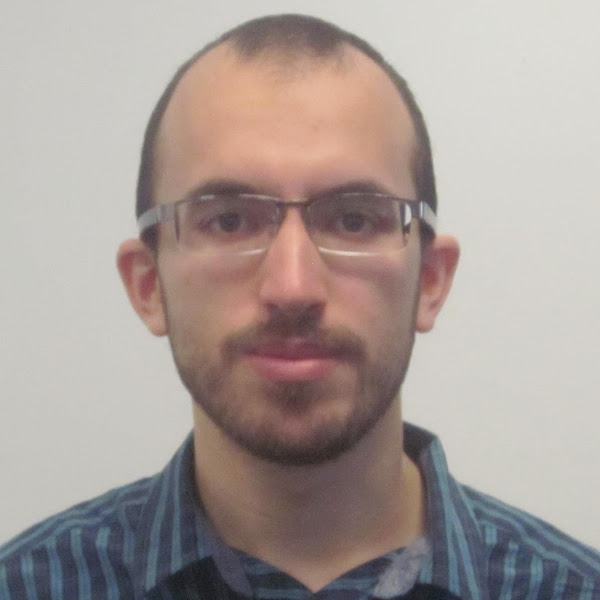
Victor Ungureanu
Research Areas
Authored Publications
Sort By
Real-time Speech Frequency Bandwidth Extension
Dominik Roblek
Marco Tagliasacchi
Oleg Rybakov
2021 IEEE International Conference on Acoustics, Speech and Signal Processing (to appear)
Preview abstract
In this paper we propose a lightweight model that performs frequency bandwidth extension of speech signals, increasing the sampling frequency from 8kHz to 16kHz, while restoring the high frequency content to a level that is indistinguishable from the original samples at 16kHz. The model architecture is based on SEANet (Sound EnhAncement Network), a wave-to-wave fully convolutional model, which adopts a combination of feature losses and adversarial losses to reconstruct an enhanced version of the input speech. In addition, we propose a version of SEANet that can be deployed on device in streaming mode, achieving an architecture latency of 16ms. When profiled on a single mobile CPU, processing one 16ms frame takes only 1.5ms, so that the total latency is compatible with a deployment in bi-directional voice communication systems.
View details
Preview abstract
Common text-to-speech (TTS) systems rely on training data
for modelling human speech. The quality of this data can
range from professional voice actors recording hand-curated
sentences in high-quality studio conditions, to found voice data
representing arbitrary domains. For years, the unit selection
technology dominant in the field required many hours of data
that was expensive and time-consuming to collect. With the advancement
of statistical methods of waveform generation, there
have been experiments with more noisy and often much larger
datasets (“big data”), testing the inherent flexibility of such systems.
In this paper we examine the relationship between training
data and speech synthesis quality. We then hypothesise that
statistical text-to-speech benefits from high acoustic quality corpora
with high level of prosodic variation, but that beyond the
first few hours of training data we don’t observe quality gains.
We then describe how we engineered a training dataset containing
optimized distribution of features, and how these features
were defined. Lastly, we present results from a series of
evaluation tests. These confirm our hypothesis and show how
a carefully engineered training corpus of a smaller size yields
the same speech quality as much larger datasets, particularly
for voices that use WaveNet.
View details