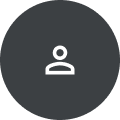
Krzysztof Rzadca
I am currently a visiting researcher at Google where I work on scheduling and resource management in Google Cloud. I am on leave from my faculty job - I'm an associate professor in the Institute of Informatics of the Faculty of Mathematics, Informatics and Mechanics, University of Warsaw, Poland, where I did my habilitation (HDR) in 2015 (my academic website)
Before joining UW, I was working as a research fellow (post-doc) in Anwitaman Datta's SANDS working group in the School of Computer Engineering (SCE) of the Nanyang Technological University (NTU), Singapore. I did my PhD on resource management in grids jointly in Laboratoire d'Informatique de Grenoble of Institut national polytechnique de Grenoble, France and Polish-Japanese Institute of Information Technology, Warsaw, Poland, as a French government fellow (co-tutelle grant). I graduated with a MSc from the Faculty of Electronics and Information Technology, Warsaw University of Technology, Poland.
I'm interested in resource management and scheduling in large-scale distributed systems, such as clouds, datacenters or supercomputers.
Research Areas
Authored Publications
Sort By
Google
Take it to the Limit: Peak Prediction-driven Resource Overcommitment in Datacenters
Noman Bashir
David Irwin
Sree Kodakara
Rohit Jnagal
Eurosys 2021 (2021)
Autopilot: Workload Autoscaling at Google Scale
Paweł Findeisen
Jacek Świderski
Przemyslaw Broniek
Beata Strack
Piotr Witusowski
Proceedings of the Fifteenth European Conference on Computer Systems, Association for Computing Machinery (2020) (to appear)