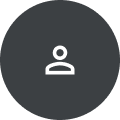
Omer Tov
Research Areas
Authored Publications
Sort By
BlazeStyleGAN: A Real-Time On-Device StyleGAN
Fei Deng
Lu Wang
Chuo-Ling Chang
Tingbo Hou
(2023)
Preview abstract
StyleGAN models have been widely adopted for generating and editing face images. Yet, few work investigated running StyleGAN models on mobile devices. In this work, we introduce BlazeStyleGAN --- to the best of our knowledge, the first StyleGAN model that can run in real-time on smartphones. We design an efficient synthesis network with the auxiliary head to convert features to RGB at each level of the generator, and only keep the last one at inference. We also improve the distillation strategy with a multi-scale perceptual loss using the auxiliary heads, and an adversarial loss for the student generator and discriminator. With these optimizations, BlazeStyleGAN can achieve real-time performance on high-end mobile GPUs. Experimental results demonstrate that BlazeStyleGAN generates high-quality face images and even mitigates some artifacts from the teacher model.
View details
Imagic: Non-Rigid Real Image Editing with Text-Conditioned Diffusion Models
Bahjat Kawar
Huiwen Chang
Michal Irani
Shiran Zada
arxiv (2023) (to appear)
Preview abstract
Text-conditioned image editing has recently attracted considerable interest. However, most methods are currently limited to simple edits (e.g., painting something on an object), are applied to synthetically generated images, or require multiple input images of a common object.
In this paper we demonstrate, for the very first time, the ability to apply complex non-rigid edits to a single real image -- i.e., change the pose of an object inside a real image, while preserving the remaining parts of the image. Our method can make a standing dog sit down or jump, cause a bird to spread its wings, etc. -- each within its single high-resolution natural image provided by the user.
Contrary to previous work, our proposed method requires only a single input image and a target text (the desired edit). It operates on real images, and does not require any additional inputs (such as image masks or additional views of the scene/object).
Our method, which we call Imagic, leverages a pre-trained text-to-image diffusion model for this task. It modifies the text embedding to satisfy both the input image and the target text, while fine-tuning the diffusion model to capture the image-specific appearance.
We demonstrate the quality and versatility of our method on numerous inputs from various domains, showcasing high quality complex image edits.
View details
Teaching CLIP to Count to Ten
Michal Irani
Roni Paiss
Shiran Zada
Submission to CVPR 2023 (2023)
Preview abstract
Large vision-language models, such as CLIP, learn robust representations of text and images, facilitating advances in many downstream tasks, including zero-shot classification and text-to-image generation. However, these models have several well-documented limitations. They fail to encapsulate compositional concepts, such as counting objects in an image or the relations between objects.
To the best of our knowledge, this work is the first to extend CLIP to handle object counting. We introduce a simple yet effective method to improve the quantitative understanding of vision-language models, while maintaining their overall performance on common benchmarks.
Our method automatically augments image captions to create hard negative samples that differ from the original captions by only the number of objects. For example, an image of three dogs can be contrasted with the negative caption "Six dogs playing in the yard". A dedicated loss encourages discrimination between the correct caption and its negative variant.
We introduce CountBench, a new benchmark for evaluating a model's understanding of object counting, and demonstrate significant improvement over baseline models on this task. Furthermore, we leverage our improved CLIP representations for image generation, and show that our model can produce specific counts of objects more reliably than existing ones.
View details
Self-Distilled StyleGAN: Towards Generation from Internet Photos
Ron Mokady
Michal Yarom
Michal Irani
Proceedings of the 49th Annual Conference on Computer Graphics and Interactive Techniques (2022)
Preview abstract
StyleGAN is known to produce high-fidelity images, while also offering unprecedented semantic editing. However, these fascinating abilities have been demonstrated only on a limited set of datasets, which are usually structurally aligned and well curated.
In this paper, we show how StyleGAN can be adapted to work on raw uncurated images collected from the Internet. Such image collections impose two main challenges to StyleGAN: they contain many outlier images, and are characterized by a multi-modal distribution. Training StyleGAN on such raw image collections results in degraded image synthesis quality. To meet these challenges, we proposed a StyleGAN-based self-distillation approach, which consists of two main components: (i) A generative-based self-filtering of the dataset to eliminate out-of-distribution images, in order to generate an adequate training set, and (ii) Perceptual clustering of the generated images to detect the inherent data modalities, which are then employed to improve StyleGAN’s “truncation trick” in the image synthesis process. The presented technique enables the generation of high-quality images, while better reserving the diversity of the data. Through qualitative and quantitative evaluation, we demonstrate the power of our approach to new challenging and diverse domains collected from the Internet. New datasets and pre-trained models will be published upon acceptance.
View details