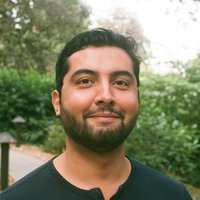
Leonardo Zepeda-Núñez
I'm a research scientist at Google Research working on Scientific Machine Learning with a focus on time-dependent physical systems with downstream applications to weather and climate. Before joining Google I was an assistant professor of mathematics at the University of Wisconsin-Madison. I hold a Ph.D. in Mathematics from MIT and I'm an alumni École Polytechnique. For more details see my homepage.
Research Areas
Authored Publications
Sort By
Google
DySLIM: Dynamics Stable Learning by Invariant Measure for Chaotic Systems
Yair Schiff
Jeff Parker
Volodymyr Kuleshov
International Conference on Machine Learning (ICML) (2024)
Neural Ideal Large Eddy Simulation: Modeling Turbulence with Neural Stochastic Differential Equations
Anudhyan Boral
James Lottes
Yi-fan Chen
John Anderson
Advances in Neural Information Processing Systems (NeurIPS) 36 (2023)
Debias Coarsely, Sample Conditionally: Statistical Downscaling through Optimal Transport and Probabilistic Diffusion Models
Ricardo Baptista
Yi-fan Chen
John Anderson
Anudhyan Boral
Advances in Neural Information Processing Systems (NeurIPS) 36 (2023)
Evolve Smoothly, Fit Consistently: Learning Smooth Latent Dynamics For Advection-Dominated Systems
Anudhyan Boral
International Conference on Learning Representations (ICLR) (2023)