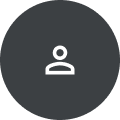
Xinyu Qian
Authored Publications
Sort By
Google
Bootstrapping Recommendations at Chrome Web Store
Po Hu
Chary Chen
Mike Bendersky
27th ACM SIGKDD Conference on Knowledge Discovery and Data Mining (KDD) (2021)
Cross-Positional Attention for Debiasing Clicks
Mike Bendersky
Po Hu
Chary Chen
Proceedings of the Web Conference 2021 (WWW)