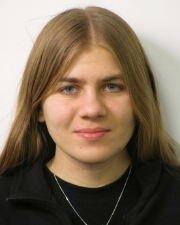
Angelika Moscicki
Authored Publications
Sort By
Google
Evaluating Login Challenges as a Defense Against Account Takeover
Periwinkle Doerfler
Maija Marincenko
Juri Ranieri
Yu Jiang
Damon McCoy
(2019)
Data breaches, phishing, or malware? Understanding the risks of stolen credentials
Frank Li
Juri Ranieri
Yarik Markov
Oxana Comanescu
Vijay Eranti
Daniel Margolis
Vern Paxson
(2017)
Easy Does It: More Usable CAPTCHAs
Celine Fabry
Steven Bethard
John C. Mitchell
Dan Jurafasky
CHI '14 Proceedings of the SIGCHI Conference on Human Factors in Computing Systems, ACM, 1600 Amphitheatre Pkwy (2014), pp. 2637-2646
The End is Nigh: Generic Solving of Text-based CAPTCHAs
Jonathan Aigrain
John C. Mitchell
WOOT'14 Proceedings of the 8th USENIX conference on Offensive Technologies, Usenix (2014)