland
Mai Kobori
Universal Access in Human-Computer Interaction, Springer-Verlag (2025) (to appear)
Preview abstract
Today’s smartphone interactions are typically designed with one primary preset, accompanied by customization settings that can be manually adjusted. To promote the creation of contextually aware experiences, researchers have highlighted the factors that influence mobile device usage in the ability-based design framework. This paper expands upon existing frameworks and contributes to an empirical understanding of smartphone accessibility. Through a 10-day longitudinal diary study and video interview with 24 individuals who do and do not identify as having a disability, the research also illustrates the reactions of reattempt, adaptation, and avoidance, which were used in response to a lack of smartphone accessibility. Despite experiencing scenarios where accessibility settings could be leveraged, 20 out of 24 participants did not use accessibility settings on their smartphone. A total of 12 out of 24 participants tried accessibility settings on their smartphones, however identifying accessibility was not for them. This work highlights the need to shift current design practices to better serve the accessibility community.
View details
SSDTrain: Faster Large Language Model Training Using SSD-Based Activation Offloading
Mert Hidayetoğlu
Steven Lumetta
Kun Wu
Sitao Huang
Jeongmin Brian Park
Wen-mei Hwu
Vikram Sharma Mailthody
Design Automation Conference (DAC) (2025)
Preview abstract
The scaling up of Large Language Models (LLMs) demands more memory than current GPUs can provide, hindering the training process. To address this challenge, we propose SSDTrain to efficiently offload activations, the intermediate tensors produced during LLM training, to SSDs. This approach reduces GPU memory usage without impacting performance by adaptively overlapping data transfers with computation. SSDTrain is compatible with popular deep learning frameworks like PyTorch, Megatron, and DeepSpeed, and it employs techniques such as tensor deduplication, forwarding, and adaptive offloading to further enhance efficiency. We conduct extensive experiments on Llama, BERT, and T5. Results demonstrate that SSDTrain effectively reduces 45% of the activation peak memory usage. It can perfectly overlap the IO with the computation without introducing performance penalty. SSDTrain can achieve a performance boost of up to 31% compared to the conventional training strategy using the same GPU systems.
View details
AI as a Catalyst for Educational Equity: Addressing Global Teacher Shortages and Learning Disparities
International Journal of Scientific Research in Computer Science, Engineering and Information Technology (IJSRCERT) (2025)
Preview abstract
The global education system is grappling with a critical shortage of teachers, threatening the achievement of universal quality education. This article examines how artificial intelligence (AI) technologies can revolutionize educational access and equity by addressing these systemic challenges. Through a comprehensive article analysis of AI-enabled solutions, including personalized learning mechanisms, virtual tutoring systems, and intelligent content distribution platforms, the article explores the transformative potential of these technologies in democratizing education. The article investigates the implementation of AI across established educational platforms, examining their effectiveness in providing adaptive learning experiences, breaking down language barriers, and ensuring cultural relevance. The article demonstrates that strategic AI integration can significantly impact learning outcomes while helping to bridge the global teacher shortage gap. The article also addresses critical implementation challenges, providing policy recommendations and resource allocation frameworks for successful AI adoption in education systems worldwide. This article analysis contributes to the growing body of knowledge on educational technology by offering practical insights into how AI can be leveraged to create more inclusive, effective, and accessible learning environments, ultimately advancing the goal of quality education for all.
View details
Preview abstract
We consider the Coalition Structure Learning (CSL) problem in multi-agent systems, motivated by the existence of coalitions in many real-world systems, e.g., trading platforms and auction systems. In this problem, there is a hidden coalition structure within a set of $n$ agents, which affects the behavior of the agents in games. Our goal is to actively design a sequence of games
for the agents to play, such that observations in these games can be used to learn the hidden coalition structure. In particular, we consider the setting where in each round, we design and present a game together with a strategy profile to the agents, and receive a multiple-bit observation -- for each agent, we observe whether or not they would like to deviate from the specified strategy in this given game. Our contributions are three-fold: First, we show that we can learn the coalition structure in $O(\log n)$ rounds if we are allowed to choose any normal-form game in each round, matching the information-theoretical lower bound, and the result can be extended to congestion games. Second, in a more restricted setting where we can only choose a graphical game with degree limit $d$, we develop an algorithm to learn the coalition structure in $O(n/d+\log d)$ rounds. Third, when we can only learn the coalition structure through running second-price auctions with personalized reserve prices, we show that the coalition structure can be learned in $O(c\log n)$ rounds, where $c$ is the size of the largest coalition.
View details
Governance, Risk and Compliance (GRC) Engineering: Data, AI, Automation, and the Future of Compliance to Audits
Eric Zhang
Ruchi Khurana
Vikram Khare
2025
Preview abstract
In today's rapidly evolving business landscape, Governance, Risk, and Compliance (GRC) leaders in large, complex organizations face unprecedented challenges. The cloud has revolutionized how businesses operate, offering unprecedented scalability, flexibility, cost-efficiency, additional security and resilience. However, this transformation also presents new challenges for GRC professionals. In a cloud-native world, where applications are built and deployed in dynamic, distributed environments, traditional GRC on-prem approaches, manual processes and spreadsheets struggle to keep pace. The key to success lies in embracing a data-driven GRC strategy that leverages the power of the cloud to enhance agility, visibility, and resilience.
View details
Society-Centric Product Innovation in the Era of Customer Obsession
International Journal of Science and Research Archive (IJSRA), Volume 14 - Issue 1 (2025)
Preview abstract
This article provides a comprehensive analysis of the evolving landscape of innovation in the technology sector, with a focus on the intersection of technological progress and social responsibility. The article explores key challenges facing the industry, including public trust erosion, digital privacy concerns, and the impact of automation on workforce dynamics. It investigates responsible innovation frameworks' emergence and implementation across various organizations, highlighting the transformation from traditional development approaches to more society-centric models. The article demonstrates how companies balance innovation speed with social responsibility, incorporate ethical considerations into their development processes, and address digital disparities across different demographics. By examining how companies balance the pace of innovation with ethical responsibilities, integrate social considerations into their processes, and address digital inequities across diverse demographics, the article underscores the transformative potential of these frameworks. Through insights into cross-functional teams, impact assessment tools, and stakeholder engagement strategies, it demonstrates how responsible innovation drives both sustainable business value and societal progress.
View details
InstructPipe: Building Visual Programming Pipelines in Visual Blocks with Human Instructions Using LLMs
Alex Olwal
Mark Sherwood
Jing Jin
Na Li
Jingtao Zhou
Jun Jiang
Ram Iyengar
Zhongyi Zhou
Yiyi Huang
Kristen Wright
Xiuxiu Yuan
Jason Mayes
Proceedings of the 2025 CHI Conference on Human Factors in Computing Systems (CHI), ACM, pp. 23
Preview abstract
Visual programming provides beginner-level programmers with a coding-free experience to build their customized pipelines. Existing systems require users to build a pipeline entirely from scratch, implying that novice users need to set up and link appropriate nodes all by themselves, starting from a blank workspace. We present InstructPipe, an AI assistant that enables users to start prototyping machine learning (ML) pipelines with text instructions. We designed two LLM modules and a code interpreter to execute our solution. LLM modules generate pseudocode of a target pipeline, and the interpreter renders a pipeline in the node-graph editor for further human-AI collaboration. Technical evaluations reveal that InstructPipe reduces user interactions by 81.1% compared to traditional methods. Our user study (N=16) showed that InstructPipe empowers novice users to streamline their workflow in creating desired ML pipelines, reduce their learning curve, and spark innovative ideas with open-ended commands.
View details
Security Signals: Making Web Security Posture Measurable At Scale
Santiago (Sal) Díaz
David Dworken
Artur Janc
Workshop on Measurements, Attacks, and Defenses for the Web (MADWeb)
Preview abstract
The area of security measurability is gaining increased attention, with a wide range of organizations calling for the development of scalable approaches for assessing the security of software systems and infrastructure. In this paper, we present our experience developing Security Signals, a comprehensive system providing security measurability for web services, deployed in a complex application ecosystem of thousands of web services handling traffic from billions of users. The system collects security-relevant information from production HTTP traffic at the reverse proxy layer, utilizing novel concepts such as synthetic signals augmented with additional risk information to provide a holistic view of the security posture of individual services and the broader application ecosystem. This approach to measurability has enabled large-scale security improvements to our services, including prioritized rollouts of security enhancements and the implementation of automated regression monitoring. Furthermore, it has proven valuable for security research and prioritization of defensive work. Security Signals addresses shortcomings of prior web measurability proposals by tracking a comprehensive set of security properties relevant to web applications, and by extracting insights from collected data for use by both security experts and non-experts. We believe the lessons learned from the implementation and use of Security Signals offer valuable insights for practitioners responsible for web service security, potentially inspiring new approaches to web security measurability.
View details