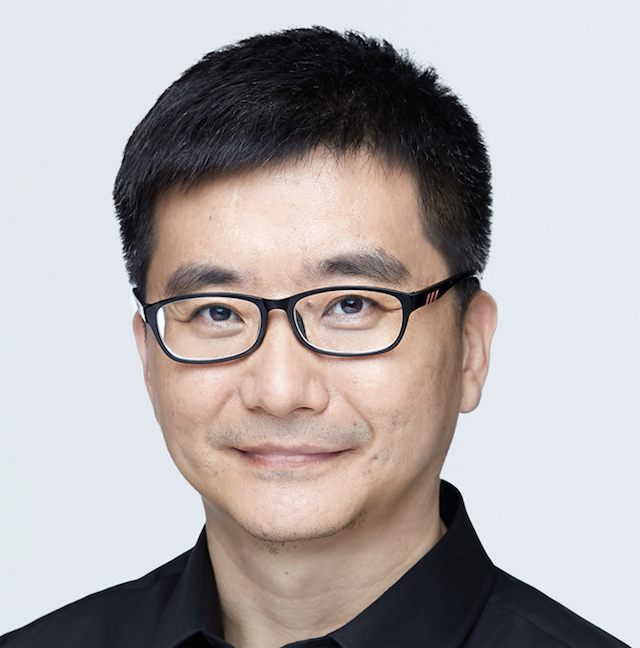
Jingtao Wang
Dr. Jingtao Wang is a Research Scientist and Tech Lead Manager at Google. His research interests include - large generative models, on-device machine learning, and educational technology. Before joining Google, Dr. Wang was an Assistant Professor of Computer Science at the University of Pittsburgh. He was the recipient of a Microsoft Azure for Research Award, a Google Faculty Research Award, and an ACIE Innovation in Education Award. Dr. Wang received his Ph.D. degree in Computer Science from the University of California, Berkeley.
Authored Publications
Sort By
StrategicReading: Understanding Complex Mobile Reading Strategies via Implicit Behavior Sensing
Wei Guo
Byeong-Young Cho
Proceedings of ACM International Conference on Multimodal Interaction (ICMI 2020), ACM
Preview abstract
Mobile devices are becoming an important platform for reading. However, existing research on mobile reading primarily focuses on low-level metrics such as speed and comprehension. In particular, for complex reading tasks involving information seeking, source evaluation, and integrative comprehension, researchers still rely on the labor-intensive analysis of reader-generated verbal reports. We present StrategicReading, an intelligent reading system running on unmodified smartphones, to understand high-level strategic reading behaviors on mobile devices. StrategicReading leverages multimodal behavior sensing and takes advantage of signals from camera-based gaze sensing, kinematic scrolling patterns, and the evolution of cross-page behaviors. Through a 40-participant study, we found that gaze patterns, muscle stiffness signals, and reading paths captured by StrategicReading can infer both users' reading strategies and reading performance with high accuracy.
View details
Towards Web-based Etymological Hanzi learning
Genze Wu
Jia Xing
Julia (Wenli) Zhu
Jun Chen
Kevin Jing
Sijia Ma
Wenhui Guo
Yaolin Chen
Yingying Zhao
(2020)
Preview abstract
Modern-day Chinese characters, or Hanzi, originate from the ancient oracle-bone scripts (甲骨文). Such etymological relationship creates unique opportunities for Chinese literacy learning. This work proposes to use Web-based tools and the latest machine learning techniques to scale-up and enhance etymological Hanzi learning. By sharing our implementation details from launching an interactive sketch-based learning exhibition, we hope education-AI becomes more widely incorporated into today’s commercial Web applications.
View details
Living Jiagu : Enabling Constructive Etymology for Chinese Learning
Sijia Ma
Jun Chen
Wenhui Guo
Yingying Zhao
Yaolin Chen
Kevin Jing
Julia (Wenli) Zhu
Extended Abstracts of the 2020 CHI Conference on Human Factors in Computing Systems, ACM, pp. 1-4
Preview abstract
Living Jiagu is an interactive, wall-sized exhibition for the engaging learning of Chinese writing. Living Jiagu leverages state-of-the-art machine learning technologies to enable the recognition and recall of Chinese characters via constructive etymology in context. That is, learning the writing and meaning of a pictographic character from image prompts similar to the creators of Oracle Bone Script (OBS) 3000 years ago and experiencing how these characters function and interact in natural scene. An installation of Living Jiagu received positive feedback from over one thousand users.
View details
Improving Hindi Decoding Skills via a Mobile Game
Adeetee Bhide
Wencan Luo
Nivita Vijay
Charles Perfetti
Adrian Maries
Sonali Nag
Reading and Writing, 2019(2019), pp. 1-30
Preview abstract
Previous research with alphasyllabaries has shown that children struggle with akshara that have two or more consonants, known as complex akshara. We developed a mobile game that teaches 4th grade children Hindi decoding skills, with an emphasis on complex akshara. All of the children were second language learners of Hindi. There were two versions of the game that varied in terms of stimuli spacing (massed and distributed). We found that the game improved participants’ akshara recognition and their ability to read and spell words that contain complex akshara. There is also evidence of learning in the online data; participants were able to more quickly arrive at the correct answers as the game progressed. Both versions of the game yielded equivalent levels of improvement, but participants played the massed version faster. The spacing results are interpreted using the desirable difficulties framework. Overall, the results suggest that mobile technology can effectively improve akshara knowledge.
View details
Preview abstract
Understanding a target audience’s emotional responses to a video advertisement is crucial to evaluate the advertisement’s effectiveness. However, traditional methods for collecting such information are slow, expensive, and coarse-grained. We propose AttentiveVideo, a scalable intelligent mobile interface with corresponding inference algorithms to monitor and quantify the effects of mobile video advertising in real time. Without requiring additional sensors, AttentiveVideo employs a combination of implicit photoplethysmography (PPG) sensing and facial expression analysis (FEA) to detect the attention, engagement, and sentiment of viewers as they watch video advertisements on unmodified smartphones. In a 24-participant study, AttentiveVideo achieved good accuracy on a wide range of emotional measures (the best average accuracy = 82.6% across 9 measures). While feature fusion alone did not improve prediction accuracy with a single model, it significantly improved the accuracy when working together with model fusion. We also found that the PPG sensing channel and the FEA technique have different strength in data availability, latency detection, accuracy, and usage environment. These findings show the potential for both low-cost collection and deep understanding of emotional responses to mobile video advertisements.
View details
Adaptive Review for Mobile MOOC Learning via Multimodal Physiological Signal Sensing - a Longitudinal Study
Phuong Pham
Proceedings of the 20th ACM International Conference on Multimodal Interaction, ACM, New York, NY, USA(2018)
Preview abstract
Despite the great potential, Massive Open Online Courses (MOOCs) face major challenges such as low retention rate, limited feedback, and lack of personalization. In this paper, we report the results of a longitudinal study on AttentiveReview2, a multimodal intelligent tutoring system optimized for MOOC learning on unmodified mobile devices. AttentiveReview2 continuously monitors learners’ physiological signals, facial expressions, and touch interactions during learning and recommends personalized review materials by predicting each learner’s perceived difficulty on each learning topic. In a 3-week study involving 28 learners, we found that AttentiveReview2 on average improved learning gains by 21.8% in weekly tests. Follow-up analysis shows that multi-modal signals collected from the learning process can also benefit instructors by providing rich and fine-grained insights on the learning progress. Taking advantage of such signals also improves prediction accuracies in emotion and test scores when compared with clickstream analysis.
View details
Understanding Mobile Reading via Camera Based Gaze Tracking and Kinematic Touch Modeling
Wei Guo
Proceedings of the 20th ACM International Conference on Multimodal Interaction, ACM, New York, NY, USA(2018)
Preview abstract
Despite the ubiquity and rapid growth of mobile reading activities, researchers and practitioners today either rely on coarse-grained metrics such as click-through-rate (CTR) and dwell time, or expensive equipment such as gaze trackers to understand users’ reading behavior on mobile devices. We present Lepton, an intelligent mobile reading system and a set of dual-channel sensing algorithms to achieve scalable and fine-grained understanding of users’ reading behaviors, comprehension, and engagements on unmodified smartphones. Lepton tracks the periodic lateral patterns, i.e. saccade, of users’ eye gaze via the front camera, and infers their muscle stiffness during text scrolling via a Mass-Spring-Damper (MSD) based kinematic model from touch events. Through a 25-participant study, we found that both the periodic saccade patterns and muscle stiffness signals captured by Lepton can be used as expressive features to infer users’ comprehension and engagement in mobile reading. Overall, our new signals lead to significantly higher performances in predicting users’ comprehension (correlation: 0.36 vs. 0.29), concentration (0.36 vs. 0.16), confidence (0.5 vs. 0.47), and engagement (0.34 vs. 0.16) than using traditional dwell-time based features via a user-independent model.
View details
Towards Attentive Speed Reading on Small Screen Wearable Devices
Wei Guo
Proceedings of the 20th ACM International Conference on Multimodal Interaction, ACM, New York, NY, USA(2018)
Preview abstract
Smart watches can enrich everyday interactions by providing both glanceable information and instant access to frequent tasks. However, reading text messages on a 1.5-inch small screen is inherently challenging, especially when a user’s attention is divided. We present SmartRSVP, an attentive speed-reading system to facilitate text reading on small-screen wearable devices. SmartRSVP leverages camera-based visual attention tracking and implicit physiological signal sensing to make text reading via Rapid Serial Visual Presentation (RSVP) more enjoyable and practical on smart watches. Through a series of three studies involving 40 participants, we found that 1) SmartRSVP can achieve a significantly higher comprehension rate (57.5% vs. 23.9%) and perceived comfort (3.8 vs. 2.1) than traditional RSVP; 2) Users prefer SmartRSVP over traditional reading interfaces when they walk and read; 3) SmartRSVP can predict users’ cognitive workloads and adjust the reading speed accordingly in real-time with 83.3% precision.
View details