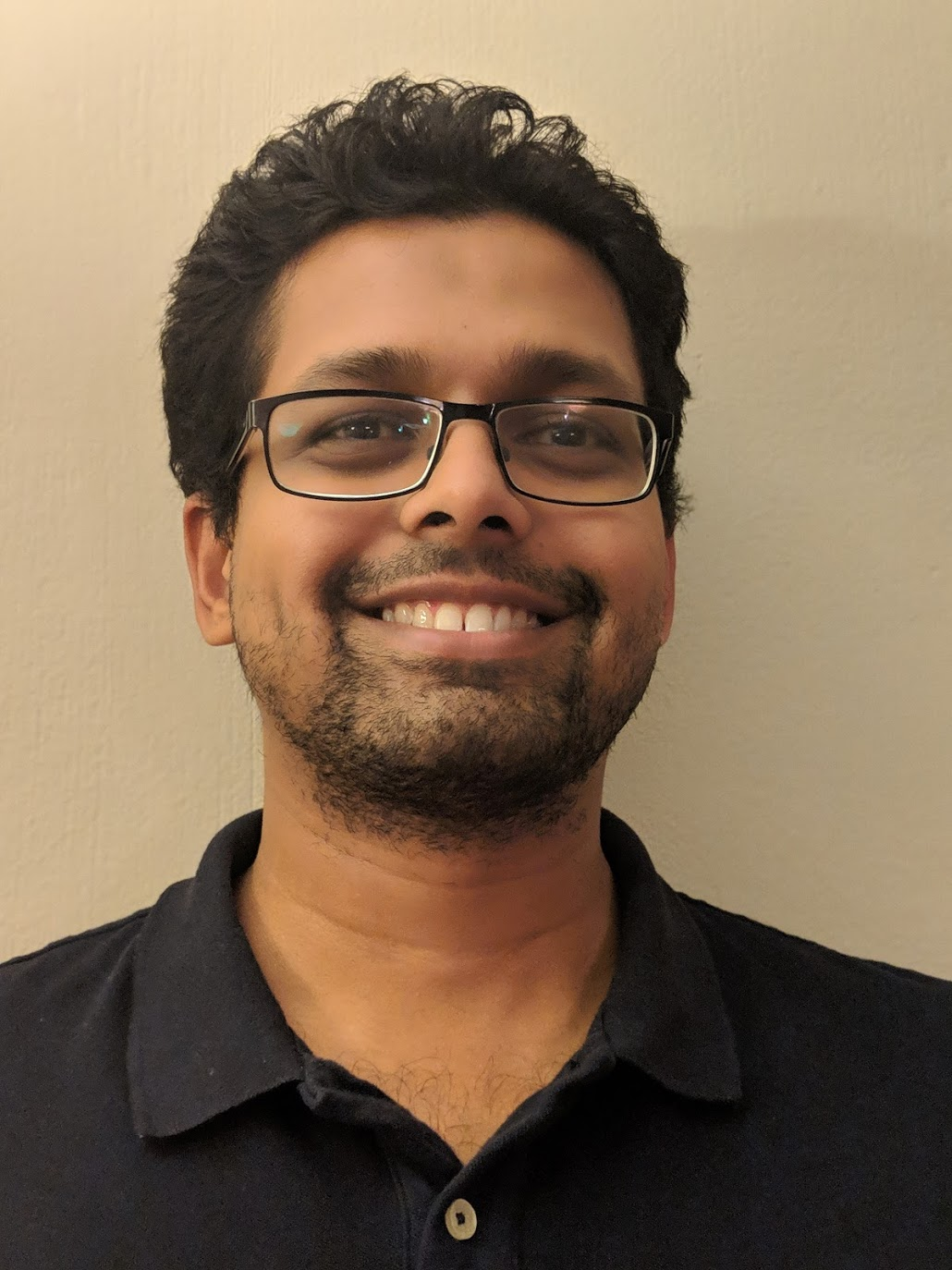
Sourish Chaudhuri
Research Areas
Authored Publications
Sort By
AVA-ActiveSpeaker: An Audio-Visual Dataset for Active Speaker Detection
Ondrej Klejch
Radhika Marvin
Liat Kaver
Sharadh Ramaswamy
Arkadiusz Stopczynski
ICASSP, IEEE(2020)
Preview abstract
Active speaker detection is an important component in video analysis algorithms for applications such as speaker diarization, video re-targeting for meetings, speech enhancement, and human-robot interaction. The absence of a large, carefully labeled audio-visual active speaker dataset has limited evaluation in terms of data diversity, environments, and accuracy. In this paper, we present the AVA Active Speaker detection dataset (AVA-ActiveSpeaker) which has been publicly released to facilitate algorithm development and comparison. It contains temporally labeled face tracks in videos, where each face instance is labeled as speaking or not, and whether the speech is audible. The dataset contains about 3.65 million human labeled frames spanning 38.5 hours. We also introduce a state-of-the-art, jointly trained audio-visual model for real-time active speaker detection and compare several variants. The evaluation clearly demonstrates a significant gain due to audio-visual modeling and temporal integration over multiple frames.
View details
Using audio-visual information to understand speaker activity: Tracking active speakers on and off screen
Ken Hoover
Ian Rutherford Sturdy
Malcolm Slaney
Proceedings of ICASSP, 2018
Preview abstract
We present a system that associates faces with voices in a video by fusing information from the audio and visual signals. The thesis underlying our work is that an extreme simple approach to generating (weak) speech clusters can be combined with strong visual signals to effectively associate faces and voices by aggregating statistics across a video. This approach does not need any training data specific to this task and leverages the natural coherence of information in the audio and visual streams. It is particularly applicable to tracking speakers in videos on the web where a priori information about the environment (e.g., number of speakers, spatial signals for beamforming) is not available.
View details
AVA-Speech: A Densely Labeled Dataset of Speech Activity in Movies
Liat Kaver
Radhika Marvin
Nathan Christopher Reale
Loretta Guarino Reid
Proceedings of Interspeech, 2018
Preview abstract
Speech activity detection (or endpointing) is an important processing step for applications such as speech recognition, language identification and speaker diarization. Both audio- and vision-based approaches have been used for this task in various settings and with multiple variations tailored toward applications. Unfortunately, much of the prior work reports results in synthetic settings, on task-specific datasets, or on datasets that are not openly available. This makes it difficult to compare approaches in similar settings and to understand their strengths and weaknesses. In this paper, we describe a new dataset of densely labeled speech activity in YouTube video clips, which has been designed to address these issues and will be released publicly. The dataset labels go beyond speech alone, annotating three specific speech activity situations: clean speech, speech and music co-occurring, and speech and noise co-occurring. These classes will enable further analysis of model performance in the presence of noise. We report benchmark performance numbers on this dataset using state-of-the-art audio and vision models.
View details
CNN Architectures for Large-Scale Audio Classification
Jort F. Gemmeke
Devin Platt
Malcolm Slaney
International Conference on Acoustics, Speech and Signal Processing (ICASSP), IEEE(2017)
Preview abstract
Convolutional Neural Networks (CNNs) have proven very effective
in image classification and have shown promise for audio classification.
We apply various CNN architectures to audio and investigate
their ability to classify videos with a very large scale data set of 70M
training videos (5.24 million hours) with 30,871 labels. We examine
fully connected Deep Neural Networks (DNNs), AlexNet [1],
VGG [2], Inception [3], and ResNet [4]. We explore the effects of
training with different sized subsets of the 70M training videos. Additionally
we report the effect of training over different subsets of
the 30,871 labels. While our dataset contains video-level labels, we
are also interested in Acoustic Event Detection (AED) and train a
classifier on embeddings learned from the video-level task on AudioSet
[5]. We find that derivatives of image classification networks
do well on our audio classification task, that increasing the number
of labels we train on provides some improved performance over subsets
of labels, that performance of models improves as we increase
training set size, and that a model using embeddings learned from
the video-level task do much better than a baseline on the AudioSet
classification task.
View details