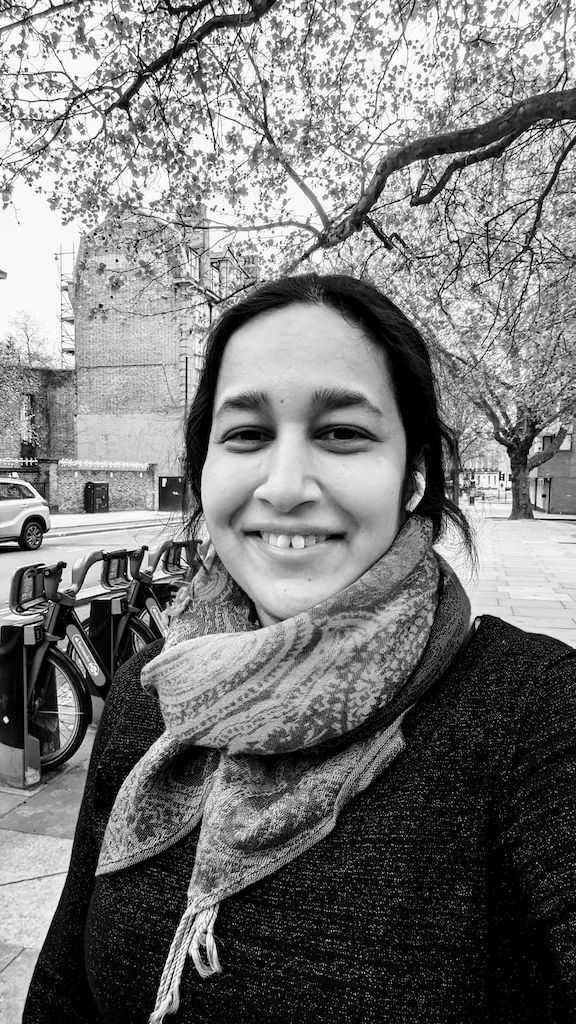
Preksha Nema
Preksha Nema is a Research Scientist in Advertising-Sciences team in Google Research, Bengaluru, India. Her work revolves around understanding and generating purposeful Ads, with a focus on multilingual and low-resource settings. Broadly, her research interests encompass Natural Language Generation, and Interpretable NLP models. Prior to Google, she was a doctoral student at IIT Madras since 2015. She was awarded Google PhD India Fellowship in 2017. She has worked in Nvidia as a System Software Engineer from 2012-2015. She finished her Bachelors from NIT Nagpur in Computer Science and Engineering in 2012.
Research Areas
Authored Publications
Sort By
Analyzing User Perspectives on Mobile App Privacy at Scale
International Conference on Software Engineering (ICSE) (2022)
Preview abstract
In this paper we present a methodology to analyze users’ concerns and perspectives about privacy at scale. We leverage NLP
techniques to process millions of mobile app reviews and extract
privacy concerns. Our methodology is composed of a binary classifier that distinguishes between privacy and non-privacy related
reviews. We use clustering to gather reviews that discuss similar
privacy concerns, and employ summarization metrics to extract
representative reviews to summarize each cluster. We apply our
methods on 287M reviews for about 2M apps across the 29 categories in Google Play to identify top privacy pain points in mobile
apps. We identified approximately 440K privacy related reviews.
We find that privacy related reviews occur in all 29 categories, with
some issues arising across numerous app categories and other issues
only surfacing in a small set of app categories. We show empirical
evidence that confirms dominant privacy themes – concerns about
apps requesting unnecessary permissions, collection of personal
information, frustration with privacy controls, tracking and the selling of personal data. As far as we know, this is the first large scale
analysis to confirm these findings based on hundreds of thousands
of user inputs. We also observe some unexpected findings such
as users warning each other not to install an app due to privacy
issues, users uninstalling apps due to privacy reasons, as well as
positive reviews that reward developers for privacy friendly apps.
Finally we discuss the implications of our method and findings for
developers and app stores.
View details
Disentangling Preference Representations for Recommendation Critiquing with β-VAE
Alexandros Karatzoglou
30th ACM International Conference on Information and Knowledge Management (CIKM 2021), ACM, New York
Preview abstract
Modern recommender systems usually embed users and items into a learned vector space representation. Similarity in this space is used to generate recommendations, and recommendation methods are agnostic to the structure of the embedding space. Motivated by the need for recommendation systems to be more transparent and controllable, we postulate that it is beneficial to assign meaning to some of the dimensions of user and item representations. Disentanglement is one technique commonly used for this purpose. We present a novel supervised disentangling approach for recommendation tasks. Our model learns embeddings where attributes of interest are disentangled, while requiring only a very small number of labeled items at training time. The model can then generate interactive and critiquable recommendations for all users, without requiring any labels at recommendation time, and without sacrificing any recommendation performance. Our approach thus provides users with levers to manipulate, critique and fine-tune recommendations, and gives insight into why particular recommendations are made. Given only user-item interactions at recommendation time, we show that it identifies user tastes with respect to the attributes that have been disentangled, allowing for users to manipulate recommendations across these attributes.
View details