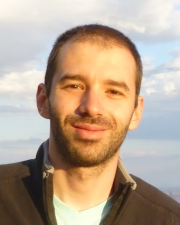
Kuzman Ganchev
I was born in Sofia, Bulgaria where I lived until February 1989. My family moved to Zimbabwe and then in 1995 to New Zealand where I went to high school. I came to the US in 1999 to study at Swarthmore College. I spent the 2001-2002 academic year studying abroad in Paris. After graduating with a Bachelor of Arts in Computer Science in 2003 I worked at StreamSage Inc. in Washington DC until starting at the University of Pennsylvania in Fall 2004. During the summer of 2007 I was an intern at TrialPay in Mountain View, CA and during the summer of 2008 I was an intern at Bank of America in New York. I graduated from UPenn in 2010 and have since been working at Google Inc. in New York.
Research Areas
Authored Publications
Sort By
Text-Blueprint: An Interactive Platform for Plan-based Conditional Generation
Fantine Huot
Reinald Kim Amplayo
Mirella Lapata
Proceedings of the 17th Conference of the European Chapter of the Association for Computational Linguistics: System Demonstrations (2023)
Preview abstract
While conditional generation models can now generate natural language well enough to create fluent text, it is still difficult to control the generation process, leading to irrelevant, repetitive, and hallucinated content. Recent work shows that planning can be a useful intermediate step to render conditional generation less opaque and more grounded. We present a web browser-based demonstration for query-focused summarization that uses a sequence of question-answer pairs, as a blueprint plan for guiding text generation (i.e., what to say and in what order). We illustrate how users may interact with the generated text and associated plan visualizations, e.g., by editing and modifying the blueprint in order to improve or control the generated output.
View details
Conditional Generation with a Question-Answering Blueprint
Reinald Kim Amplayo
Fantine Huot
Mirella Lapata
Transactions of the Association for Computational Linguistics (2023) (to appear)
Preview abstract
The ability to convey relevant and faithful information is critical for many tasks in conditional generation and yet remains elusive for neural seq-to-seq models whose outputs often reveal hallucinations and fail to correctly cover important details. In this work, we advocate planning as a useful intermediate representation for rendering conditional generation less opaque and more grounded. Our work proposes a new conceptualization of text plans as a sequence of question-answer (QA) pairs. We enhance existing datasets (e.g., for summarization) with a QA blueprint operating as a proxy for both content selection (i.e., what to say) and planning (i.e., in what order). We obtain blueprints automatically by exploiting state-of-the-art question generation technology and convert input-output pairs into input-blueprint-output tuples. We develop Transformer-based models, each varying in how they incorporate the blueprint in the generated output (e.g., as a global plan or iteratively). Evaluation across metrics and datasets demonstrates that blueprint models are more factual than alternatives which do not resort to planning and allow tighter control of the generation output.
View details
QAmeleon: Multilingual QA with Only 5 Examples
Fantine Huot
Sebastian Ruder
Mirella Lapata
Arxiv (2022)
Preview abstract
The availability of large, high-quality datasets has been one of the main drivers of recent progress in question answering (QA). Such annotated datasets however are difficult and costly to collect, and rarely exist in languages other than English, rendering QA technology inaccessible to underrepresented languages. An alternative to building large monolingual training datasets is to leverage pre-trained language models (PLMs) under a few-shot learning setting. Our approach, QAmeleon, uses a PLM to automatically generate multilingual data upon which QA models are trained, thus avoiding costly annotation. Prompt tuning the PLM for data synthesis with only five examples per language delivers accuracy superior to translation-based baselines, bridges nearly 60% of the gap between an English-only baseline and a fully supervised upper bound trained on almost 50,000 hand labeled examples, and always leads to substantial improvements compared to fine-tuning a QA model directly on labeled examples in low resource settings. Experiments on the TyDiQA-GoldP and MLQA benchmarks show that few-shot prompt tuning for data synthesis scales across languages and is a viable alternative to large-scale annotation.
View details
Attributed Question Answering: Evaluation and Modeling for Attributed Large Language Models
Pat Verga
Jianmo Ni
arXiv (2022)
Preview abstract
Large language models (LLMs) have shown impressive results across a variety of tasks while requiring little or no direct supervision. Further, there is mounting evidence that LLMs may have potential in information-seeking scenarios. We believe the ability of an LLM to attribute the text that it generates is likely to be crucial for both system developers and users in this setting. We propose and study Attributed QA as a key first step in the development of attributed LLMs. We develop a reproducable evaluation framework for the task, using human annotations as a gold standard and a correlated automatic metric that we show is suitable for development settings. We describe and benchmark a broad set of architectures for the task. Our contributions give some concrete answers to two key questions (How to measure attribution?, and How well do current state-of-the-art methods perform on attribution?), and give some hints as to how to address a third key question (How to build LLMs with attribution?).
View details
Preview abstract
The paper presents an approach to semantic grounding of language models (LMs) that conceptualizes the LM as a conditional model generating text given a desired semantic message. It embeds the LM in an auto-encoder by feeding its output to a semantic parser whose output is in the same representation domain as the input message.
Compared to a baseline that generates text using greedy search, we demonstrate two techniques that improve the fluency and semantic accuracy of the generated text: The first technique samples multiple candidate text sequences from which the semantic parser chooses. The second trains the language model while keeping the semantic parser frozen to improve the semantic accuracy of the auto-encoder.
We carry out experiments on the English WebNLG 3.0 data set, using BLEU to measure the fluency of generated text and standard parsing metrics to measure semantic accuracy. We show that our proposed approaches significantly improve on the greedy search baseline. Human evaluation corroborates the results of the automatic evaluation experiments.
View details
Preview abstract
A wide variety of neural-network architectures have been proposed for the task of Chinese word segmentation. Surprisingly, we find that a bidirectional LSTM model, when combined with standard deep learning techniques and best practices, can achieve better accuracy on many of the popular datasets as compared to models based on more complex neuralnetwork architectures. Furthermore, our error analysis shows that out-of-vocabulary words remain challenging for neural-network models, and many of the remaining errors are unlikely to be fixed through architecture changes. Instead, more effort should be made on exploring resources for further improvement.
View details
Globally Normalized Transition-Based Neural Networks
Association for Computational Linguistics (2016)
Preview abstract
We introduce a globally normalized transition-based neural network
model that achieves state-of-the-art part-of-speech tagging,
dependency parsing and sentence compression results. Our model is a
simple feed-forward neural network that operates on a task-specific
transition system, yet achieves comparable or better accuracies than
recurrent models.
We discuss the importance of global as opposed to local normalization:
a key insight is that the label bias problem implies that
globally
normalized models can be strictly more expressive
than locally normalized models.
View details
Semantic Role Labeling with Neural Network Factors
Oscar Täckström
Proceedings of the 2015 Conference on Empirical Methods in Natural Language Processing (EMNLP '15), Association for Computational Linguistics
Preview abstract
We present a new method for semantic role labeling in which arguments and semantic roles are jointly embedded in a shared vector space for a given predicate. These embeddings belong to a neural network, whose output represents the potential functions of a graphical model designed for the SRL task. We consider both local and structured learning methods and obtain strong results on standard PropBank and FrameNet corpora with a straightforward product-of-experts model. We further show how the model can learn jointly from PropBank and FrameNet annotations to obtain additional improvements on the smaller FrameNet dataset.
View details
Efficient Inference and Structured Learning for Semantic Role Labeling
Oscar Täckström
Transactions of the Association for Computational Linguistics, 3 (2015), pp. 29-41
Preview abstract
We present a dynamic programming algorithm for efficient constrained inference in semantic role labeling. The algorithm tractably captures a majority of the structural constraints examined by prior work in this area, which has resorted to either approximate methods or off-the-shelf integer linear programming solvers. In addition, it allows training a globally-normalized log-linear model with respect to constrained conditional likelihood. We show that the dynamic program is several times faster than an off-the-shelf integer linear programming solver, while reaching the same solution. Furthermore, we show that our structured model results in significant improvements over its local counterpart, achieving state-of-the-art results on both PropBank- and FrameNet-annotated corpora.
View details
Semantic Frame Identification with Distributed Word Representations
Karl Moritz Hermann
Jason Weston
Proceedings of the 52th Annual Meeting of the Association for Computational Linguistics (2014)
Preview abstract
We present a novel technique for semantic frame
identification using distributed representations of
predicates and their syntactic context; this technique leverages automatic syntactic parses and a generic set of word embeddings. Given labeled data annotated with frame-semantic parses, we learn a model that projects the set of word representations for the syntactic context around a predicate to a low dimensional representation. The latter is used for semantic frame identification; with a standard argument identification method inspired by prior work,
we achieve state-of-the-art results on FrameNet-style frame-semantic analysis. Additionally, we report strong results on PropBank-style semantic role labeling in comparison to prior work.
View details