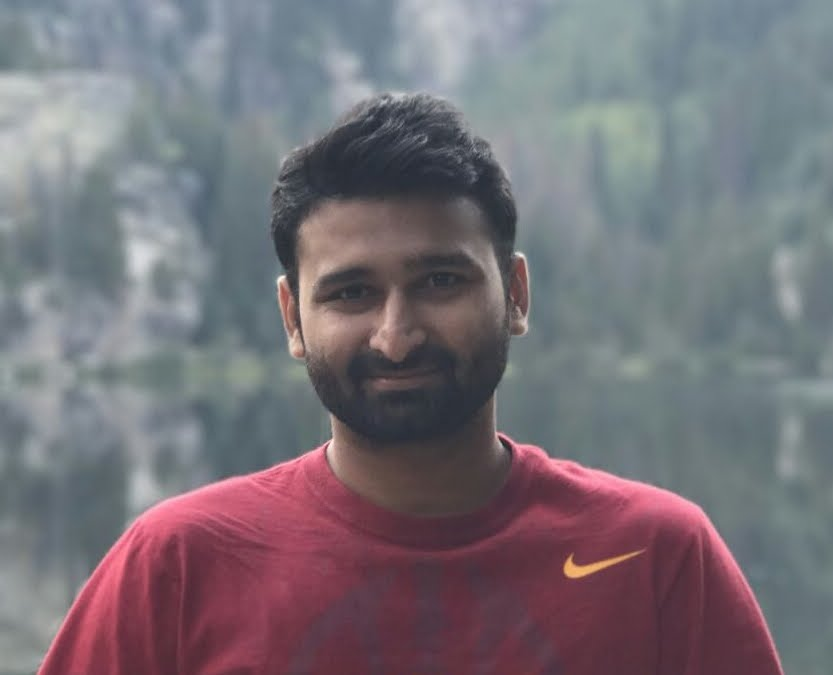
Roopal Garg
Roopal Garg is a Staff Software Engineer at Google DeepMind, where he works on improving multimodal content understanding. His focus is on building high-quality datasets efficiently and using them to develop autoraters and metrics to advance and evaluate modern Vision-Language Models (VLMs). Recently, he's been focused on hyper-detailed image descriptions, their implications for text-to-image models, and expanding these capabilities to include global geographical and cultural understanding. He received his MS in Computer Science with a focus on Natural Language Processing from the University of Southern California in 2013.
Research Areas
Authored Publications
Sort By
DOCCI: Descriptions of Connected and Contrasting Images
Garrett Tanzer
Jaemin Cho
Su Wang
Sunayana Rane
Zack Berger
Zarana Parekh
(2024)
Preview abstract
Despite recent advancements, text-to-image (T2I) models still exhibit critical limitations, such as errors in understanding spatial relationships, object counting, text rendering, and more. One challenge in overcoming these failure modes is the lack of resources; the majority of existing image-text datasets provide only brief captions that do not offer sufficient detail to discrepancies between images and their descriptions. To advance the development of T2I models further, we introduce \textbf{Descriptions of Connected and Contrasting Images (DOCCI)}, a dataset of 15k images taken by a single person with detailed human-annotated descriptions in English. We meticulously annotated detailed and coherent descriptions, averaging 136 words, which sufficiently differentiate images from related or similar ones. We intentionally curated images that showcase a diverse range of visual properties, including entities with their attributes, various orientations, and lighting effects, many of which are related to each other. We thoroughly analyze the quality and characteristics of the image-description pairs, and assess the performance of the latest T2I and I2T models. The experimental results indicate that the current state-of-the-art T2I models still struggle with the aforementioned challenges, and even the SOTA models have not fully addressed them. DOCCI is publicly available, and we believe that this dataset will be a valuable benchmark for vision-language research.
View details
ImageInWords: Unlocking Hyper-Detailed Image Descriptions
Andrew Bunner
Ranjay Krishna
(2024)
Preview abstract
Despite the longstanding adage "an image is worth a thousand words," creating accurate and hyper-detailed image descriptions for training Vision-Language models remains challenging.
Current datasets typically have web-scraped descriptions that are short, low-granularity, and often contain details unrelated to the visual content. As a result, models trained on such data generate descriptions replete with missing information, visual inconsistencies, and hallucinations. To address these issues, we introduce ImageInWords (IIW), a carefully designed human-in-the-loop annotation framework for curating hyper-detailed image descriptions and a new dataset resulting from this process.
We validate the framework through evaluations focused on the quality of the dataset and its utility for fine-tuning with considerations for readability, comprehensiveness, specificity, hallucinations, and human-likeness. Our dataset significantly improves across these dimensions compared to recently released datasets (+66%) and GPT-4V outputs (+48%). Furthermore, models fine-tuned with IIW data excel by +31% against prior work along the same human evaluation dimensions. Given our fine-tuned models, we also evaluate text-to-image generation and vision-language reasoning. Our model's descriptions can generate images closest to the original, as judged by both automated and human metrics. We also find our model produces more compositionally rich descriptions, outperforming the best baseline by up to 6% on ARO, SVO-Probes, and Winoground datasets.
View details
Mismatch Quest: Visual and Textual Feedback for Image-Text Misalignment
Brian Gordon
Dani Lischinski
Daniel Cohen-Or
arXiv (2023)
Preview abstract
While existing image/text alignment models reach high quality binary assessments, they fall short of pinpointing the exact source of misalignment.
In this paper, we present a method to provide detailed textual and visual explanation of detected misalignments between text/image pairs.
We leverage large language models to automatically construct a training set that holds plausible misaligned captions for a given image and corresponding textual explanations and visual indicators. We also introduce a new human curated test set comprising ground-truth textual and visual misalignment annotations. Empirical results show that fine-tuning vision language models on our training set enables them to articulate misalignments and visually indicate them within images, outperforming strong baselines both on the binary alignment classification and the explanation generation tasks.
View details