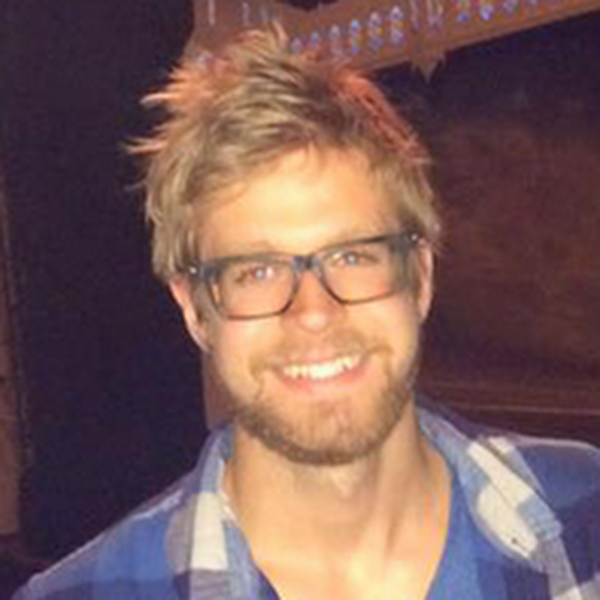
Per Karlsson
Authored Publications
Sort By
Preview abstract
With a proliferation of generic domain-adaptation approaches, we report a simple yet effective technique for learning difficult per-pixel 2.5D and 3D regression representations of articulated people. We obtained strong sim-to-real domain generalization for the 2.5D DensePose estimation task and the 3D human surface normal estimation task. On the multi-person DensePose MSCOCO benchmark, our approach outperforms the state-of-the-art methods which are trained on real images that are densely labelled. This is an important result since obtaining human manifold's intrinsic $uv$ coordinates on real images is time consuming and prone to labeling noise. Additionally, we present our model's 3D surface normal predictions on the MSCOCO dataset that lacks any real 3D surface normal labels. The key to our approach is to mitigate the ``Inter-domain Covariate Shift" with a carefully selected training batch from a mixture of domain samples, a deep batch-normalized residual network, and a modified multi-task learning objective. Our approach is complementary to existing domain-adaptation techniques and can be applied to other dense per-pixel pose estimation problems.
View details
Preview abstract
We present a method that learns to integrate temporal information, from a learned
dynamics model, with ambiguous visual information, from a learned vision model,
in the context of interacting agents. Our method is based on a graph-structured
variational recurrent neural network (Graph-VRNN), which is trained end-to-end
to infer the current state of the (partially observed) world, as well as to forecast
future states. We show that our method outperforms various baselines on two sports
datasets, one based on real basketball trajectories, and one generated by a soccer
game engine.
View details