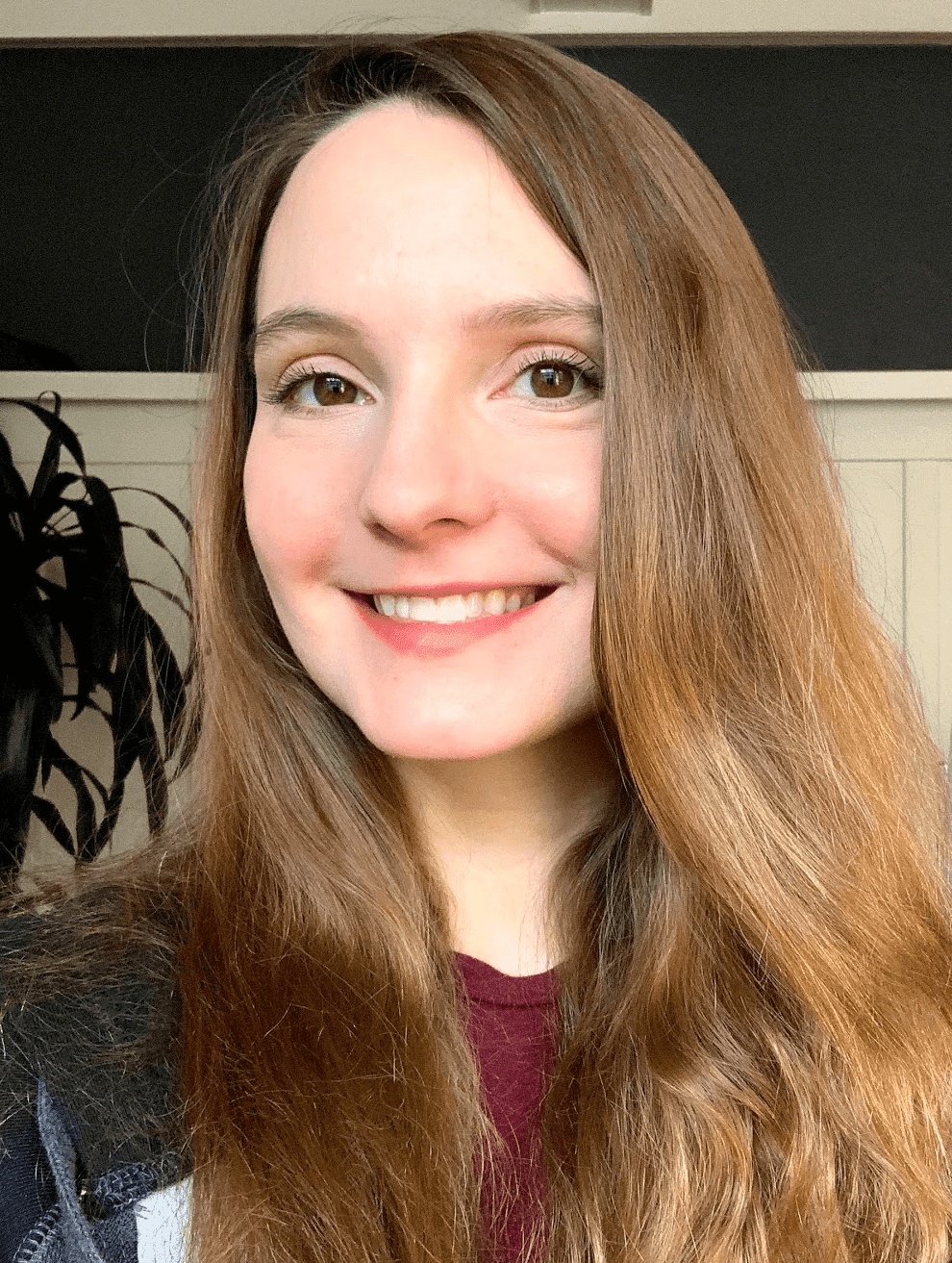
Natasha Jaques
Natasha Jaques holds a joint position as a Research Scientist at Google Brain and post-doc at UC Berkeley. Her research focuses on social reinforcement learning---developing multi-agent RL algorithms that can improve single-agent learning, generalization, coordination, and human-AI collaboration. Natasha received her PhD from MIT, where she worked on Affective Computing and deep/reinforcement/machine learning. Her work has received the best demo award at NeurIPS 2016, best paper at the NeurIPS workshops on ML for Healthcare and Cooperative AI, and an honourable mention for best paper at ICML 2019. She has interned at DeepMind, Google Brain, and is an OpenAI Scholars mentor. Her work has been featured in Quartz, the MIT Technology Review, Boston Magazine, and on CBC radio. Natasha earned her Masters degree from the University of British Columbia, and undergraduate degrees in Computer Science and Psychology from the University of Regina.
See all publications at: https://scholar.google.com/citations?user=8iCb2TwAAAAJ
Authored Publications
Sort By
Google
Less is More: Generating Grounded Navigation Instructions from Landmarks
Su Wang
Jordi Orbay
Vighnesh Birodkar
Izzeddin Gur
Peter Anderson
CVPR (2022) (to appear)
Joint Attention for Multi-Agent Coordination and Social Learning
Dennis Lee
Jiaxing Wu
ICRA Workshop on Social Intelligence in Humans and Robots (2021)
Environment Generation for Zero-Shot Compositional Reinforcement Learning
Izzeddin Gur
Yingjie Miao
Jongwook Choi
Manoj Tiwari
Honglak Lee
Thirty-Fifth Conference on Neural Information Processing Systems (NeurIPS) (2021)
PsiPhi-Learning: Reinforcement Learning with Demonstrations using Successor Features and Inverse Temporal Difference Learning
Angelos Filos
Clare Lyle
Yarin Gal
Sergey Levine
Greg Farquhar
International Conference on Machine Learning (2021)
Emergent Social Learning via Multi-agent Reinforcement Learning
Kamal Ndousse
Sergey Levine
International Conference on Machine Learning (ICML) (2021)
Emergent Complexity and Zero-shot Transfer via Unsupervised Environment Design
Michael Dennis*
Eugene Vinitsky
Alexandre Bayen
Stuart Russell
Andrew Critch
Sergey Levine
Neural Information Processing Systems (2020)
Learning via Social Awareness: Improving a Deep Generative Sketching Model with Facial Feedback
Jennifer McCleary
David Ha
Fred Bertsch
Rosalind Picard
International Joint Conference on Artificial Intelligence (IJCAI) 2018 (2020), pp. 1-9
Human-centric dialog training via offline reinforcement learning
Judy Hanwen Shen
Craig Ferguson
Agata Lapedriza
Noah Jones
Shixiang Gu
Rosalind Picard
Empirical Methods in Natural Language Processing (EMNLP) (2020)
Social Influence as Intrinsic Motivation for Multi-Agent Deep Reinforcement Learning
Angeliki Lazaridou
Edward Hughes
Caglar Gulcehre
Pedro A. Ortega
DJ Strouse
Joel Z Leibo
Nando de Freitas
ICML 2019 (2019)
Learning via Social Awareness: Improving a Deep Generative Sketching Model with Facial Feedback
Jennifer McCleary
David Ha
Fred Bertsch
Rosalind Picard
ICLR 2018 Workshop