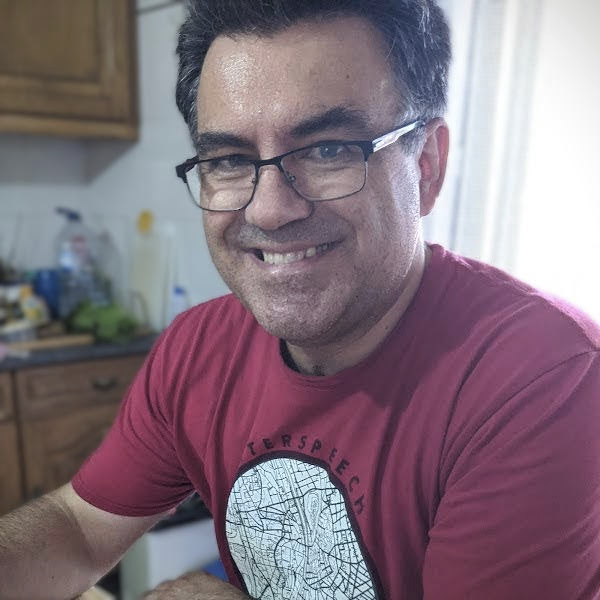
Diamantino Caseiro
Diamantino Caseiro is a research scientist at Google from May 2014. Prior to joining Google, he was a researcher at AT&T Labs Research. Before that he held the position of assistant professor of computer science at Instituto Superior Tecnico, Technical University of Lisbon, and was a researcher at INESC-ID Lisbon. His main areas of interest are Automatic Speech Recognition, Speech Processing, Natural Language Processing, and Artificial Intelligence.
Authored Publications
Sort By
An Efficient Streaming Non-Recurrent On-Device End-to-End Model with Improvements to Rare-Word Modeling
Rami Botros
Ruoming Pang
David Johannes Rybach
James Qin
Quoc-Nam Le-The
Anmol Gulati
Cal Peyser
Chung-Cheng Chiu
Emmanuel Guzman
Jiahui Yu
Qiao Liang
Wei Li
Yu Zhang
Interspeech (2021) (to appear)
Preview abstract
On-device end-to-end (E2E) models have shown improvementsover a conventional model on Search test sets in both quality, as measured by Word Error Rate (WER), and latency, measured by the time the result is finalized after the user stops speaking. However, the E2E model is trained on a small fraction of audio-text pairs compared to the 100 billion text utterances that a conventional language model (LM) is trained with. Thus E2E models perform poorly on rare words and phrases. In this paper, building upon the two-pass streaming Cascaded Encoder E2E model, we explore using a Hybrid Autoregressive Transducer (HAT) factorization to better integrate an on-device neural LM trained on text-only data. Furthermore, to further improve decoder latency we introduce a non-recurrent embedding decoder, in place of the typical LSTM decoder, into the Cascaded Encoder model. Overall, we present a streaming on-device model that incorporates an external neural LM and outperforms the conventional model in both search and rare-word quality, as well as latency, and is 318X smaller.
View details
Improving Automatic Speech Recognition with Neural Embeddings
Christopher Li
2021 IEEE International Conference on Acoustics, Speech, and Signal Processing, 111 8th Ave
New York, NY 10011 (2021)
Preview abstract
A common challenge in automatic speech recognition (ASR) systems is successfully decoding utterances containing long tail entities. Examples of entities include unique contact names and local restaurant names that may be out of vocabulary, and therefore absent from the training set. As a result, during decoding, such entities are assigned low likelihoods by the model and are unlikely to be recognized. In this paper, we apply retrieval in an embedding space to recover such entities. In the aforementioned embedding space, embedding representations of phonetically similar entities are designed to be close to one another in cosine distance. We describe the neural networks and the infrastructure to produce such embeddings. We also demonstrate that using neural embeddings improves ASR quality by achieving an over 50% reduction in word error rate (WER) on evaluation sets for popular media queries.
View details
Preview abstract
End-to-end (E2E) mixed case automatic speech recognition systems
(ASR) that directly predict words in the written domain are attractive
due to being simple to build, not requiring explicit capitalization
models, allowing streaming capitalization without additional effort
beyond that required for streaming ASR, and their small size.
However, the fact that these systems produce various versions of the same
word with different capitalizations, and even different word
segmentations for different case variants when wordpieces (WP) are predicted,
leads to multiple problems with contextual ASR. In particular,
the size and time to build contextual models grows considerably
with the number of variants per word. In this paper, we propose
separating orthographic recognition from capitalization, so that the
ASR system first predicts a word, then predicts its capitalization in
the form of a capitalization mask. We show that the use of capitalization
masks achieves the same low error rate as traditional mixed
case ASR, while reducing the size and compilation time of contextual models.
Furthermore, we observe significant improvements in capitalization quality.
View details
Entropy Based Pruning of Backoff MaxEnt Language Models with Contextual Features
Tongzhou Chen
Proceedings of the IEEE International Conference on Acoustics, Speech and Signal Proceesing, Calgary, Canada (2018)
Preview abstract
In this paper, we present a pruning technique for maximum entropy (MaxEnt)
language models. It is based on computing the exact entropy loss when removing
each feature from the model, and it explicitly supports backoff features by
replacing each removed feature with its backoff.
The algorithm computes the loss on the training data, so it is not restricted to
models with n-gram like features, allowing models with any feature, including
long range skips, triggers, and contextual features such as device location.
Results on the 1-billion word corpus show large perplexity improvements
relative for frequency pruned models of comparable size.
Automatic speech recognition (ASR) experiments show up to 0.2\% absolute WER
improvements in a large-scale cloud based mobile ASR system for Italian.
View details
Preview abstract
Maximum Entropy (MaxEnt) Language Models (LMs) are powerful models
that can incorporate linguistic and non-linguistic contextual signals
in a unified framework, by optimizing a convex loss function.
In addition to their flexibility, a key advantage is their scalability,
in terms of model size and the amount of data that can be used during
training. We present the following two contributions to
MaxEnt training: (1) By leveraging smaller amounts of transcribed
data, we demonstrate that a MaxEnt LM trained on various
types of corpora can be easily adapted to better match the test
distribution of speech recognition; (2) A novel adaptive-training approach that efficiently
models multiple types of non-linguistic features in a
universal model.
We test the impact of these approaches on Google's state-of-the-art
speech recognizer for the task of voice-search transcription and
dictation. Training 10B parameter models utilizing a corpus
of up to 1T words, we show large reductions in word error
rate from adaptation across multiple languages. Also, human evaluations
show strong significant improvements on a wide range of domains from
using non-linguistic signals. For example, adapting to geographical
domains (e.g., US States and cities) affects about 4% of test
utterances, with 2:1 wins to loss ratio.
View details
Sparse Non-negative Matrix Language Modeling: Maximum Entropy Flexibility on the Cheap
The 18th Annual Conference of the International Speech Communication Association, Stockholm, Sweden, pp. 2725-2729 (to appear)
Preview abstract
We present a new method for estimating the sparse non-negative model (SNM) by
using a small amount of held-out data and the multinomial loss that is natural
for language modeling; we validate it experimentally against the previous
estimation method which uses leave-one-out on training data and a binary loss
function and show that it performs equally well. Being able to train on
held-out data is very important in practical situations where training data is
mismatched from held-out/test data. We find that fairly small amounts of
held-out data (on the order of 30-70 thousand words) are sufficient for
training the adjustment model, which is the only model component estimated
using gradient descent; the bulk of model parameters are relative frequencies
counted on training data.
A second contribution is a comparison between SNM and the related class of
Maximum Entropy language models. While much cheaper computationally, we show
that SNM achieves slightly better perplexity results for the same feature set
and same speech recognition accuracy on voice search and short message
dictation.
View details