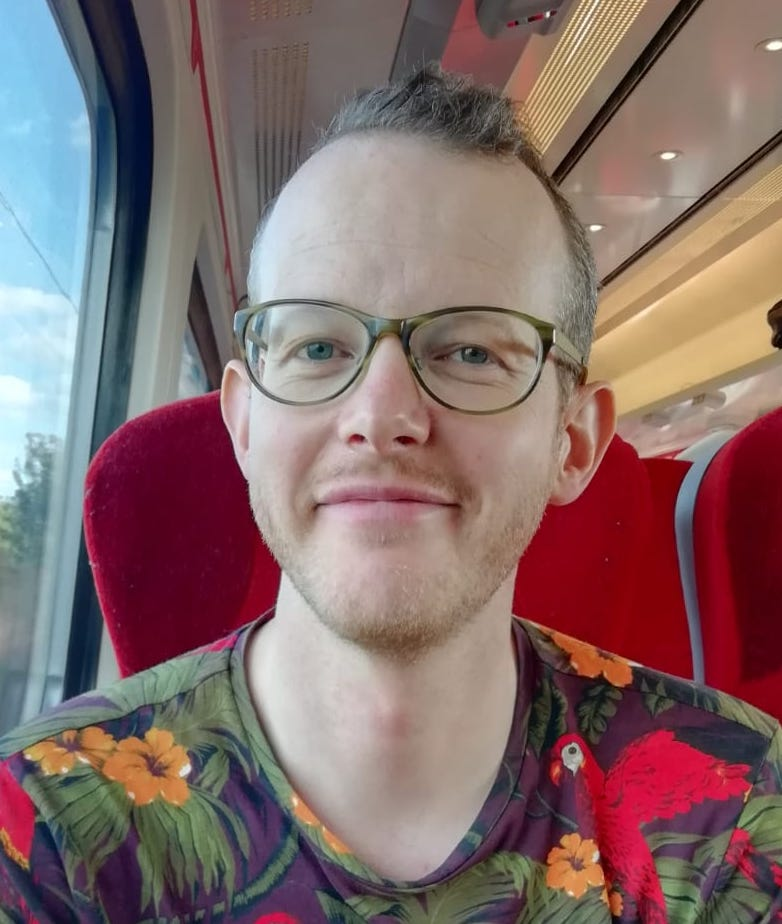
John Quinn
John Quinn is a researcher with the Google AI team in Ghana. His interests are in the application of machine intelligence methods to global challenges, e.g. in health, agriculture, and disaster response, and for addressing information gaps in the developing world. He is also a faculty member in the Department of Computer Science at Makerere University in Uganda, where he has worked since 2007. For five years prior to joining Google, he was the technical lead on a number of Africa analytics projects for United Nations Global Pulse, a UN data science initiative. He received a BA in Computer Science from the University of Cambridge in 2000, and PhD in machine learning from the University of Edinburgh in 2007.
Research Areas
Authored Publications
Sort By
Google
High Resolution Building and Road Segmentation from Sentinel-2 Imagery
Abdoulaye Diack
Abel Tesfaye Korme
Emmanuel Asiedu Brempong
Jason Hickey
Juliana Marcos
Krishna Sapkota
Mohammed Alewi Hassen
Wojciech Sirko
arXiv, https://arxiv.org/abs/2310.11622 (2023)
Continental-scale building detection from high resolution satellite imagery
Wojciech Sirko
Yasser Salah Eddine Bouchareb
Maxim Neumann
Moustapha Cisse
arXiv (2021)