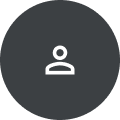
Andrei Kapishnikov
Authored Publications
Sort By
Google
Beyond Rewards: a Hierarchical Perspective on Offline Multiagent Behavioral Analysis
Shayegan Omidshafiei
Yannick Assogba
Advances in Neural Information Processing Systems (NeurIPS) (2022) (to appear)
Guided Integrated Gradients: An Adaptive Path Method for Removing Noise
Besim Namik Avci
Proceedings of the IEEE/CVF Conference on Computer Vision and Pattern Recognition (CVPR), 2021, pp. 5050-5058
Evaluation of the Use of Combined Artificial Intelligence and Pathologist Assessment to Review and Grade Prostate Biopsies
Kunal Nagpal
Davis J. Foote
Adam Pearce
Samantha Winter
Matthew Symonds
Liron Yatziv
Trissia Brown
Isabelle Flament-Auvigne
Fraser Tan
Martin C. Stumpe
Cameron Chen
Craig Mermel
JAMA Network Open (2020)
XRAI: Better Attributions Through Regions
International Conference on Computer Vision 2019 (ICCV) (2019) (to appear)