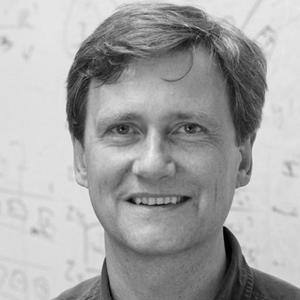
Klaus-Robert Müller
Klaus-Robert Müller has been a professor of computer science at Technische Universität Berlin since 2006; at the same time he is directing rsp. co-directing the Berlin Machine Learning Center and the Berlin Big Data Center and most recently BIFOLD . He studied physics in Karlsruhe from1984 to 1989 and obtained his Ph.D. degree in computer science at Technische Universität Karlsruhe in 1992. After completing a postdoctoral position at GMD FIRST in Berlin, he was a research fellow at the University of Tokyo from 1994 to 1995. In 1995, he founded the Intelligent Data Analysis group at GMD-FIRST (later Fraunhofer FIRST) and directed it until 2008. From 1999 to 2006, he was a professor at the University of Potsdam. From 2012 he has been Distinguished Professor at Korea University in Seoul. In 2020/2021 he spent his sabbatical at Google Brain as a Principal Scientist. Among others, he was awarded the Olympus Prize for Pattern Recognition (1999), the SEL Alcatel Communication Award (2006), the Science Prize of Berlin by the Governing Mayor of Berlin (2014), the Vodafone Innovations Award (2017), Hector Science Award (2024), Pattern Recognition Best Paper award (2020), Digital Signal Processing Best Paper award (2022). In 2012, he was elected member of the German National Academy of Sciences-Leopoldina, in 2017 of the Berlin Brandenburg Academy of Sciences, in 2021 of the German National Academy of Science and Engineering and also in 2017 external scientific member of the Max Planck Society. From 2019 on he became an ISI Highly Cited researcher in the cross-disciplinary area. His research interests are intelligent data analysis and Machine Learning in the sciences (Neuroscience (specifically Brain-Computer Interfaces, Physics, Chemistry) and in industry.
Authored Publications
Sort By
Accurate global machine learning force fields for molecules with hundreds of atoms
Stefan Chmiela
Valentin Vassilev Galindo
Adil Kabylda
Huziel E. Sauceda
Alexandre Tkatchenko
Science Advances, 9(2) (2023), eadf0873
Preview abstract
Global machine learning force fields, with the capacity to capture collective interactions in molecular systems, now scale up to a few dozen atoms due to considerable growth of model complexity with system size. For larger molecules, locality assumptions are introduced, with the consequence that nonlocal interactions are not described. Here, we develop an exact iterative approach to train global symmetric gradient domain machine learning (sGDML) force fields (FFs) for several hundred atoms, without resorting to any potentially uncontrolled approximations. All atomic degrees of freedom remain correlated in the global sGDML FF, allowing the accurate description of complex molecules and materials that present phenomena with far-reaching characteristic correlation lengths. We assess the accuracy and efficiency of sGDML on a newly developed MD22 benchmark dataset containing molecules from 42 to 370 atoms. The robustness of our approach is demonstrated in nanosecond path-integral molecular dynamics simulations for supramolecular complexes in the MD22 dataset.
View details
Canonical Response Parameterization: Quantifying the structure of responses to single-pulse intracranial electrical brain stimulation
Kai J. Miller
Gabriela Ojeda Valencia
Harvey Huang
Nicholas M. Gregg
Gregory A. Worrell
Dora Hermes
Plos Computational Biology, 19(5) (2023), e1011105
Preview abstract
Single-pulse electrical stimulation in the nervous system, often called cortico-cortical evoked potential (CCEP) measurement, is an important technique to understand how brain regions interact with one another. Voltages are measured from implanted electrodes in one brain area while stimulating another with brief current impulses separated by several seconds. Historically, researchers have tried to understand the significance of evoked voltage polyphasic deflections by visual inspection, but no general-purpose tool has emerged to understand their shapes or describe them mathematically. We describe and illustrate a new technique to parameterize brain stimulation data, where voltage response traces are projected into one another using a semi-normalized dot product. The length of timepoints from stimulation included in the dot product is varied to obtain a temporal profile of structural significance, and the peak of the profile uniquely identifies the duration of the response. Using linear kernel PCA, a canonical response shape is obtained over this duration, and then single-trial traces are parameterized as a projection of this canonical shape with a residual term. Such parameterization allows for dissimilar trace shapes from different brain areas to be directly compared by quantifying cross-projection magnitudes, response duration, canonical shape projection amplitudes, signal-to-noise ratios, explained variance, and statistical significance. Artifactual trials are automatically identified by outliers in sub-distributions of cross-projection magnitude, and rejected. This technique, which we call “Canonical Response Parameterization” (CRP) dramatically simplifies the study of CCEP shapes, and may also be applied in a wide range of other settings involving event-triggered data.
View details
Harmoni: a Method for Eliminating Spurious Interactions due to the Harmonic Components in Neuronal Data
Mina Jamshidi Idaji
Juanli Zhang
Tilman Stephani
Guido Nolte
Arno Villringer
Vadim Nikulin
Neuroimage, 252 (2022), pp. 119053
Preview abstract
Cross-frequency synchronization (CFS) has been proposed as a mechanism for integrating spatially and spectrally distributed information in the brain. However, investigating CFS in Magneto- and Electroencephalography (MEG/EEG) is hampered by the presence of spurious neuronal interactions due to the non-sinusoidal waveshape of brain oscillations. Such waveshape gives rise to the presence of oscillatory harmonics mimicking genuine neuronal oscillations. Until recently, however, there has been no methodology for removing these harmonics from neuronal data. In order to address this long-standing challenge, we introduce a novel method (called HARMOnic miNImization - Harmoni) that removes the signal components which can be harmonics of a non-sinusoidal signal. Harmoni’s working principle is based on the presence of CFS between harmonic components and the fundamental component of a non-sinusoidal signal. We extensively tested Harmoni in realistic EEG simulations. The simulated couplings between the source signals represented genuine and spurious CFS and within-frequency phase synchronization. Using diverse evaluation criteria, including ROC analyses, we showed that the within- and cross-frequency spurious interactions are suppressed significantly, while the genuine activities are not affected. Additionally, we applied Harmoni to real resting-state EEG data revealing intricate remote connectivity patterns which are usually masked by the spurious connections. Given the ubiquity of non-sinusoidal neuronal oscillations in electrophysiological recordings, Harmoni is expected to facilitate novel insights into genuine neuronal interactions in various research fields, and can also serve as a steppingstone towards the development of further signal processing methods aiming at refining within- and cross-frequency synchronization in electrophysiological recordings.
View details
So3krates - Self-attention for higher-order geometric interactions on arbitrary length-scales
Thorben Frank
Advances in Neural Information Processing Systems (2022) (to appear)
Preview abstract
The application of machine learning (ML) methods in quantum chemistry has enabled the study of numerous chemical phenomena, which are computationally intractable with traditional ab initio methods. However, some quantum mechanical properties of molecules and materials depend on non-local electronic effects, which are often neglected due to the difficulty of modelling them efficiently. This work proposes a modified attention mechanism adapted to the underlying physics, which allows to recover the relevant non-local effects. Namely, we introduce spherical harmonic coordinates (SPHCs) to reflect higher order geometric information for each atom in a molecule, enabling a non-local formulation of attention in the SPHC space. Our proposed model So3krates -- a self-attention based message passing neural network (MPNN) -- uncouples geometric information from atomic features, making them independently amenable to attention mechanisms. We show that in contrast to other published methods, So3krates is able to describe quantum mechanical effects due to orbital overlap over arbitrary length scales. Further, So3krates is shown to match or exceed state-of-the-art performance on the popular MD-17 and QM-7X benchmarks, notably, requiring a significantly lower number of parameters while at the same time giving a substantial speedup compared to other models.
View details
BIGDML—Towards accurate quantum machine learning force fields for materials
Huziel Sauceda
Luis Gálvez-González
Stefan Chmiela
Lauro Oliver Paz Borbon
Alexandre Tkatchenko
Nature Communications, 13 (2022), pp. 3733
Preview abstract
Machine-learning force fields (MLFF) should be accurate, computationally and data efficient, and applicable to molecules, materials, and interfaces thereof. Currently, MLFFs often introduce tradeoffs that restrict their practical applicability to small subsets of chemical space or require exhaustive datasets for training. Here, we introduce the Bravais-Inspired Gradient-Domain Machine Learning (BIGDML) approach and demonstrate its ability to construct reliable force fields using a training set with just 10–200 geometries for materials including pristine and defect-containing 2D and 3D semiconductors and metals, as well as chemisorbed and physisorbed atomic and molecular adsorbates on surfaces. The BIGDML model employs the full relevant symmetry group for a given material, does not assume artificial atom types or localization of atomic interactions and exhibits high data efficiency and state-of-the-art energy accuracies (errors substantially below 1 meV per atom) for an extended set of materials. Extensive path-integral molecular dynamics carried out with BIGDML models demonstrate the counterintuitive localization of benzene–graphene dynamics induced by nuclear quantum effects and their strong contributions to the hydrogen diffusion coefficient in a Pd crystal for a wide range of temperatures.
View details
Artificial Intelligence and Pathology: from Principles to Practice and Future Applications in Histomorphology and Molecular Profiling
Albrecht Stenzinger
Max Alber
Michael Allgäuer
Phillip Jurmeister
Michael Bockmayr
Jan Budczies
Jochen Lennerz
Johannes Eschrich
Daniel Kazdal
Peter Schirmacher
Alex H Wagner
Frank Tacke
David Capper
Frederick Klauschen
Seminars in Cancer Biology, 84 (2022), pp. 129-143
Preview abstract
The complexity of diagnostic (surgical) pathology has increased substantially over the last
decades with respect to histomorphological and molecular profiling and has steadily expanded
its role in tumor diagnostics and beyond from disease entity identification via prognosis
estimation to precision therapy prediction. It is therefore not surprising that pathology is among
the disciplines in medicine with high expectations in the application of artificial intelligence (AI)
or machine learning approaches given its capabilities to analyse complex data in a quantitative
and standardised manner to further enhance scope and precision of diagnostics. While an
obvious application is the analysis of histological images, recent applications for the analysis
of molecular profiling data from different sources and clinical data support the notion that AI
will support both histopathology and molecular pathology in the future. At the same time,
current literature should not be misunderstood in a way that pathologists will likely be replaced
by AI applications in the foreseeable future. Although AI will likely transform pathology in the
coming years, recent studies reporting AI algorithms to diagnose cancer or predict certain
molecular properties deal with relatively simple diagnostic problems that fall short of the
diagnostic complexity pathologists face in clinical routine. Here, we review the pertinent
literature of AI methods and their applications to pathology, and put the current achievements
and what can be expected in the future in the context of the requirements for research and
routine diagnostics.
View details
Algorithmic Differentiation for Automatized Modelling of Machine Learned Force Fields
Niklas Schmitz
Stefan Chmiela
The Journal of Physical Chemistry Letters, 13(43) (2022), pp. 10183-10189
Preview abstract
Reconstructing force fields (FFs) from atomistic simulation data is a challenge since accurate data can be highly expensive. Here, machine learning (ML) models can help to be data economic as they can be successfully constrained using the underlying symmetry and conservation laws of physics. However, so far, every descriptor newly proposed for an ML model has required a cumbersome and mathematically tedious remodeling. We therefore propose using modern techniques from algorithmic differentiation within the ML modeling process, effectively enabling the usage of novel descriptors or models fully automatically at an order of magnitude higher computational efficiency. This paradigmatic approach enables not only a versatile usage of novel representations and the efficient computation of larger systems─all of high value to the FF community─but also the simple inclusion of further physical knowledge, such as higher-order information (e.g., Hessians, more complex partial differential equations constraints etc.), even beyond the presented FF domain.
View details
Towards Robust Explanations for Deep Neural Networks
Ann-Kathrin Dombrowski
Christopher Johannes Anders
Pan Kessel
Pattern Recognition, 121 (2022), pp. 108194
Preview abstract
Explanation methods shed light on the decision process of black-box classifiers such as deep neural networks. But their usefulness can be compromised because they are susceptible to manipulations. With this work, we aim to enhance the resilience of explanations. We develop a unified theoretical framework for deriving bounds on the maximal manipulability of a model. Based on these theoretical insights, we present three different techniques to boost robustness against manipulation: training with weight decay, smoothing activation functions, and minimizing the Hessian of the network. Our experimental results confirm the effectiveness of these approaches.
View details
Super-resolution in Molecular Dynamics Trajectory Reconstruction with Bi-Directional Neural Networks
Paul Ludwig Winkler
Huziel Saucceda
Machine Learning: Science and Technology, 3 (2022), pp. 025011
Preview abstract
Molecular dynamics (MD) simulations are a cornerstone in science, enabling the investigation of a
system’s thermodynamics all the way to analyzing intricate molecular interactions. In general,
creating extended molecular trajectories can be a computationally expensive process, for example,
when running ab-initio simulations. Hence, repeating such calculations to either obtain more
accurate thermodynamics or to get a higher resolution in the dynamics generated by a fine-grained
quantum interaction can be time- and computational resource-consuming. In this work, we
explore different machine learning methodologies to increase the resolution of MD trajectories
on-demand within a post-processing step. As a proof of concept, we analyse the performance of
bi-directional neural networks (NNs) such as neural ODEs, Hamiltonian networks, recurrent NNs
and long short-term memories, as well as the uni-directional variants as a reference, for MD
simulations (here: the MD17 dataset). We have found that Bi-LSTMs are the best performing
models; by utilizing the local time-symmetry of thermostated trajectories they can even learn
long-range correlations and display high robustness to noisy dynamics across molecular
complexity. Our models can reach accuracies of up to 10−4 Å in trajectory interpolation, which
leads to the faithful reconstruction of several unseen high-frequency molecular vibration cycles.
This renders the comparison between the learned and reference trajectories indistinguishable. The
results reported in this work can serve (1) as a baseline for larger systems, as well as (2) for the
construction of better MD integrators.
View details
Efficient Computation of Higher-Order Subgraph Attribution via Message Passing
Ping Xiong
Thomas Schnake
Gregoire Montavon
Shin Nakajima
ICML (2022) (to appear)
Preview abstract
Explaining graph neural networks (GNNs) has become more and more important recently. Higherorder interpretation schemes, such as GNNLRP (layer-wise relevance propagation for GNN),
emerged as powerful tools for unraveling how
different features interact thereby contributing to
explaining GNNs. Methods such as GNN-LRP
perform walks between nodes at each layer, and
there are exponentially many such walks. In this
work, we demonstrate that such exponential complexity can be avoided, in particular, we propose
novel linear-time (w.r.t. depth) algorithms that
enable to efficiently perform GNN-LRP for subgraphs. Our algorithms are derived via message
passing techniques that make use of the distributive property, thereby directly computing quantities for higher-order explanations. We further
adapt our efficient algorithms to compute a generalization of subgraph attributions that also takes
into account the neighboring graph features. Experimental results show significant acceleration of
the proposed algorithms and demonstrate a high
usefulness and scalability of our novel generalized
subgraph attribution.
View details