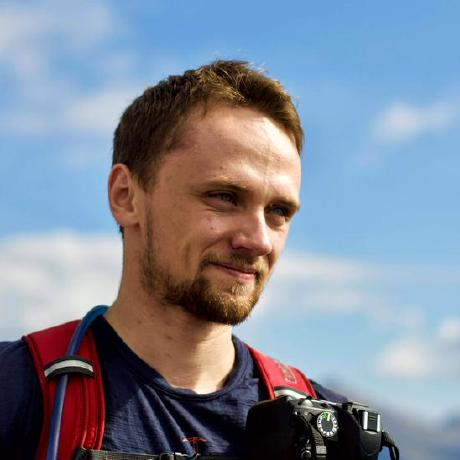
Ondrej Skopek
Research Areas
Authored Publications
Sort By
Towards Better Evaluation of Instruction-Following: A Case-Study in Summarization
Rahul Aralikatte
Sian Gooding
Proceedings of the 27th Conference on Computational Natural Language Learning (CoNLL), Association for Computational Linguistics(2023)
Preview abstract
Despite recent advances, evaluating how well large language models (LLMs) follow user instructions remains an open problem. While evaluation methods of language models have seen a rise in prompt-based approaches, limited work on the correctness of these methods has been conducted.
In this work, we perform a meta-evaluation of a variety of metrics to quantify how accurately they measure the instruction-following abilities of LLMs. Our investigation is performed on grounded query-based summarization by collecting a new short-form, real-world dataset riSum, containing 300 document-instruction pairs with 3 answers each. All 900 answers are rated by 3 human annotators. Using riSum, we analyze the agreement between evaluation methods and human judgment.
Finally, we propose new LLM-based reference-free evaluation methods that improve upon established baselines and perform on par with costly reference-based metrics that require high-quality summaries.
View details
REPLACING HUMAN-RECORDED AUDIO WITH SYNTHETIC AUDIOFOR ON-DEVICE UNSPOKEN PUNCTUATION PREDICTION
Bogdan Prisacari
Daria Soboleva
Felix Weissenberger
Justin Lu
Márius Šajgalík
ICASSP 2021: International Conference on Acoustics, Speech and Signal Processing(2021) (to appear)
Preview abstract
We present a novel multi-modal unspoken punctuation prediction system for the English language, which relies on Quasi-Recurrent Neural Networks (QRNNs) applied jointly on the text output from automatic speech recognition and acoustic features.
%
We show significant improvements from adding acoustic features compared to the text-only baseline. Because annotated acoustic data is hard to obtain, we demonstrate that relying on only 20% of human-annotated audio and replacing the rest with synthetic text-to-speech (TTS) predictions, does not suffer from quality loss on LibriTTS corpus.
%
Furthermore, we demonstrate that through data augmentation using TTS models, we can remove human-recorded audio completely and outperform models trained on it.
View details