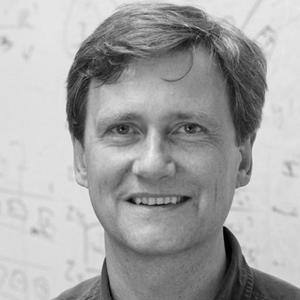
Klaus-Robert Müller
Klaus-Robert Müller has been a professor of computer science at Technische Universität Berlin since 2006; at the same time he is directing rsp. co-directing the Berlin Machine Learning Center and the Berlin Big Data Center and most recently BIFOLD . He studied physics in Karlsruhe from1984 to 1989 and obtained his Ph.D. degree in computer science at Technische Universität Karlsruhe in 1992. After completing a postdoctoral position at GMD FIRST in Berlin, he was a research fellow at the University of Tokyo from 1994 to 1995. In 1995, he founded the Intelligent Data Analysis group at GMD-FIRST (later Fraunhofer FIRST) and directed it until 2008. From 1999 to 2006, he was a professor at the University of Potsdam. From 2012 he has been Distinguished Professor at Korea University in Seoul. In 2020/2021 he spent his sabbatical at Google Brain as a Principal Scientist. Among others, he was awarded the Olympus Prize for Pattern Recognition (1999), the SEL Alcatel Communication Award (2006), the Science Prize of Berlin by the Governing Mayor of Berlin (2014), the Vodafone Innovations Award (2017), Hector Science Award (2024), Pattern Recognition Best Paper award (2020), Digital Signal Processing Best Paper award (2022). In 2012, he was elected member of the German National Academy of Sciences-Leopoldina, in 2017 of the Berlin Brandenburg Academy of Sciences, in 2021 of the German National Academy of Science and Engineering and also in 2017 external scientific member of the Max Planck Society. From 2019 on he became an ISI Highly Cited researcher in the cross-disciplinary area. His research interests are intelligent data analysis and Machine Learning in the sciences (Neuroscience (specifically Brain-Computer Interfaces, Physics, Chemistry) and in industry.
Authored Publications
Sort By
Google
Canonical Response Parameterization: Quantifying the structure of responses to single-pulse intracranial electrical brain stimulation
Kai J. Miller
Gabriela Ojeda Valencia
Harvey Huang
Nicholas M. Gregg
Gregory A. Worrell
Dora Hermes
Plos Computational Biology, 19(5) (2023), e1011105
Accurate global machine learning force fields for molecules with hundreds of atoms
Stefan Chmiela
Valentin Vassilev Galindo
Adil Kabylda
Huziel E. Sauceda
Alexandre Tkatchenko
Science Advances, 9(2) (2023), eadf0873
Toward Explainable Artificial Intelligence for Regression Models: A methodological perspective
Simon Letzgus
Jonas Lederer
Wojciech Samek
Gregoire Montavon
IEEE Signal Processing Magazine, 39 (4) (2022), 40–58
Harmoni: a Method for Eliminating Spurious Interactions due to the Harmonic Components in Neuronal Data
Mina Jamshidi Idaji
Juanli Zhang
Tilman Stephani
Guido Nolte
Arno Villringer
Vadim Nikulin
Neuroimage, 252 (2022), pp. 119053
Artificial Intelligence and Pathology: from Principles to Practice and Future Applications in Histomorphology and Molecular Profiling
Albrecht Stenzinger
Max Alber
Michael Allgäuer
Phillip Jurmeister
Michael Bockmayr
Jan Budczies
Jochen Lennerz
Johannes Eschrich
Daniel Kazdal
Peter Schirmacher
Alex H Wagner
Frank Tacke
David Capper
Frederick Klauschen
Seminars in Cancer Biology, 84 (2022), pp. 129-143
Super-resolution in Molecular Dynamics Trajectory Reconstruction with Bi-Directional Neural Networks
Paul Ludwig Winkler
Huziel Saucceda
Machine Learning: Science and Technology, 3 (2022), pp. 025011
So3krates - Self-attention for higher-order geometric interactions on arbitrary length-scales
Thorben Frank
Advances in Neural Information Processing Systems (2022) (to appear)
Higher-Order Explanations of Graph Neural Networks via Relevant Walks
Thomas Schnake
Oliver Eberle
Jonas Lederer
Shin Nakajima
Kristof T. Schütt
Gregoire Montavon
IEEE Transactions on Pattern Analysis and Machine Intelligence, 44(11) (2022), pp. 7581 - 7596
Efficient Computation of Higher-Order Subgraph Attribution via Message Passing
Ping Xiong
Thomas Schnake
Gregoire Montavon
Shin Nakajima
ICML (2022) (to appear)
Towards Robust Explanations for Deep Neural Networks
Ann-Kathrin Dombrowski
Christopher Johannes Anders
Pan Kessel
Pattern Recognition, 121 (2022), pp. 108194