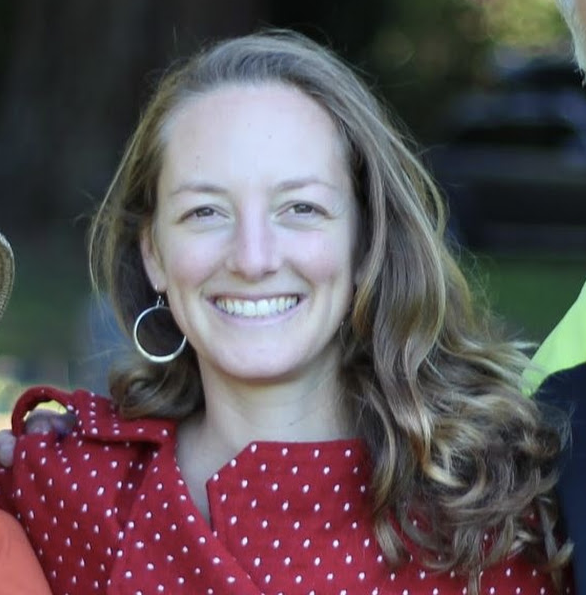
Michal Lahav
Michal Lahav is a Staff User Experience Researcher for AIUX in Google Research. Her research areas include human centered approaches to generative AI, AI memory, collaborative AI and assistive speech technologies. Her research supports incorporating global perspectives, community-based research practices, and helping AI be more equitable for underrepresented communities.
Authored Publications
Sort By
Google
A Systematic Review and Thematic Analysis of Community-Collaborative Approaches to Computing Research
Ned Cooper
Tiffanie Horne
Gillian Hayes
Jess Scon Holbrook
Lauren Wilcox
ACM Conference on Human Factors in Computing Systems (ACM CHI) 2022 (2022)
LaMPost: Evaluation of an AI-assisted Writing Email Editor Prototype for Adults with Dyslexia
Steven Goodman
Erin Buehler
Patrick Clary
Andy Coenen
Aaron Michael Donsbach
Tiffanie Horne
Bob MacDonald
Rain Breaw Michaels
Ajit Narayanan
Joel Christopher Riley
Alex Santana
Rachel Sweeney
Phil Weaver
Ann Yuan
Proceedings of ASSETS 2022, ACM (2022) (to appear)
"I don't think these devices are very culturally sensitive." - The impact of errors on African Americans in Automated Speech Recognition
Zion Mengesha
Juliana Sublewski
Elyse Tuennerman
Frontiers in Artificial Intelligence, 26 (2021)
Three Directions for the Design of Human-Centered Machine Translation
Samantha Robertson
Wesley Deng
Timnit Gebru
Margaret Mitchell
Samy Bengio
Niloufar Salehi
(2021)
Unmet Needs and Opportunities for Mobile Translation AI
Abigail Evans
Aaron Michael Donsbach
Boris Smus
Jess Scon Holbrook
Proceedings of the 2020 CHI Conference on Human Factors in Computing Systems (CHI ’20), ACM, Honolulu, Hawaii, USA