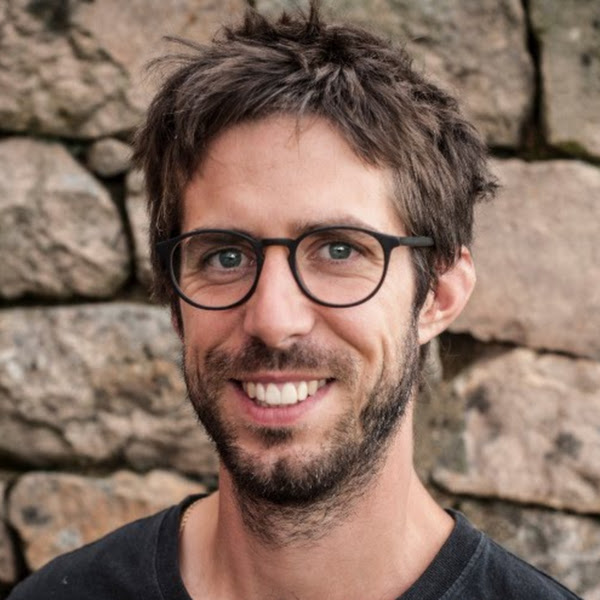
Gabriel Dulac-Arnold
Gabriel first joined Google a Research Scientist at DeepMind where he worked on bringing reinforcement learning into real-world problems. While there he worked on many Google-related problems, namely reducing the energy usage of Google data centers using reinforcement learning. At Brain, Gabriel now works on general problems related to using reinforcement learning in real-world systems, and more generally in algorithmic barriers to wider adoption of machine learning in real systems.
Research Areas
Authored Publications
Sort By
Challenges of Real-World Reinforcement Learning:Definitions, Benchmarks & Analysis
Cosmin Paduraru
Daniel J. Mankowitz
Jerry Li
Nir Levine
Todd Hester
Machine Learning Journal (2021)
Preview abstract
Reinforcement learning (RL) has proven its worth in a series of artificialdomains, and is beginning to show some successes in real-world scenarios. However,much of the research advances in RL are hard to leverage in real-world systemsdue to a series of assumptions that are rarely satisfied in practice. We identifyand formalize a series of independent challenges that embody the difficulties thatmust be addressed for RL to be commonly deployed in real-world systems. Foreach challenge, we define it formally in the context of a Markov Decision Process,analyze the effects of the challenge on state-of-the-art learning algorithms, andpresent some existing attempts at tackling it. We believe that an approach thataddresses all nine challenges would be readily deployable in a large number of realworld problems. We implement our proposed challenges in a suite of continuouscontrol environments calledrealworldrl-suitewhich we propose an as an open-source benchmark.
View details
Preview abstract
Offline learning is a key part of making reinforcement learning (RL) useable in real systems. Offline RL looks at scenarios where there is data from a system's operation, but no direct access to the system when learning a policy. Recent work on training RL policies from offline data has shown results where a model-free policy is learned either from the data, or a modelled representation of the data. Model-free policies tend to be more performant, but are more opaque, harder to command externally, and less easy to integrate into larger systems. We propose an offline learner that generates a model that can be used to control the system directly through planning. This allows us to have easily controllable policies directly from data, without ever interacting with the system. We show the performance of our algorithm, Model-Based Offline Planning (MBOP) on a series of robotics-inspired tasks, and demonstrate its ability leverage planning to respect environmental constraints. We are able to create goal-conditioned polices for certain simulated systems from as little as 100 seconds of real-time system interaction.
View details
AI-based mobile application to fight antibiotic resistance
Marco Pascucci
Guilhem Royer
Jakub Adámek
Mai Al Asmar
David Aristizabal
Laetitia Blanche
Amine Bezzarga
Guillaume Boniface-Chang
Alex Brunner
Christian Curel
Rasheed M. Fakhri
Nada Malou
Clara Nordon
Vincent Runge
Franck Samson
Ellen Marie Sebastian
Dena Soukieh
Jean-Philippe Vert
Christophe Ambroise
Mohammed-Amin Madoui
Nature Communications, 12 (2021), pp. 1173
Preview abstract
Antimicrobial resistance is a major global health threat and its development is promoted by antibiotic misuse. While disk diffusion antibiotic susceptibility testing (AST, also called antibiogram) is broadly used to test for antibiotic resistance in bacterial infections, it faces strong criticism because of inter-operator variability and the complexity of interpretative reading. Automatic reading systems address these issues, but are not always adapted or available to resource-limited settings. We present NewAppName, the first artificial intelligence (AI)-based, offline smartphone application for antibiogram analysis. NewAppName captures images with the phone’s camera, and the user is guided throughout the analysis on the same device by a user-friendly graphical interface. An embedded expert system validates the coherence of the antibiogram data and provides interpreted results. The fully automatic measurement procedure of NewAppName’s reading system achieves an overall agreement of 90 % on susceptibility categorization against a hospital-standard automatic system and 98 % against manual measurement (gold standard), with reduced inter-operator variability. NewAppName performance showed that the automatic reading of antibiotic resistance testing is entirely feasible on a smartphone. NewAppName is suited for resource-limited settings, and therefore has the potential to significantly increase patients’ access to AST worldwide.
View details
A Geometric Perspective on Self-Supervised Policy Adaptation
Cristian Bodnar
Karol Hausman
Rico Jonschkowski
NeurIPS Workshop on Challenges of Real-World RL (2020)
Preview abstract
One of the most challenging aspects of real-world reinforcement learning (RL) is the multitude of unpredictable and ever-changing distractions that could divert an agent from what was tasked to do in its training environment. While an agent could learn from reward signals to ignore them, the complexity of the real-world can make rewards hard to acquire, or, at best, extremely sparse. A recent class of self-supervised methods have shown promise that reward-free adaptation under challenging distractions is possible. However, previous work focused on a short one-episode adaptation setting. In this paper, we consider a long-term adaptation setup that is more akin to the specifics of the real-world and propose a geometric perspective on self-supervised adaptation. We empirically describe the processes that take place in the embedding space during this adaptation process, reveal some of its undesirable effects on performance and show how they can be eliminated. Moreover, we theoretically study how actor-based and actor-free agents can further generalise to the target environment by manipulating the geometry of the manifolds described by the actor and critic functions.
View details
Preview abstract
We propose a learning algorithm capable of learning from label proportions instead of direct data labels. In this scenario, our data are arranged into various bags of a certain size, and only the proportions of each label within a given bag are known. This is a common situation in cases where per-data labeling is lengthy, but a more general label is easily accessible. Several approaches have been proposed to learn in this setting with linear models in the multiclass setting, or with nonlinear models in the binary classification setting. Here we investigate the more general nonlinear multiclass setting, and compare two differentiable loss functions to train end-to-end deep neural networks from bags with label proportions. We illustrate the relevance of our methods on an image classification benchmark, and demonstrate the possibility to learn accurate image classifiers from bags of images.
View details
Preview abstract
Clustering is a fundamental unsupervised learning approach. Many clustering algorithms -- such as $k$-means -- rely on the euclidean distance as a similarity measure, which is often not the most relevant metric for high dimensional data such as images. Learning a lower-dimensional embedding that can better reflect the geometry of the dataset is therefore instrumental for performance. We propose a new approach for this task where the embedding is performed by a differentiable model such as a deep neural network. By rewriting the $k$-means clustering algorithm as an optimal transport task, and adding an entropic regularization, we derive a fully differentiable loss function that can be minimized with respect to both the embedding parameters and the cluster parameters via stochastic gradient descent. We show that this new formulation generalizes a recently proposed state-of-the-art method based on soft-$k$-means by adding constraints on the cluster sizes. Empirical evaluations on image classification benchmarks suggest that compared to state-of-the-art methods, our optimal transport-based approach provide better unsupervised accuracy and does not require a pre-training phase.
View details
Challenges of Real-World Reinforcement Learning
Daniel J. Mankowitz
Todd Hester
ICML Workshop on Real-Life Reinforcement Learning (2019)
Preview abstract
Reinforcement learning (RL) has proven its worth in a series of artificial domains, and is beginning to show some successes in real-world scenarios. However, much of the research advances in RL are often hard to leverage in real-world systems due to a series of assumptions that are rarely satisfied in practice. We present a set of nine unique challenges that must be addressed to productionize RL to real world problems. For each of these challenges, we specify the exact meaning of the challenge, present some approaches from the literature, and specify some metrics for evaluating that challenge. An approach that addresses all nine challenges would be applicable to a large number of real world problems. We also present a toy example domain that has been modified to present these challenges as a testbed for practical RL research.
View details
Deep Q-learning from Demonstrations
Todd Hester
Matej Vecerik
Olivier Pietquin
Marc Lanctot
Tom Schaul
Bilal Piot
Dan Horgan
John Quan
Andrew Sendonaris
Ian Osband
John Agapiou
Joel Z Leibo
Audrunas Gruslys
Annual Meeting of the Association for the Advancement of Artificial Intelligence (AAAI), New Orleans (USA) (2018)
Preview abstract
Deep reinforcement learning (RL) has achieved several
high profile successes in difficult decision-making problems.
However, these algorithms typically require a huge amount of
data before they reach reasonable performance. In fact, their
performance during learning can be extremely poor. This may
be acceptable for a simulator, but it severely limits the appli-
cability of deep RL to many real-world tasks, where the agent
must learn in the real environment. In this paper we study a
setting where the agent may access data from previous con-
trol of the system. We present an algorithm, Deep Q-learning
from Demonstrations (DQfD), that leverages small sets of
demonstration data to massively accelerate the learning pro-
cess even from relatively small amounts of demonstration
data and is able to automatically assess the necessary ratio
of demonstration data while learning thanks to a prioritized
replay mechanism. DQfD works by combining temporal dif-
ference updates with supervised classification of the demon-
strator’s actions. We show that DQfD has better initial per-
formance than Prioritized Dueling Double Deep Q-Networks
(PDD DQN) as it starts with better scores on the first million
steps on 41 of 42 games and on average it takes PDD DQN
83 million steps to catch up to DQfD’s performance. DQfD
learns to out-perform the best demonstration given in 14 of
42 games. In addition, DQfD leverages human demonstra-
tions to achieve state-of-the-art results for 11 games. Finally,
we show that DQfD performs better than three related algo-
rithms for incorporating demonstration data into DQN.
View details