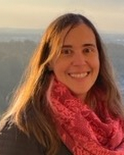
Tiffany Knearem
Tiffany is a User Experience Researcher with expertise in Human-Computer Interaction (HCI), currently conducting research in the area of front-end development (e.g., designer-developer collaboration, design handoff, AI-supported product development). She has wide-ranging interests in the areas of human-AI alignment, product design, creativity support tooling and participatory design.
She is active in HCI research communities, specifically ACM CHI, CSCW, C&C and UIST.
Tiffany holds a PhD in Information Sciences and Technologies from Pennsylvania State University, advised by Dr. John M. Carroll. Her PhD focused on community informatics; she developed a research program to understand and enable community innovation and peer-to-peer care through information and communication technologies.
For more information, please visit her Google Scholar.