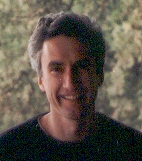
Richard Rose
Rick Rose has been a research scientist at Google in New York City since October, 2014. While at Google he has contributed to efforts in far-field speech recognition, including multi-style training, data perturbation, and multi-channel microphone dereverberation. More recently, he has been working on acoustic modeling for ASR in YouTube videos. Before coming to Google, he served as a Professor of Electrical and Computer Engineering at McGill University in Montreal since 2004, as a member of research staff at AT&T Labs / Bell Labs, and member of staff at MIT Lincoln Labs. He received his PhD degree in Electrical Engineering from the Georgia Institute of Technology. He has been active in the IEEE Signal Processing Society. He has served twice as an Associate Editor of IEEE SPS Transactions, twice as a member of the Speech Technical Committee, as member of the SPS Board of Directors, and several times on organizing committees of IEEE workshops. He is a Fellow of the IEEE.
Research Areas
Authored Publications
Sort By
Google
End-to-end audio-visual speech recognition for overlapping speech
Olivier Siohan
INTERSPEECH 2021: Conference of the International Speech Communication Association
Acoustic Modeling for Google Home
Joe Caroselli
Kean Chin
Chanwoo Kim
Olivier Siohan
Mitchel Weintraub
Erik McDermott
INTERSPEECH 2017 (2017)
Automatic Optimization of Data Perturbation Distributions for Multi-Style Training in Speech Recognition
Mortaza Doulaty
Olivier Siohan
Proceedings of the IEEE 2016 Workshop on Spoken Language Technology (SLT2016)