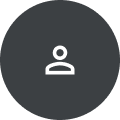
Hendrik Fichtenberger
Research Areas
Authored Publications
Sort By
Google
Constant Matters: Fine-grained Complexity of Differentially Private Continual Observation
Preview
Monika Henzinger
Jalaj Upadhyay
Proceedings of the 40th International Conference on Machine Learning (ICML) (2023)
Optimal Fully Dynamic k-Center Clustering for Adaptive and Oblivious Adversaries
Preview
Monika Henzinger
Andreas Wiese
Proceedings of the 2023 Annual ACM-SIAM Symposium on Discrete Algorithms (SODA)
HUGE: Huge Unsupervised Graph Embeddings with TPUs
SIGKDD Conference on Knowledge Discovery and Data Mining, ACM (2023) (to appear)