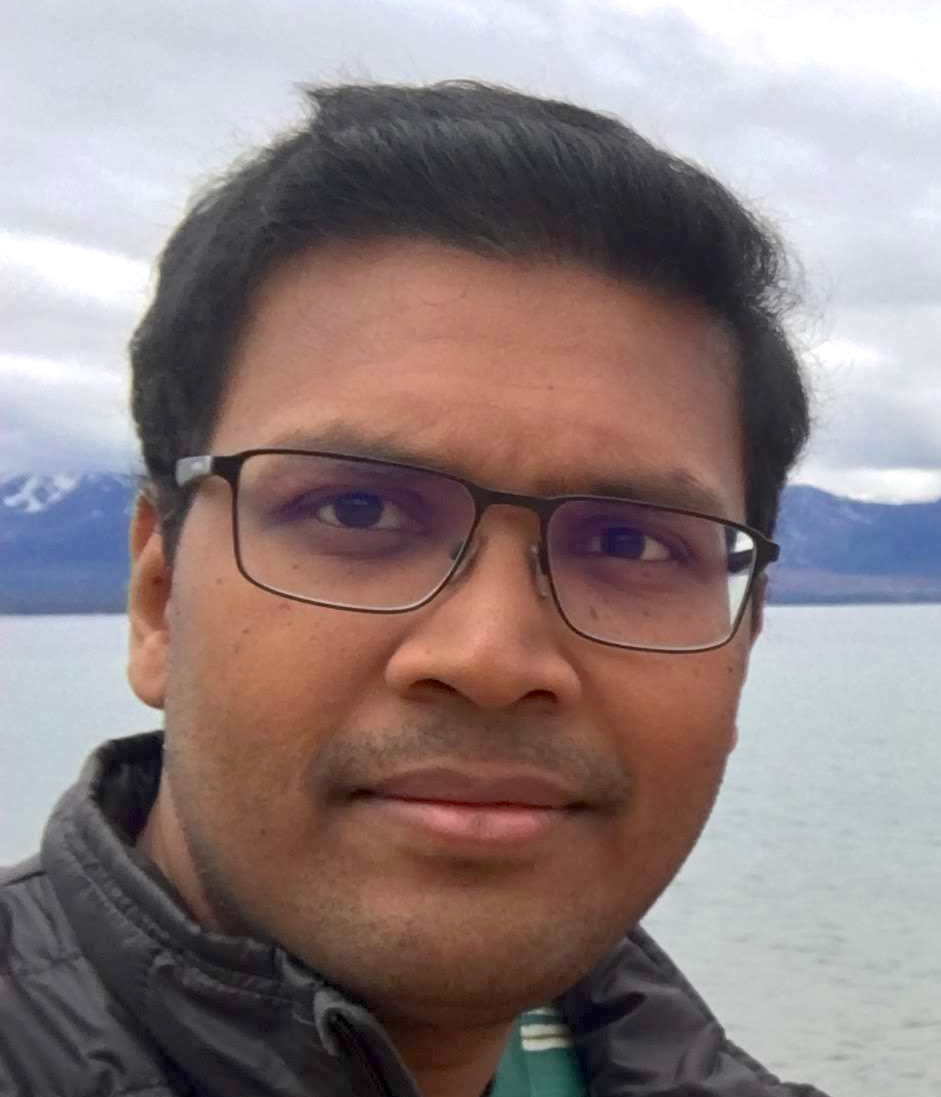
Tanvir Amin
Tanvir Amin is a Software Engineer at Google specializing in Social Networks, Information Retrieval, and Natural Language Processing. Tanvir earned a Ph.D. in Computer Science from the University of Illinois at Urbana-Champaign, where he developed scalable algorithms and systems for real-time summarization and factual extraction of social content streams, addressing challenges such as polarization, bias, and influence. Tanvir led the development of the Apollo Social Sensing Toolkit, SocialTrove, and Polarization Analysis as part of the ARL Network Science CTA program. Tanvir is a member of the editorial board for Frontiers in Big Data and has served on the program committee for the Google Faculty Research Award program and various conferences, including IEEE DCoSS-IOT and ASONAM. Tanvir received the best paper award at IEEE ICAC 2015, best-in-session presentation award at IEEE INFOCOM 2017, and Chirag Foundation Graduate Fellowship in Computer Science (2011-12).
Authored Publications
Sort By
Preview abstract
A vast amount of human discussion, storytelling, content creation,
and reporting now occurs on social media platforms. As such, social
media posts are often quoted on web pages as context. In this
paper, we argue that these quotations and their surrounding page
context provide a rich, platform-independent source of data for
studying the intersection of natural language and social media.
We introduce a taxonomy of quotation roles that categorizes how
social media posts are used within content. We release a dataset
of 38M social quotes derived from the Common Crawl, and role
labels for a subset assessed by human raters. We show that the
interplay of accounts, roles, and topics across the web graph reveal
valuable social diffusion patterns, and that roles can be predicted
with fine-tuned large language models from web context.
View details
Creator Context for Tweet Recommendation
Matt Colen
Sergey Levi
Vladimir Ofitserov
Proceedings of the 2023 Conference on Empirical Methods in Natural Language Processing: Industry Track
Preview abstract
When discussing a tweet, people usually not only refer to the content it delivers, but also to the person behind the tweet. In other words, grounding the interpretation of the tweet in the context of its creator plays an important role in deciphering the true intent and the importance of the tweet.
In this paper, we attempt to answer the question of how creator context should be used to advance tweet understanding. Specifically, we investigate the usefulness of different types of creator context, and examine different model structures for incorporating creator context in tweet modeling. We evaluate our tweet understanding models on a practical use case -- recommending relevant tweets to news articles. This use case already exists in popular news apps, and can also serve as a useful assistive tool for journalists. We discover that creator context is essential for tweet understanding, and can improve application metrics by a large margin. However, we also observe that not all creator contexts are equal. Creator context can be time sensitive and noisy. Careful creator context selection and deliberate model structure design play an important role in creator context effectiveness.
View details
FauxBuster: A Content-free Fauxtography Detector Using Social Media Comments
Daniel Zhang
Lanyu Shang
Biao Geng
Shuyue Lai
Ke Li
Hongmin Zhu
Dong Wang
Proceedings of IEEE BigData 2018 (to appear)
Preview abstract
With the increasing popularity of online social media (e.g., Facebook, Twitter, Reddit), the detection of misleading content on social media has become a critical problem. This paper focuses on an important but largely unsolved problem: detecting fauxtography (i.e., social media posts with misleading images). We found that the existing literature falls short in solving this problem. In particular, current solutions either focus on the detection of fake images or misinformed texts of a social media post. However, they cannot solve our problem because the detection of fauxtography depends not only on the truthfulness of the images and the texts but also on the information they deliver together on the posts. In this paper, we develop the FauxBuster, an end-to-end supervised learning scheme that can effectively track down fauxtography by exploring the valuable clues from user’s comments of a post on social media. The FauxBuster is content-free in that it does not rely on the analysis of the actual content of the images, and hence is robust against sophisticated uploaders who can intentionally modify the description and presentation of the images. We evaluate FauxBuster on real-world datasets collected from two mainstream social media platforms - Reddit and Twitter. The results show our scheme is both effective and efficient in addressing the fauxtography problem.
View details