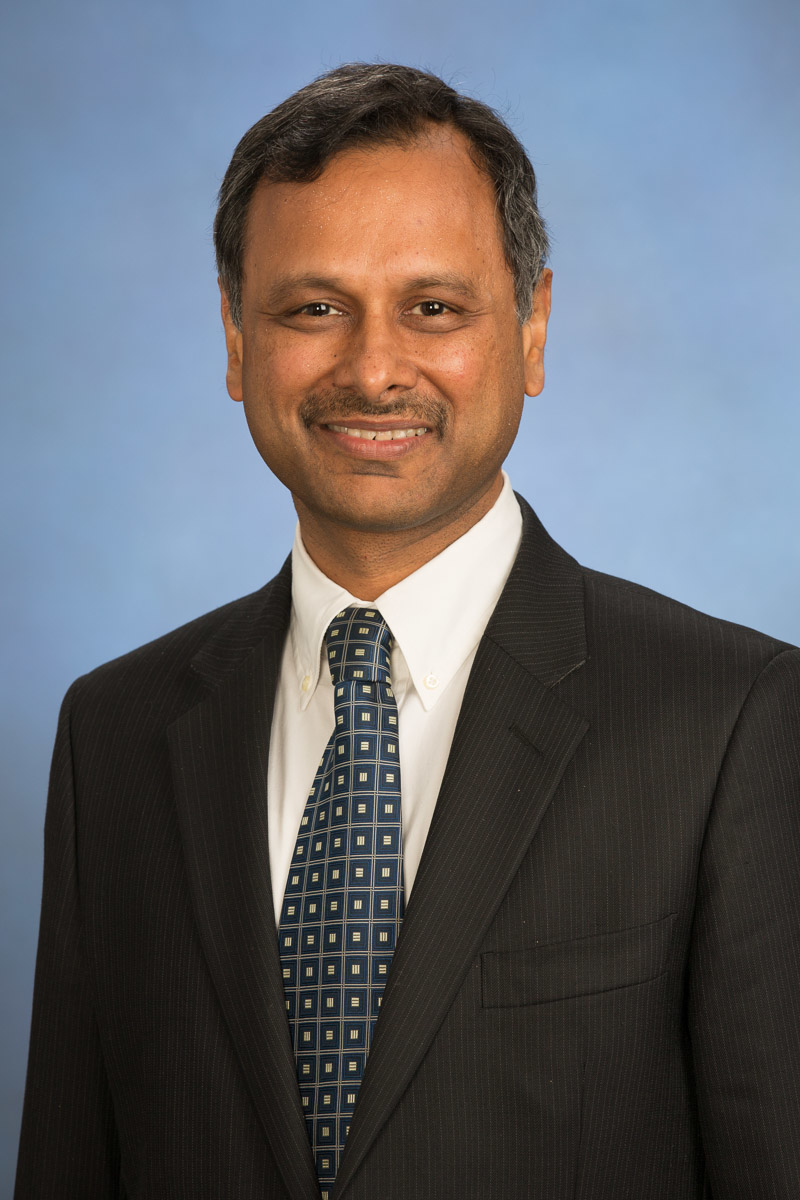
Manish Gupta
Dr. Manish Gupta is the Director of Google Research India. He holds an additional appointment as Infosys Foundation Chair Professor at IIIT Bangalore. Previously, Manish has led VideoKen, a video technology startup, and the research centers for Xerox and IBM in India. As a Senior Manager at the IBM T.J. Watson Research Center in Yorktown Heights, New York, Manish led the team developing system software for the Blue Gene/L supercomputer. IBM was awarded a National Medal of Technology and Innovation for Blue Gene by US President Barack Obama in 2009.
Manish holds a Ph.D. in Computer Science from the University of Illinois at Urbana Champaign. He has co-authored about 75 papers, with more than 7,000 citations in Google Scholar (and an h-index of 46), and has been granted 19 US patents. While at IBM, Manish received two Outstanding Technical Achievement Awards, an Outstanding Innovation Award and the Lou Gerstner Team Award for Client Excellence. Manish is a Fellow of ACM and the Indian National Academy of Engineering, and a recipient of a Distinguished Alumnus Award from IIT Delhi.
Authored Publications
Sort By
Preview abstract
Deep networks often make confident, yet, incorrect, predictions when tested with outlier data that is far removed from their training distributions. Likelihoods computed by deep generative models (DGMs) are a candidate metric for outlier detection with unlabeled data. Yet, previous studies have shown that DGM likelihoods are unreliable and can be easily biased by simple transformations to input data. Here, we examine outlier detection with variational autoencoders (VAEs), among the simplest of DGMs. We propose novel analytical and algorithmic approaches to ameliorate key biases with VAE likelihoods. Our bias corrections are sample-specific, computationally inexpensive, and readily computed for various decoder visible distributions. Next, we show that a well-known image pre-processing technique – contrast stretching – extends the effectiveness of bias correction to further improve outlier detection. Our approach achieves state-of-the-art accuracies with nine grayscale and natural image datasets, and demonstrates significant advantages – both with speed and performance – over four recent, competing approaches. In summary, lightweight remedies suffice to achieve robust outlier detection with VAEs.
View details
Walking with PACE — Personalized and Automated Coaching Engine
Nur Muhammad
Sriram Lakshminarasimhan
Deepak Nathani
Rahul Singh
Madhurima Vardhan
Martin Gamunu Seneviratne
Karina Lorenzana Livingston
Eshan Motwani
Srujana Merugu
Shantanu Prabhat
UMAP: 30th ACM Conference on User Modeling, Adaptation and Personalization (2022)
Preview abstract
Fitness coaching is effective in helping individuals to develop and maintain healthy lifestyle habits. However, there is a significant shortage of fitness coaches, particularly in low resource communities. Automated coaching assistants may help to improve the accessibility of personalized fitness coaching. Although a variety of automated nudge systems have been developed, few make use of formal behavior science principles and they are limited in their level of personalization. In this work, we introduce a computational framework leveraging the Fogg’s behavioral science model which serves as a personalised and automated coaching engine (PACE).PACE is a rule-based system that infers user state and suggests appropriate text nudges. We compared the effectiveness of PACE to human coaches in a Wizard-of-Oz deployment study with 33 participants over 21 days. Participants were randomized to either a human coach (’human’ arm, n=18) or the PACE framework handled by a human coach (’wizard’ arm, n=15). Coaches and participants interacted via a chat interface. We tracked coach-participant conversations, step counts and qualitative survey feedback. Our findings indicate that the PACE framework strongly emulated human coaching with no significant differences in the overall number of active days (PACE: 85.33% vs human: 92%, [p=NS]) and step count (PACE: 6674 vs human: 6605, [p=NS]) of participants from both groups.The qualitative user feedback suggests that PACE cultivated a coach-like experience, offering barrier resolution, motivation and educational support. As a post-hoc analysis, we annotated the conversation logs from the human coaching arm based on the Fogg framework, and then trained machine learning (ML) models on these data sets to predict the next coach action (AUC 0.73±0.02). This suggests that a ML-driven approach may be a viable alternative to a rule-based system in suggesting personalized nudges. In future, such an ML system could be made increasingly personalized and adaptive based on user behaviors.
View details