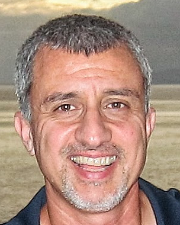
Peyman Milanfar
I lead the Computational Imaging/ Image Processing team in Google Research. My team develops core imaging technologies that are used in a number of products at Google.
One of these technologies is RAISR (Rapid and Accurate Image Super-Resolution): Given an image, we wish to produce an image of larger size with significantly more pixels and higher image quality. With pairs of example images, we train a set of filters (i.e., a mapping) that when applied to a given image that is not in the training set, will produce a higher resolution version of it. The work was highlighted in a Research Blog post. The technology was launched for G+ photos G+ Photos worldwide; and also as part of the MotionStills app .
Another is Turbo Denoising for camera pipelines and other imaging applications. We produced a single-frame denoiser that is (1) fast enough to be practical even for mobile devices, and (2) handles content dependent noise that is typical for real camera captures. For realistic camera noise, our results are competitive with BM3D, but with nearly 400 times speedup. This technique allowed us to speed up denoising algorithm by two orders of magnitude, while producing quality that is state of the art. As a side benefit, less noisy images compress better and lead to smaller file sizes.
Another is Style Transfer which is a process of migrating a style from a given image to the content of another, synthesizing a new image which is an artistic mixture of the two. Our algorithm extends earlier work on texture-synthesis, while aiming to get stylized images that get closer in quality to ones produced by Convolutional Neural Networks. The proposed algorithm is fast and flexible, being able to process any pair of content + style images .
My team also works on more theoretical questions. For instance, in RED (Regularization by Denoising) we proposed a new way to use the denoising engine in defining the regularization for any inverse problem. RED is an explicit image-adaptive Laplacian-based regularization functional, making the overall objective functional clear and well-defined. With a complete flexibility to choose the iterative optimization procedure for minimizing the above functional, RED is capable of incorporating any image denoising algorithm, treat general inverse problems very effectively, and is guaranteed to converge to the globally optimal result. As examples of its utility, we test this approach and demonstrate state-of-the-art results in the image deblurring and super-resolution problems.
A bit about my background: Prior to joining Google, I was a Professor of Electrical Engineering at UC Santa Cruz from 1999-2014. I was also Associate Dean for Research at the School of Engineering from 2010-12. From 2012-2014 I was on leave at Google-x, where I helped develop the imaging pipeline for Google Glass. I received my undergraduate education in electrical engineering and mathematics from the University of California, Berkeley, and the MS and PhD degrees in electrical engineering from MIT. I hold 11 US patents, several of which are commercially licensed. He founded MotionDSP in 2005. I've been keynote speaker at numerous technical conferences including Picture Coding Symposium (PCS), SIAM Imaging Sciences, SPIE, and the International Conference on Multimedia (ICME). Along with my former students, I won several best paper awards from the IEEE Signal Processing Society.
I am a Distinguished Lecturer of the IEEE Signal Processing Society, and a Fellow of the IEEE "for contributions to inverse problems and super-resolution in imaging."
Please visit my public website, for the most up to date list of my publications, cv, etc.
One of these technologies is RAISR (Rapid and Accurate Image Super-Resolution): Given an image, we wish to produce an image of larger size with significantly more pixels and higher image quality. With pairs of example images, we train a set of filters (i.e., a mapping) that when applied to a given image that is not in the training set, will produce a higher resolution version of it. The work was highlighted in a Research Blog post. The technology was launched for G+ photos G+ Photos worldwide; and also as part of the MotionStills app .
Another is Turbo Denoising for camera pipelines and other imaging applications. We produced a single-frame denoiser that is (1) fast enough to be practical even for mobile devices, and (2) handles content dependent noise that is typical for real camera captures. For realistic camera noise, our results are competitive with BM3D, but with nearly 400 times speedup. This technique allowed us to speed up denoising algorithm by two orders of magnitude, while producing quality that is state of the art. As a side benefit, less noisy images compress better and lead to smaller file sizes.
Another is Style Transfer which is a process of migrating a style from a given image to the content of another, synthesizing a new image which is an artistic mixture of the two. Our algorithm extends earlier work on texture-synthesis, while aiming to get stylized images that get closer in quality to ones produced by Convolutional Neural Networks. The proposed algorithm is fast and flexible, being able to process any pair of content + style images .
My team also works on more theoretical questions. For instance, in RED (Regularization by Denoising) we proposed a new way to use the denoising engine in defining the regularization for any inverse problem. RED is an explicit image-adaptive Laplacian-based regularization functional, making the overall objective functional clear and well-defined. With a complete flexibility to choose the iterative optimization procedure for minimizing the above functional, RED is capable of incorporating any image denoising algorithm, treat general inverse problems very effectively, and is guaranteed to converge to the globally optimal result. As examples of its utility, we test this approach and demonstrate state-of-the-art results in the image deblurring and super-resolution problems.
A bit about my background: Prior to joining Google, I was a Professor of Electrical Engineering at UC Santa Cruz from 1999-2014. I was also Associate Dean for Research at the School of Engineering from 2010-12. From 2012-2014 I was on leave at Google-x, where I helped develop the imaging pipeline for Google Glass. I received my undergraduate education in electrical engineering and mathematics from the University of California, Berkeley, and the MS and PhD degrees in electrical engineering from MIT. I hold 11 US patents, several of which are commercially licensed. He founded MotionDSP in 2005. I've been keynote speaker at numerous technical conferences including Picture Coding Symposium (PCS), SIAM Imaging Sciences, SPIE, and the International Conference on Multimedia (ICME). Along with my former students, I won several best paper awards from the IEEE Signal Processing Society.
I am a Distinguished Lecturer of the IEEE Signal Processing Society, and a Fellow of the IEEE "for contributions to inverse problems and super-resolution in imaging."
Please visit my public website, for the most up to date list of my publications, cv, etc.
Research Areas
Authored Publications
Sort By
DVMark: A Deep Multiscale Network for Video Watermarking
Huiwen Chang
Ce Liu
IEEE Transactions on Image Processing (2023)
Preview abstract
Video watermarking embeds a message into a cover video in an imperceptible manner, which can be retrieved even if the video undergoes certain modifications or distortions. Traditional watermarking methods are often manually designed for particular types of distortions and thus cannot simultaneously handle a broad spectrum of distortions. To this end, we propose a robust deep learning-based solution for video watermarking that is end-to-end trainable. Our model consists of a novel multiscale design where the watermarks are distributed across multiple spatial-temporal scales. Extensive evaluations on a wide variety of distortions show that our method outperforms traditional video watermarking methods as well as deep image watermarking models by a large margin. We further demonstrate the practicality of our method on a realistic video-editing application.
View details
SVDiff: Compact Parameter Space for Diffusion Fine-Tuning
Ligong Han
Han Zhang
Dimitris Metaxas
IEEE/CVF International Conference on Computer Vision (ICCV) (2023)
Preview abstract
Diffusion models have achieved remarkable success in text-to-image generation, enabling the creation of high-quality images from text prompts or other modalities. However, existing methods for customizing these models are limited by handling multiple personalized subjects and the risk of overfitting. Moreover, their large number of parameters is inefficient for model storage. In this paper, we propose a novel approach to address these limitations in existing text-to-image diffusion models for personalization. Our method involves fine-tuning the singular values of the weight matrices, leading to a compact and efficient parameter space that reduces the risk of overfitting and language-drifting. We also propose a Cut-Mix-Unmix data-augmentation technique to enhance the quality of multi-subject image generation and a simple text-based image editing framework. Our proposed SVDiff method has a significantly smaller model size (1.7MB for StableDiffusion) compared to existing methods (vanilla DreamBooth 3.66GB, Custom Diffusion 73MB), making it more practical for real-world applications.
View details
Preview abstract
Inversion by Direct Iteration (InDI) is a new formulation for supervised image restoration that avoids the so-called ``regression to the mean'' effect and produces more realistic and detailed images than existing regression-based methods. It does this by gradually improving image quality in small steps, similar to generative denoising diffusion models.
Image restoration is an ill-posed problem where multiple high-quality images are plausible reconstructions of a given low-quality input. Therefore, the outcome of a single step regression model is typically an aggregate of all possible explanations, therefore lacking details and realism. The main advantage of InDI is that it does not try to predict the clean target image in a single step but instead gradually improves the image in small steps, resulting in better perceptual quality.
While generative denoising diffusion models also work in small steps, our formulation is distinct in that it does not require knowledge of any analytic form of the degradation process. Instead, we directly learn an iterative restoration process from low-quality and high-quality paired examples. InDI can be applied to virtually any image degradation, given paired training data. In conditional denoising diffusion image restoration the denoising network generates the restored image by repeatedly denoising an initial image of pure noise, conditioned on the degraded input. Contrary to conditional denoising formulations, InDI directly proceeds by iteratively restoring the input low-quality image, producing high-quality results on a variety of image restoration tasks, including motion and out-of-focus deblurring, super-resolution, compression artifact removal, and denoising.
View details
Soft Diffusion: Score Matching with General Corruptions
Giannis Daras
Alexandros Dimakis
Transactions on Machine Learning Research (TMLR) (2023)
Preview abstract
We define a broader family of corruption processes that generalizes previously known diffusion models. To reverse these general diffusions, we propose a new objective called Soft Score Matching. Soft Score Matching incorporates the degradation process in the network and provably learns the score function for any linear corruption process. Our new loss trains the model to predict a clean image, that after corruption, matches the diffused observation. This objective learns the gradient of the likelihood under suitable regularity conditions for the family of linear corruption processes. We further develop an algorithm to select the corruption levels for general diffusion processes and a novel sampling method that we call Momentum Sampler. We show experimentally that our framework works for general linear corruption processes, such as Gaussian blur and masking. Our method outperforms all linear diffusion models on CelebA-64 achieving FID score 1.85. We also show computational benefits compared to vanilla denoising diffusion.
View details
Interpretable Unsupervised Diversity Denoising and Artefact Removal
Mangal Prakash
Florian Jug
International Conference on Learning Representations (2022)
Preview abstract
Image denoising and artefact removal are complex inverse problems admitting multiple valid solutions. Unsupervised diversity restoration, that is, obtaining a diverse set of possible restorations given a corrupted image, is important for ambiguity removal in many applications such as microscopy where paired data for supervised training are often unobtainable. In real world applications, imaging noise and artefacts are typically hard to model, leading to unsatisfactory performance of existing unsupervised approaches. This work presents an interpretable approach for unsupervised and diverse image restoration. To this end, we introduce a capable architecture called Hierarchical DivNoising (HDN) based on hierarchical Variational Autoencoder. We show that HDN learns an interpretable multi-scale representation of artefacts and we leverage this interpretability to remove imaging artefacts commonly occurring in microscopy data. Our method achieves state-of-the-art results on twelve benchmark image denoising datasets while providing access to a whole distribution of sensibly restored solutions.
Additionally, we demonstrate on three real microscopy datasets that HDN removes artefacts without supervision, being the first method capable of doing so while generating multiple plausible restorations all consistent with the given corrupted image.
View details
Deep 3D-to-2D Watermarking: Embedding Messages in 3D Meshes and Extracting Them from 2D Renderings
Ce Liu
Huiwen Chang
Innfarn Yoo
Ondrej Stava
Computer Vision and Pattern Recognition (2022)
Preview abstract
Digital watermarking is widely used for copyright protection. Traditional 3D watermarking approaches or commercial software are typically designed to embed messages into 3D meshes, and later retrieve the messages directly from distorted/undistorted watermarked 3D meshes. However, in many cases, users only have access to rendered 2D images instead of 3D meshes. Unfortunately, retrieving messages from 2D renderings of 3D meshes is still challenging and underexplored. We introduce a novel end-toend learning framework to solve this problem through: 1) an encoder to covertly embed messages in both mesh geometry and textures; 2) a differentiable renderer to render watermarked 3D objects from different camera angles and under varied lighting conditions; 3) a decoder to recover
the messages from 2D rendered images. From our experiments, we show that our model can learn to embed information visually imperceptible to humans, and to retrieve the embedded information from 2D renderings that undergo 3D distortions. In addition, we demonstrate that our method can also work with other renderers, such as ray tracers and real-time renderers with and without fine-tuning.
View details
Deblurring via Stochastic Refinement
Jay Whang
Chitwan Saharia
Alexandros Dimakis
CVPR (2022)
Preview abstract
Image deblurring is an ill-posed problem with multiple plausible solutions given a single input image. However, most existing methods produce a deterministic estimate of the clean image and are trained to minimize pixel-level distortion. These metrics are known to be poorly correlated with human perception, and often lead to unrealistic reconstructions.
We present an alternative framework for single-image blind deblurring based on conditional diffusion models. Unlike existing techniques, we train a stochastic sampler that refines the output of a deterministic predictor and is capable of producing a diverse set of plausible reconstructions for a single input. This leads to a significant improvement in perceptual quality over existing state-of-the-art methods across multiple standard benchmarks. Our predict-and-refine approach also enables much more efficient sampling compared to the standard diffusion model. Combined with a carefully tuned network architecture and inference procedure, our method is shown to be competitive in terms of traditional quantitative distortion metrics such as PSNR. These results show clear benefits of stochastic diffusion-based methods for deblurring and challenge the widely used strategy of producing a single, deterministic reconstruction.
View details
MAXIM: Multi-Axis MLP for Image Processing
Zhengzhong Tu
Han Zhang
Alan Bovik
IEEE/CVF Conference on Computer Vision and Pattern Recognition (2022)
Preview abstract
Recent progress on Transformers and MLP-like models has shown new architecture design paradigms on many computer vision tasks. However, efficacy and efficiency of these models for low-level vision tasks have not been studied extensively. In this paper, we present MAXIM, a general image processing architecture with multi-axis gated MLPs, to advance the possibility of global operators for low-level vision. Our single-stage MAXIM backbone shares a UNet-shaped hierarchy structure and enjoys a long-range interaction brought by spatial-gated MLPs. Specifically, MAXIM contains two MLP-based building blocks. First, we devise a multi-axis gated MLP that allows efficient and scalable spatial mixing of local and global information. Second, we propose a cross-gating block, an alternative to cross-attention, which accounts for cross-example mutual conditioning. Both modules are exclusively based on MLPs, but benefit from being both global and `fully-convolutional,' two desired properties for low-level vision tasks. Our extensive experimental results show that our proposed MAXIM model achieves state-of-the-art performance on more than ten benchmarks across a range of image processing tasks including denoising, deblurring, deraining, dehazing, and enhancement with less or comparable parameters and FLOPs.
View details
MaxViT: Multi-Axis Vision Transformer
Zhengzhong Tu
Han Zhang
Alan Bovik
European Conference on Computer Vision (ECCV) (2022)
Preview abstract
Transformers have recently gained significant attention in the computer vision community. However, the lack of scalability of self-attention mechanisms with respect to image size has limited their wide adoption in state-of-the-art vision backbones. In this paper we introduce an efficient and scalable attention model we call multi-axis attention, which consists of two aspects: blocked local and dilated global attention. These design choices allow global-local spatial interactions on arbitrary input resolutions with only linear complexity. We also present a new architectural element by effectively blending our proposed attention model with convolutions, and accordingly propose a simple hierarchical vision backbone, dubbed MaxViT, by simply repeating the basic building block over multiple stages. Notably, MaxViT is able to “see” globally throughout the entire network, even in earlier, high-resolution stages. We demonstrate the effectiveness of our model on a broad spectrum of vision tasks. On image classification, MaxViT achieves state-of-the-art performance under various settings: without extra data, MaxViT attains 86.5% ImageNet-1K top-1 accuracy; with ImageNet-21K pre-training,
our model achieves 88.7% top-1 accuracy. For downstream tasks, MaxViT as a backbone delivers favorable performance on object detection as well as visual aesthetic assessment. We also show that our proposed model expresses strong generative modeling capability on ImageNet, demonstrating the superior potential of MaxViT blocks as a universal vision module. The source code and trained models will be available at https://github.com/google-research/maxvit.
View details
Preview abstract
JPEG is an old yet popular image compression format, sup-ported by all imaging devices and software packages. A key ingredientgoverning its performance are the two quantization tables (for Luma andChroma) that dictate the loss induced on each DCT coefficient. Pastwork has offered various ideas for better tuning these tables, mainly fo-cusing on rate-distortion performance and using derivative-free optimiza-tion techniques. This work offers a novel optimal tuning of these tablesvia continuous optimization, leveraging a differential implementation ofboth the JPEG encoder-decoder and an entropy estimator. This enablesus to offer a unified framework that considers the interplay between fourperformance measures: rate, distortion, perceptual quality, and classi-fication accuracy. We also propose a deep-neural network design thatautomatically assigns optimized quantization tables to each incomingimage. In all these fronts, we report a substantial boost in performanceby a simple and easily implemented modification of these tables.
View details