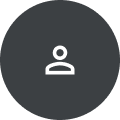
Anshuman Tripathi
Research Areas
Authored Publications
Sort By
Google
End-to-end audio-visual speech recognition for overlapping speech
Olivier Siohan
INTERSPEECH 2021: Conference of the International Speech Communication Association
Turn-To-Diarize: Online speaker diarization constrained by transformer transducer speaker turn detection
Han Lu
Wei Xia
Submitted to ICASSP 2022, IEEE (2021)
Multilingual Speech Recognition with Self-Attention Structured Parameterization
Yun Zhu
Brian Farris
Hainan Xu
Han Lu
Pedro Jose Moreno Mengibar
Qian Zhang
Interspeech 2020, 21st Annual Conference of the International Speech Communication Association, ISCA
Speech recognition for medical conversations
Chung-Cheng Chiu
Kat Chou
Chris Co
Navdeep Jaitly
Diana Jaunzeikare
Patrick Nguyen
Ananth Sankar
Justin Jesada Tansuwan
Nathan Wan
Yonghui Wu
Frank Zhang
Interspeech 2018 (2018)
TEMPORAL MODELING USING DILATED CONVOLUTION AND GATING FOR VOICE-ACTIVITY-DETECTION
Gabor Simko
Aäron van den Oord
ICASSP 2018