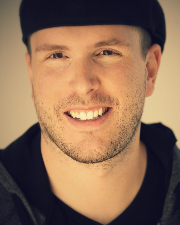
Elie Bursztein
I lead Google's anti-abuse research team, which invents ways to protect users against cyber-criminal activities and Internet threats. I've redesigned Google's CAPTCHA to make it easier, and I've made Chrome safer and faster by implementing better cryptography. I spend my spare time doing video game research, photography, and magic tricks. I was born in Paris, France, wear berets, and now live with my wife in Mountain View, California
Authored Publications
Sort By
Google
Generalized Power Attacks against Crypto Hardware using Long-Range Deep Learning
Karel Král
Marina Zhang
Transactions on Cryptographic Hardware and Embedded Systems (TCHES), IACR (2024)
Leveraging Virtual Reality to Enhance Diversity and Inclusion training at Google
Karla Brown
Patrick Gage Kelley
Leonie Sanderson
2024 CHI Conference on Human Factors in Computing Systems, ACM
Identifying and Mitigating the Security Risks of Generative AI
Clark Barrett
Brad Boyd
Nicholas Carlini
Brad Chen
Jihye Choi
Amrita Roy Chowdhury
Anupam Datta
Soheil Feizi
Kathleen Fisher
Tatsunori B. Hashimoto
Dan Hendrycks
Somesh Jha
Daniel Kang
Florian Kerschbaum
Eric Mitchell
John Mitchell
Zulfikar Ramzan
Khawaja Shams
Dawn Song
Ankur Taly
Diyi Yang
Foundations and Trends in Privacy and Security, 6 (2023), pp. 1-52
Hybrid Post-Quantum Signatures in Hardware Security Keys
Diana Ghinea
Jennifer Pullman
Julien Cretin
Rafael Misoczki
Stefan Kölbl
Applied Cryptography and Network Security Workshop (2023)
SoK: Hate, Harassment, and the Changing Landscape of Online Abuse
Devdatta Akhawe
Michael Bailey
Dan Boneh
Nicola Dell
Zakir Durumeric
Patrick Gage Kelley
Deepak Kumar
Damon McCoy
Sarah Meiklejohn
Thomas Ristenpart
Gianluca Stringhini
(2021)
Designing Toxic Content Classification for a Diversity of Perspectives
Deepak Kumar
Patrick Gage Kelley
Joshua Mason
Zakir Durumeric
Michael Bailey
(2021)
“Why wouldn’t someone think of democracy as a target?”: Security practices & challenges of people involved with U.S. political campaigns
Patrick Gage Kelley
Tara Matthews
Lee Carosi Dunn
Proceedings of the USENIX Security Symposium (2021)
Who is targeted by email-based phishing and malware? Measuring factors that differentiate risk
Camelia Simoiu
Proceedings of the Internet Measurement Conference (2020)
Spotlight: Malware Lead Generation at Scale
Bernhard Grill
Jennifer Pullman
Cecilia M. Procopiuc
David Tao
Borbala Benko
Proceedings of Annual Computer Security Applications Conference (ACSAC) (2020)