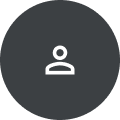
Christopher H Van Arsdale
Chris currently leads Google Research’s Climate & Energy group.
He studied Physics and Computer Science at the University of Washington, and has since led many large scale computing, remote sensing, and information retrieval projects inside Google. Chris has previously worked in venture capital before focusing on Climate Change research.
He studied Physics and Computer Science at the University of Washington, and has since led many large scale computing, remote sensing, and information retrieval projects inside Google. Chris has previously worked in venture capital before focusing on Climate Change research.
Authored Publications
Sort By
Satellite Sunroof: High-res Digital Surface Models and Roof Segmentation for Global Solar Mapping
Alex Wilson
Betty Peng
Carl Elkin
Umangi Jain
Preview abstract
The transition to renewable energy sources such as solar is crucial for mitigating climate change. Google Maps Platform Solar API aims to accelerate this transition by providing accurate estimates of solar potential for buildings covered by aerial imagery. However, its impact is limited by geographical coverage and data availability. This paper presents an approach to expand the project's capabilities using satellite imagery, enabling global-scale solar potential assessment. We address challenges specific to satellite imagery, such as lower resolution and oblique views, by developing deep learning models for Digital Surface Model (DSM) estimation and roof plane segmentation. The models are trained and evaluated on datasets comprising of spatially aligned satellite and aerial imagery. Our results demonstrate the effectiveness of our approach in accurately predicting DSMs and roof segments from satellite imagery, paving the way for a significant expansion of the Solar API and impact in promoting solar adoption.
View details
The effect of uncertainty in humidity and model parameters on the prediction of contrail energy forcing
Marc Shapiro
Zebediah Engberg
Marc E.J. Stettler
Roger Teoh
Ulrich Schumann
Susanne Rohs
Erica Brand
Environmental Research Communications, 6 (2024), pp. 095015
Preview abstract
Previous work has shown that while the net effect of aircraft condensation trails (contrails) on the climate is warming, the exact magnitude of the energy forcing per meter of contrail remains uncertain. In this paper, we explore the skill of a Lagrangian contrail model (CoCiP) in identifying flight segments with high contrail energy forcing. We find that skill is greater than climatological predictions alone, even accounting for uncertainty in weather fields and model parameters. We estimate the uncertainty due to humidity by using the ensemble ERA5 weather reanalysis from the European Centre for Medium-Range Weather Forecasts (ECMWF) as Monte Carlo inputs to CoCiP. We unbias and correct under-dispersion on the ERA5 humidity data by forcing a match to the distribution of in situ humidity measurements taken at cruising altitude. We take CoCiP energy forcing estimates calculated using one of the ensemble members as a proxy for ground truth, and report the skill of CoCiP in identifying segments with large positive proxy energy forcing. We further estimate the uncertainty due to model parameters in CoCiP by performing Monte Carlo simulations with CoCiP model parameters drawn from uncertainty distributions consistent with the literature. When CoCiP outputs are averaged over seasons to form climatological predictions, the skill in predicting the proxy is 44%, while the skill of per-flight CoCiP outputs is 84%. If these results carry over to the true (unknown) contrail EF, they indicate that per-flight energy forcing predictions can reduce the number of potential contrail avoidance route adjustments by 2x, hence reducing both the cost and fuel impact of contrail avoidance.
View details
Contrail Detection on GOES-16 ABI with the OpenContrails Dataset
Joe Ng
Jian Cui
Vincent Rudolf Meijer
Erica Brand
IEEE Transactions on Geoscience and Remote Sensing (2023)
Preview abstract
Contrails (condensation trails) are line-shaped ice clouds caused by aircraft and are a substantial contributor to aviation-induced climate change. Contrail avoidance is potentially an inexpensive way to significantly reduce the climate impact of aviation. An automated contrail detection system is an essential tool to develop and evaluate contrail avoidance systems. In this article, we present a human-labeled dataset named OpenContrails to train and evaluate contrail detection models based on GOES-16 Advanced Baseline Imager (ABI) data. We propose and evaluate a contrail detection model that incorporates temporal context for improved detection accuracy. The human labeled dataset and the contrail detection outputs are publicly available on Google Cloud Storage at gs://goes_contrails_dataset .
View details
Estimates of broadband upwelling irradiance from GOES-16 ABI
Sixing Chen
Vincent Rudolf Meijer
Joe Ng
Geoff Davis
Carl Elkin
Remote Sensing of Environment, 285 (2023)
Preview abstract
Satellite-derived estimates of the Earth’s radiation budget are crucial for understanding and predicting the weather and climate. However, existing satellite products measuring broadband outgoing longwave radiation (OLR) and reflected shortwave radiation (RSR) have spatio-temporal resolutions that are too coarse to evaluate important radiative forcers like aircraft condensation trails. We present a neural network which estimates OLR and RSR based on narrowband radiances, using collocated Cloud and Earth’s Radiant Energy System (CERES) and GOES-16 Advanced Baseline Imager (ABI) data. The resulting estimates feature strong agreement with the CERES data products (R^2 = 0.977 for OLR and 0.974 for RSR on CERES Level 2 footprints), and we provide open access to the collocated satellite data and model outputs on all available GOES-16 ABI data for the 4 years from 2018–2021.
View details
Preview abstract
The majority of IPCC scenarios call for active CO2 removal (CDR) to remain below 2ºC of warming. On geological timescales, ocean uptake regulates atmospheric CO2 concentration, with two homeostats driving sequestration: dissolution of deep ocean calcite deposits and terrestrial weathering of silicate rocks, acting on 1ka to 100ka timescales. Many current ocean-based CDR proposals effectively act to accelerate the latter. Here we present a method which relies purely on the redistribution and dilution of acidity from a thin layer of the surface ocean to a thicker layer of deep ocean, with the aim of accelerating the former carbonate homeostasis. This downward transport could be seen analogous to the action of the natural biological carbon pump. The method offers advantages over other ocean CDR methods and direct air capture approaches (DAC): the conveyance of mass is minimized (acidity is pumped in situ to depth), and expensive mining, grinding and distribution of alkaline material is eliminated. No dilute substance needs to be concentrated, avoiding the Sherwood’s Rule costs typically encountered in DAC. Finally, no terrestrial material is added to the ocean, avoiding significant alteration of seawater ion concentrations and issues with heavy metal toxicity encountered in mineral-based alkalinity schemes.
The artificial transport of acidity accelerates the natural deep ocean invasion and subsequent compensation by calcium carbonate. It is estimated that the total compensation capacity of the ocean is on the order of 1500GtC. We show through simulation that pumping of ocean acidity could remove up to 150GtC from the atmosphere by 2100 without excessive increase of local ocean pH. For an acidity release below 2000m, the relaxation half time of CO2 return to the atmosphere was found to be ~2500 years (~1000yr without accounting for carbonate dissolution), with ~85% retained for at least 300 years. The uptake efficiency and residence time were found to vary with the location of acidity pumping, and optimal areas were calculated.
Requiring only local resources (ocean water and energy), this method could be uniquely suited to utilize otherwise-stranded open ocean energy sources at scale. We examine technological pathways that could be used to implement it and present a brief techno-economic estimate of 130-250$/tCO2 at current prices and as low as 86$/tCO2 under modest learning-curve assumptions.
View details
A human-labeled Landsat contrails dataset
Vincent Rudolf Meijer
Erica Wickstrom Brand
Carl Elkin
ICML workshop on Climate Change 2021 (2021)
Preview abstract
Contrails (condensation trails) are the ice clouds that trail behind aircraft as they fly through cold and moist regions of the atmosphere. Avoiding these regions could potentially be an inexpensive way to reduce over half of aviation's impact on global warming. Development and evaluation of these avoidance strategies greatly benefits from the ability to detect contrails on satellite imagery. Since little to no public data is available to develop such contrail detectors, we construct and release a dataset of several thousand Landsat-8 scenes with pixel-level annotations of contrails. The dataset will continue to grow, but currently contains 3431 scenes (of which 47\% have at least one contrail) representing 800+ person-hours of labeling time.
View details