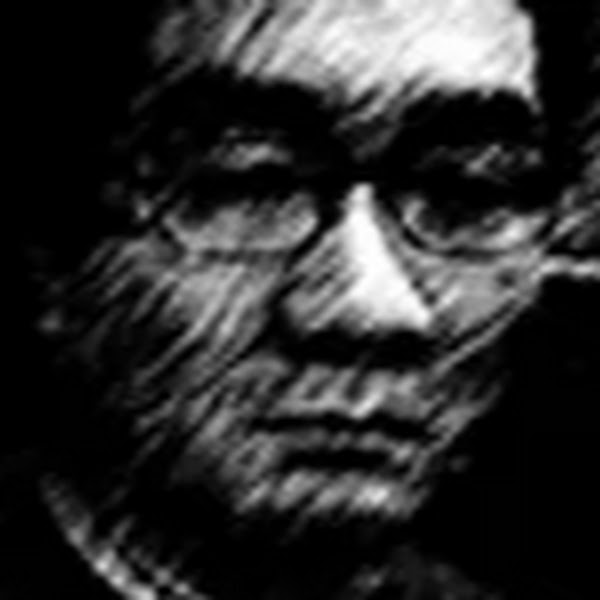
Juhyun Lee
Juhyun is a software engineer at Google LLC, where he leads efforts in GPU-accelerated machine learning inference across platforms. Before joining Google, he earned his Ph.D. in Computer Sciences from the University of Texas at Austin, focusing on compute vision for mobile robots. His work bridges cutting-edge GPU technology with scalable ML solutions, aiming to set new standards for universal GPU compute.
Research Areas
Authored Publications
Sort By
Google
Efficient Heterogeneous Video Segmentation at the Edge
Jamie Lin
Siargey Pisarchyk
David Cong Tian
Tingbo Hou
George Sung
Sixth Workshop on Computer Vision for AR/VR (CV4ARVR) (2022)
On-Device Neural Net Inference with Mobile GPUs
Nikolay Chirkov
Ekaterina Ignasheva
Yury Pisarchyk
Mogan Shieh
Fabio Riccardi
Efficient Deep Learning for Computer Vision CVPR 2019 (ECV2019) (to appear)
MediaPipe: A Framework for Perceiving and Processing Reality
Camillo Lugaresi
Jiuqiang Tang
Hadon Nash
Chris McClanahan
Esha Uboweja
Michael Hays
Fan Zhang
Chuo-Ling Chang
Ming Yong
Wan-Teh Chang
Wei Hua
Manfred Georg
Third Workshop on Computer Vision for AR/VR at IEEE Computer Vision and Pattern Recognition (CVPR) 2019