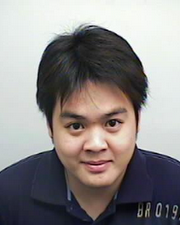
Eugene Ie
Authored Publications
Sort By
CoMSum: Dataset and Neural Model for Contextual Multi-Document Summarization
Sheide Chammas
Wan Zhu
International Conference on Document Analysis and Recognition, International Conference on Document Analysis and Recognition (2021)
Preview abstract
Summarization is the task of compressing source document(s) into coherent and succinct passages. Query-based (contextual) multi-document summarization (qMDS) is a variant that targets summaries to specific informational needs with queries providing additional contexts. Progress in qMDS has been hampered by limited availability of corresponding types of datasets. In this work, we make two contributions. First, we develop an automatic approach for creating both extractive and abstractive qMDS examples from existing language resources. We use this approach to create \qmds, a qMDS dataset for public use. Secondly, to validate the utility of \qmds, we propose a neural model for extractive summarization that exploits the hierarchical nature of the input from multiple documents. It also infuses queries into the modeling to extract query-specific summaries. The experimental results show that modeling the queries and the multiple documents hierarchically improve the performance of qMDS on this datasets. This is consitent with our intuition and supports using \qmds for developing learning methods for qMDS.
View details
RecSim NG: Toward Principled Uncertainty Modeling for Recommender Ecosystems
Martin Mladenov
Vihan Jain
Christopher Colby
Nicolas Mayoraz
Hubert Pham
Ivan Vendrov
ArXiv (2021)
Preview abstract
The development of recommender systems that optimize multi-turn interaction with users, and model the interactions of different
agents (e.g., users, content providers, vendors) in the recommender ecosystem have drawn increasing attention in recent years.
Developing and training models and algorithms for such recommenders can be especially difficult using static datasets, which often
fail to offer the types of counterfactual predictions needed to evaluate policies over extended horizons. To address this, we develop
RecSim NG, a probabilistic platform for the simulation of multi-agent recommender systems. RecSim NG is a scalable, modular,
differentiable simulator implemented in Edward2 and TensorFlow. It offers: a powerful, general probabilistic programming language for
agent-behavior specification; tools for probabilistic inference and latent-variable model learning, backed by automatic differentiation
and tracing; a TensorFlow-based runtime for running simulations on accelerated hardware. We describe RecSim NG and illustrate
how it can be used to create transparent, configurable, end-to-end models of a recommender ecosystem, complemented by a small
set of simple use cases that demonstrate how RecSim NG can help both researchers and practitioners easily develop and train novel algorithms for recommender systems.
A short version of this paper was published at RecSys 2020.
View details
On the Evaluation of Vision-and-Language Navigation Instructions
Ming Zhao
Peter Anderson
Vihan Jain
Su Wang
Conference of the European Chapter of the Association for Computational Linguistics (EACL) (2021)
Preview abstract
We introduce Room-Across-Room (RxR), a new Vision-and-Language Navigation (VLN) dataset. RxR is multilingual (English, Hindi, and Telugu) and larger (more paths and instructions) than other VLN datasets. It emphasizes the role of language in VLN by addressing known biases in paths and eliciting more references to visible entities. Furthermore, each word in an instruction is time-aligned to the virtual poses of instruction creators and validators. We establish baseline scores for monolingual and multilingual settings and multitask learning when including Room-to-Room annotations. We also provide results for a model that learns from synchronized pose traces by focusing only on portions of the panorama attended to in human demonstrations. The size, scope and detail of RxR dramatically expands the frontier for research on embodied language agents in simulated, photo-realistic environments.
View details
Preview abstract
Learning to follow instructions is of fundamental importance to autonomous agents for vision-and-language navigation (VLN). In this paper, we study how an agent can navigate long paths when learning from a corpus that consists of shorter ones. We show that existing state-of-the-art agents do not generalize well. To this end, we propose BabyWalk, a new VLN agent that is learned to navigate by decomposing long instructions into shorter ones (BabySteps) and completing them sequentially. A special design memory buffer is used by the agent to turn its past experiences into contexts for future steps. The learning process is composed of two phases. In the first phase, the agent uses imitation learning from demonstration to accomplish BabySteps. In the second phase, the agent uses curriculum-based reinforcement learning to maximize rewards on navigation tasks with increasingly longer instructions. We create two new benchmark datasets (of long navigation tasks) and use them in conjunction with existing ones to examine BabyWalk’s generalization ability. Empirical results show that BabyWalk achieves state-of-the-art results on several metrics, in particular, is able to follow long instructions better.
View details
Demonstrating Principled Uncertainty Modeling for Recommender Ecosystems with RecSim NG
Martin Mladenov
Vihan Jain
Christopher Colby
Nicolas Mayoraz
Hubert Pham
Ivan Vendrov
RecSys '20: Fourteenth ACM Conference on Recommender Systems (2020), pp. 591-593
Preview abstract
We develop RecSim NG, a probabilistic platform that supports natural, concise specification and learning of models for multi-agent recommender systems simulation. RecSim NG is a scalable, modular,
differentiable simulator implemented in Edward2 and TensorFlow.
An extended version of this paper is available as arXiv:2103.08057.
View details
Preview abstract
The Touchdown dataset (Chen et al., 2019) provides instructions by human annotators for navigation through New York City streets and for resolving spatial descriptions at a given location. To enable the wider research community to work effectively with the Touchdown tasks, we are publicly releasing the 29k raw Street View panoramas needed for Touchdown. We follow the process used for the StreetLearn data release (Mirowski et al., 2019) to check panoramas for personally identifiable information and blur them as necessary. These have been added to the StreetLearn dataset and can be obtained via the same process as used previously for StreetLearn. We also provide a reference implementation for both of the Touchdown tasks: vision and language navigation (VLN) and spatial description resolution (SDR). We compare our model results to those given in Chen et al. (2019) and show that the panoramas we have added to StreetLearn fully support both Touchdown tasks and can be used effectively for further research and comparison.
View details
Preview abstract
Learning to fuse vision and language information and represent them is an important topic with many applications. Recent progresses have leveraged the ideas of pre-training (from language modeling) and attention layers in Transformers to learn representation from datasets with images aligned with linguistic expressions that describe the images. In this paper, we propose learning representations from a set of implied visually grounded expressions between image and text, automatically mined from those datasets. In particular, we use denotation graphs to represent how specific concepts (such as sentences describing images) can be linked to abstract and generic concepts (such as short phrases) that are also visually grounded. This type of generic-to-specific relations can be discovered using linguistic analysis tools. We propose methods to incorporate such relations into learning representation. We show that state-of-the-art multimodal learning methods such as ViLBERT can be further improved by leveraging automatically harvested structural relations. The representations lead to stronger empirical results on downstream tasks of text-based image retrieval, and referral expression localization. We will release to the public both our codes and the extracted denotation graphs on both the Flickr30K and the COCO datasets.
View details
Transferable Representation Learning in Vision-and-Language Navigation
Haoshuo Huang
Vihan Jain
Harsh Mehta
Gabriel Ilharco Magalhaes
ICCV 2019 (2019)
Learning Dense Representations for Entity Retrieval
Larry Lansing
Diego Garcia-Olano
CoNLL (2019)
Preview abstract
We show that it is feasible to perform entity linking by training a dual encoder (two-tower) model that encodes mentions and entities in the same dense vector space, where candidate entities are retrieved by approximate nearest neighbor search. Unlike prior work, this setup does not rely on an alias table followed by a re-ranker, and is thus the first fully learned entity retrieval model. We show that our dual encoder, trained using only anchor-text links in Wikipedia, outperforms discrete alias table and BM25 baselines, and is competitive with the best comparable results on the standard TACKBP-2010 dataset. In addition, it can retrieve candidates extremely fast, and generalizes well to a new dataset derived from Wikinews. On the modeling side, we demonstrate the dramatic value of an unsupervised negative mining algorithm for this task.
View details