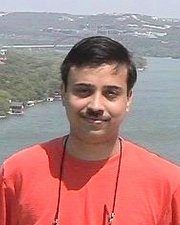
Sugato Basu
Authored Publications
Sort By
PRISM: A New Lens for Improved Color Understanding
Garima Pruthi
Inderjit Dhillon
Varun Jampani
EMNLP (2024)
Preview abstract
While image-text pre-trained models, such as CLIP, have demonstrated impressive capabilities in learning robust text and image representations, a critical area for substantial improvement remains—precise color understanding. In this paper, we address this limitation by introducing PRISM, a simple yet highly effective method that extends CLIP's capability to grasp the nuances of precise colors. PRISM seamlessly adapts to both recognized HTML colors and out-of-vocabulary RGB inputs through the utilization of our curated dataset of 100 image-text pairs, which can be effortlessly repurposed for fine-tuning with any desired color. Importantly, PRISM achieves these enhancements without compromising CLIP's performance on established benchmarks. During the fine-tuning process, PRISM encourages the disentanglement of color-relevant information from color-irrelevant details. Furthermore, we introduce a novel evaluation framework, ColorLens, featuring both seen and unseen test sets that can be readily repurposed to assess a model's precision in understanding precise colors. Our comprehensive evaluation and results demonstrate significant improvements over baseline models.
View details
Discriminative Diffusion Models as Few-shot Vision and Language Learners
Xuehai He
Weixi Feng
Tsu-Jui Fu
Varun Jampani
William Yang Wang
Xin Eric Wang
ArXiv (2023)
Preview abstract
Diffusion models, such as Stable Diffusion, have shown incredible performance on text-to-image generation. Since text-to-image generation often requires models to generate visual concepts with fine-grained details and attributes specified in text prompts, can we leverage the powerful representations learned by pre-trained diffusion models for discriminative tasks such as image-text matching? To answer this question, we propose a novel approach, Discriminative Stable Diffusion (DSD), which turns pre-trained text-to-image diffusion models into few-shot discriminative learners. Our approach uses the cross-attention score of a Stable Diffusion model to capture the mutual influence between visual and textual information and fine-tune the model via attention-based prompt learning to perform image-text matching. By comparing DSD with state-of-the-art methods on several benchmark datasets, we demonstrate the potential of using pre-trained diffusion models for discriminative tasks with superior results on few-shot image-text matching.
View details
MetaCLUE: Towards Comprehensive Visual Metaphors Research
Brendan Driscoll
Zhiwei Jia
Garima Pruthi
Leonidas Guibas
Varun Jampani
CVPR (2023)
Preview abstract
Creativity is an indispensable part of human cognition and also an inherent part of how we make sense of the world. Metaphorical abstraction is fundamental in communicating creative ideas through nuanced relationships between abstract concepts such as feelings. While computer vision benchmarks and approaches predominantly focus on understanding and generating literal interpretations of images, metaphorical comprehension of images remains relatively unexplored. Towards this goal, we introduce MetaCLUE, a set of vision tasks on visual metaphor. We also collect high-quality and rich metaphor annotations (abstract objects, concepts, relationships along with their corresponding object boxes) as there do not exist any datasets that facilitate the evaluation of these tasks. We perform a comprehensive analysis of state-of-the-art models in vision and language based on our annotations, highlighting strengths and weaknesses of current approaches in visual metaphor Classification, Localization, Understanding (retrieval, question answering, captioning) and gEneration (text-to-image synthesis) tasks. We hope this work provides a concrete step towards developing AI systems with human-like creative capabilities.
View details
LayoutGPT: Compositional Visual Planning and Generation with Large Language Models
Weixi Feng
Wangrong Zhu
Tsu-Jui Fu
Varun Jampani
Xuehai He
Xin Eric Wang
William Wang
NeurIPS (2023)
Preview abstract
Attaining a high degree of user controllability in visual generation often requires intricate, fine-grained inputs like layouts. However, such inputs impose a substantial burden on users when compared to simple text inputs. To address the issue, we study how Large Language Models (LLMs) can serve as visual planners by generating layouts from text conditions, and thus collaborate with visual generative models. We propose LayoutGPT, a method to compose in-context visual demonstrations in style sheet language to enhance the visual planning skills of LLMs. LayoutGPT can generate plausible layouts in multiple domains, ranging from 2D images to 3D indoor scenes. LayoutGPT also shows superior performance in converting challenging language concepts like numerical and spatial relations to layout arrangements for faithful text-to-image generation. When combined with a downstream image generation model, LayoutGPT outperforms text-to-image models/systems by 20-40% and achieves comparable performance as human users in designing visual layouts for numerical and spatial correctness. Lastly, LayoutGPT achieves comparable performance to supervised methods in 3D indoor scene synthesis, demonstrating its effectiveness and potential in multiple visual domains.
View details
KAFA: Rethinking Image Ad Understanding with Knowledge-Augmented Feature Adaptation of Vision-Language Models
Zhiwei Jia
Garima Pruthi
Hao Su
Varun Jampani
ACL 2023 (Industry Track) (2023)
Preview abstract
Image ad understanding is a crucial task with wide real-world applications. Although highly challenging with the involvement of diverse atypical scenes, real-world entities, and reasoning over scene-texts, how to interpret image ads is relatively under-explored, especially in the era of foundational vision-language models (VLMs) featuring impressive generalizability and adaptability. In this paper, we perform the first empirical study of image ad understanding through the lens of pre-trained VLMs. We benchmark and reveal practical challenges in adapting these VLMs to image ad understanding. We propose a simple feature adaptation strategy to effectively fuse multimodal information for image ads and further empower it with knowledge of real-world entities. We hope our study draws more attention to image ad understanding which is broadly relevant to the advertising industry.
View details
Training-Free Structured Diffusion Guidance for Compositional Text-to-Image Synthesis
Weixi Feng
Xuehai He
Tsu-Jui Fu
Varun Jampani
Xin Eric Wang
William Yang Wang
ICLR (2023)
Preview abstract
Large-scale diffusion models have achieved state-of-the-art results on text-to-image synthesis (T2I) tasks. Despite their ability to generate high-quality yet creative images, we observe that attribution-binding and compositional capabilities are still considered major challenging issues, especially when involving multiple objects. In this work, we improve the compositional skills of T2I models, specifically more accurate attribute binding and better image compositions. To do this, we incorporate linguistic structures with the diffusion guidance process based on the controllable properties of manipulating cross-attention layers in diffusion-based T2I models. We observe that keys and values in cross-attention layers have strong semantic meanings associated with object layouts and content. Therefore, we can better preserve the compositional semantics in the generated image by manipulating the cross-attention representations based on linguistic insights. Built upon Stable Diffusion, a SOTA T2I model, our structured cross-attention design is efficient that requires no additional training samples. We achieve better compositional skills in qualitative and quantitative results, leading to a 5-8% advantage in head-to-head user comparison studies. Lastly, we conduct an in-depth analysis to reveal potential causes of incorrect image compositions and justify the properties of cross-attention layers in the generation process.
View details
CPL: Counterfactual Prompt Learning for Vision and Language Models
Xuehai He
Diji Yang
Weixi Feng
Tsu-Jui Fu
Varun Jampani
William Yang Wang
Xin Eric Wang
Conference on Empirical Methods in Natural Language Processing (EMNLP) (2022)
Preview abstract
Prompt tuning is a new few-shot transfer learning technique that only tunes the learnable prompt for pre-trained vision and language models such as CLIP. However, existing prompt tuning methods tend to learn spurious or entangled representations, which leads to poor generalization to unseen concepts.
Towards non-spurious and efficient prompt learning from limited examples, this paper presents a novel Counterfactual Prompt Learning (CPL) method for vision and language models, which simultaneously employs counterfactual generation and contrastive learning in a joint optimization framework.
Particularly, CPL constructs counterfactual by identifying minimal non-spurious feature change between semantically-similar positive and negative samples that causes concept change, and learns more generalizable prompt representation from both factual and counterfactual examples via contrastive learning. Extensive experiments demonstrate that CPL can obtain superior few-shot performance on different vision and language tasks than previous prompt tuning methods on CLIP. On image classification, we achieve 3.55% average relative improvement on unseen classes across seven datasets; on image-text retrieval and visual question answering, we gain up to 4.09% and 25.08% relative improvements across three few-shot scenarios on unseen test sets respectively.
View details
Towards Understanding Sample Variance in Visually Grounded Language Generation: Evaluations and Observations
Wangrong Zhu
Xin Eric Wang
Kazoo Sone
William Wang
EMNLP 2020 (2020) (to appear)
Preview abstract
A major challenge in visually grounded language generation is to build robust benchmark datasets and models that can generalize well in real-world settings. To do this, it is critical to ensure that our evaluation protocols are correct, and benchmarks are reliable. In this work, we set forth to design a set of experiments to understand an important but often ignored problem in visually grounded language generation: given that humans have different utilities and visual attention, how will the sample variance in multi-reference datasets affect the models’ performance? Empirically, we study several multi-reference datasets and corresponding vision-and-language tasks. We show that it is of paramount importance to report variance in experiments; that human-generated references could vary drastically in different datasets/tasks, revealing the nature of each task; that metric-wise, CIDEr has shown systematically larger variances than others. Our evaluations on reference-per-instance shed light on the design of reliable datasets in the future.
View details
Product Phrase Extraction from e-Commerce Pages
Dmitrii Tochilkin
Kazoo Sone
The Proceedings of The Web Conference 2019, Companion
Preview abstract
Analyzing commercial pages to infer the products or services being offered by a web-based business is a task central to product search, product recommendation, ad placement and other e-commerce tasks. What makes this task challenging is that there are two types of e-commerce product pages. One is the single-product (SP) page where one product is featured primarily and users are able to buy that product or add to cart on the page. The other is the multi-product (MP) page, where users are presented with multiple (often 10-100) choices of products within a same category, often with thumbnail pictures and brief descriptions — users browse through the catalogue until they find a product they want to learn more about, and subsequently purchase the product of their choice on a corresponding SP page. In this paper, we take a two-step approach to identifying product phrases from commercial pages. First we
classify whether a commercial web page is a SP or MP page. To that end, we introduce two different image recognition based models to differentiate between these two types of pages. If the page is
determined to be SP, we identify the main product featured in that page. We compare the two types of image recognition models in terms of trade-offs between accuracy and latency, and empirically
demonstrate the efficacy of our overall approach.
View details
Micro-Browsing Models for Search Snippets
International Conference on Data Engineering (ICDE), IEEE (2019), pp. 1904-1909
Preview abstract
Click-through rate (CTR) is a key signal of relevance for search engine results, both organic and sponsored. CTR is the product of the probability of examination times the perceived relevance of the result. Hence there has been considerable work on user browsing models to separate out the examination and relevance components of CTR. However, the snippet text often plays a critical role in the perceived relevance of the result. In this paper, we propose a micro-browsing model for how users read result snippets. We validate the user model by considering the problem of predicting which of two different snippets will yield higher CTR. We show that classification accuracy is dramatically higher with our user model.
View details