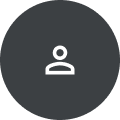
Karen DeSalvo
Dr. Karen DeSalvo is a physician executive working at the intersection of medicine, public health, and information technology to help everyone, everywhere, live a healthier life. She leads a team of experts at Google who build helpful products, develop AI solutions focused on some of the biggest health challenges and bring information and insights to consumers, caregivers and communities with the aim of democratizing access to health and healthcare. She provides clinical leadership for Google employee health, including as part of the company COVID response team. Prior to joining Google, Dr. DeSalvo was National Coordinator for Health Information Technology and Assistant Secretary for Health (Acting) in the Obama Administration. Dr. DeSalvo served as the New Orleans Health Commissioner following Hurricane Katrina and was previously Vice Dean for Community Affairs and Health Policy at the Tulane School of Medicine where she was a practicing internal medicine physician, educator, and researcher. She is co-founder of the National Alliance to Impact the Social Determinants of Health. Dr. DeSalvo serves on the Council of the National Academy of Medicine and the Board of Directors for Welltower.
Research Areas
Authored Publications
Sort By
Google
Race- and Ethnicity-Stratified Analysis of an Artificial Intelligence–Based Tool for Skin Condition Diagnosis by Primary Care Physicians and Nurse Practitioners
David Way
Vishakha Gupta
Yi Gao
Guilherme De Oliveira Marinho
Jay David Hartford
Kimberly Kanada
Kunal Nagpal
Lily Hao Yi Peng
Carter Dunn
Susan Jen Huang
Peggy Bui
(2022)
Development and Assessment of an Artificial Intelligence–Based Tool for Skin Condition Diagnosis by Primary Care Physicians and Nurse Practitioners in Teledermatology Practices
David Way
Vishakha Gupta
Yi Gao
Guilherme De Oliveira Marinho
Jay David Hartford
Kimberly Kanada
Kunal Nagpal
Lily Hao Yi Peng
Carter Dunn
Susan Jen Huang
Peggy Bui
JAMA Network Open (2021)