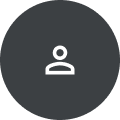
Steffen Rendle
Research Areas
Authored Publications
Sort By
Preview abstract
In recommendation systems, there has been a growth in the number of recommendable items (# of movies, music, products). When the set of recommendable items is large, training and evaluation of item recommendation models becomes computationally expensive. To lower this cost, it has become common to sample negative items. However, the recommendation quality can suffer from biases introduced by traditional negative sampling mechanisms. In this work, we demonstrate the benefits from correcting the bias introduced by sampling of negatives. We first provide sampled batch version of the well-studied WARP and LambdaRank methods. Then, we present how these methods can benefit from improved ranking estimates. Finally, we evaluate the recommendation quality as a result of correcting rank estimates and demonstrate that WARP and LambdaRank can be learned efficiently with negative sampling and our proposed correction technique.
View details
Revisiting the Performance of iALS on Item Recommendation Benchmarks
Walid Krichene
Li Zhang
Yehuda Koren
Proceedings of the 16th ACM Conference on Recommender Systems, Association for Computing Machinery (2022), pp. 427-435
Preview abstract
Matrix factorization learned by implicit alternating least squares (iALS) is a popular baseline in recommender system research publications. iALS is known to be one of the most computationally efficient and scalable collaborative filtering methods. However, recent studies suggest that its prediction quality is not competitive with the current state of the art, in particular autoencoders and other item-based collaborative filtering methods. In this work, we revisit four well-studied benchmarks where iALS was reported to perform poorly and show that with proper tuning, iALS is highly competitive and outperforms any method on at least half of the comparisons. We hope that these high quality results together with iALS's known scalability spark new interest in applying and further improving this decade old technique.
View details
Data Bootstrapping for Interactive Recommender Systems
Ajay Joshi
Ajit Apte
Anand Kesari
Anushya Subbiah
Dima Kuzmin
John Anderson
Li Zhang
Marty Zinkevich
The 2nd International Workshop on Online and Adaptive Recommender Systems (2022)
Preview abstract
Modifying recommender systems for new kinds of user interactions is costly and exploration is slow since machine learning models can be trained and evaluated on live data only after a product supporting these new interactions is deployed. Our data bootstrapping approach moves the task of developing models for new interactions into the input representation allowing a standard machine learning model (e.g. a transformer model) to be used to train a model capturing the new interactions. More specifically, we use data obtained from a launched system to generate simulated data that includes the new interactions options. This approach helps accelerate model and algorithm development, and reduce the time to launch new interaction experiences. We present machine learning methods designed specifically to work well with limited and noisy data produced via data bootstrapping.
View details
Private Alternating Least Squares: (Nearly) OptimalPrivacy/Utility Trade-off for Matrix Completion
Abhradeep Guha Thakurta
Li Zhang
Walid Krichene
NA, NA (2021), NA
Preview abstract
We study the problem of differentially private (DP) matrix completion under user-level privacy. We design an $(\epsilon,\delta)$-joint differentially private variant of the popular Alternating-Least-Squares (ALS) method that achieves: i) (nearly) optimal sample complexity for matrix completion (in terms of number of items, users), and ii) best known privacy/utility trade-off both theoretically, as well as on benchmark data sets.
In particular, despite non-convexity of low-rank matrix completion and ALS, we provide the first global convergence analysis of ALS with {\em noise} introduced to ensure DP. For $n$ being the number of users and $m$ being the number of items in the rating matrix, our analysis requires only about $\log (n+m)$ samples per user (ignoring rank, condition number factors) and obtains a sample complexity of $n=\tilde\Omega(m/(\sqrt{\zeta}\cdot \epsilon))$ to ensure relative Frobenius norm error of $\zeta$. This improves significantly on the previous state of the result of $n=\tilde\Omega\left(m^{5/4}/(\zeta^{5}\epsilon)\right)$ for the private-FW method by ~\citet{jain2018differentially}.
Furthermore, we extensively validate our method on synthetic and benchmark data sets (MovieLens 10mi, MovieLens 20mi), and observe that private ALS only suffers a 6 percentage drop in accuracy when compared to the non-private baseline for $\epsilon\leq 10$. Furthermore, compared to prior work of~\cite{jain2018differentially}, it is at least better by 10 percentage for all choice of the privacy parameters.
View details
Preview abstract
The task of item recommendation requires ranking a large catalogue of items given a context. Item recommendation algorithms are evaluated using ranking metrics that depend on the positions of relevant items. To speed up the computation of metrics, recent work often uses sampled metrics where only a smaller set of random items and the relevant items are ranked. This paper investigates sampled metrics in more detail and shows that they are inconsistent with their exact version, in the sense that they do not persist relative statements, e.g., recommender A is better than B, not even in expectation. Moreover, the smaller the sampling size, the less difference there is between metrics, and for very small sampling size, all metrics collapse to the AUC metric. We show that it is possible to improve the quality of the sampled metrics by applying a correction, obtained by minimizing different criteria such as bias or mean squared error. We conclude with an empirical evaluation of the naive sampled metrics and their corrected variants. To summarize, our work suggests that sampling should be avoided for metric calculation, however if an experimental study needs to sample, the proposed corrections can improve the quality of the estimate.
View details
Preview abstract
Embedding based models have been the state of the art in collaborative filtering for over a decade. Traditionally, the dot product or higher order equivalents have been used to combine two or more embeddings, e.g., most notably in matrix factorization. In recent years, it was suggested to replace the dot product with a learned similarity e.g. using a multilayer perceptron (MLP). This approach is often referred to as neural collaborative filtering (NCF). In this work, we revisit the experiments of the NCF paper that popularized learned similarities using MLPs. First, we show that with a proper hyperparameter selection, a simple dot product substantially outperforms the proposed learned similarities. Second, while a MLP can in theory approximate any function, we show that it is non-trivial to learn a dot product with an MLP. Finally, we discuss practical issues that arise when applying MLP based similarities and show that MLPs are too costly to use for item recommendation in production environments while dot products allow to apply very efficient retrieval algorithms. We conclude that MLPs should be used with care as embedding combiner and that dot products might be a better default choice.
View details
Zero-Shot Transfer Learning for Query-Item Cold Start in Search Retrieval and Recommendations
Ankit Kumar
Cosmo Du
Dima Kuzmin
Ellie Chio
John Roberts Anderson
Li Zhang
Nitin Jindal
Pei Cao
Ritesh Agarwal
Tao Wu
Wen Li
CIKM (2020)
Preview abstract
Most search retrieval and recommender systems predict top-K items given a query by learning directly from a large training set of (query, item) pairs, where a query can include natural language (NL), user, and context features. These approaches fall into the traditional supervised learning framework where the algorithm trains on labeled data from the target task. In this paper, we propose a new zero-shot transfer learning framework, which first learns representations of items and their NL features by predicting (item, item) correlation graphs as an auxiliary task, followed by transferring learned representations to solve the target task (query-to-item prediction), without having seen any (query, item) pairs in training. The advantages of applying this new framework include: (1) Cold-starting search and recommenders without abundant query-item data; (2) Generalizing to previously unseen or rare (query, item) pairs and alleviating the "rich get richer" problem; (3) Transferring knowledge of (item, item) correlation from domains outside of search. We show that the framework is effective on a large-scale search and recommender system.
View details
Preview abstract
We extend the idea of word pieces in natural language models to machine learning tasks on opaque ids. This is achieved by applying hash functions to map each id to multiple hash tokens in a much smaller space, similarly to a Bloom filter. We show that by applying a multi-layer Transformer to these Bloom filter digests, we are able to obtain models with high accuracy. They outperform models of a similar size without hashing and, to a large degree, models of a much larger size trained using sampled softmax with the same computational budget. Our key observation is that it is important to use a multi-layer Transformer for Bloom filter digests to remove ambiguity in the hashed input. We believe this provides an alternative method to solving problems with large vocabulary size.
View details
Preview abstract
Several machine learning models involve mapping a score vector to a probability vector. Usually, this is done by projecting the score vector onto a probability simplex, and such projections are often characterized as Lipschitz continuous approximations of the argmax function, whose Lipschitz constant is controlled by a parameter that is similar to a softmax temperature. The aforementioned parameter has been observed to affect the quality of these models and is typically either treated as a constant or decayed over time. In this work, we propose a method that adapts this parameter to individual training examples. The resulting method exhibits desirable properties, such as sparsity of its support and numerically efficient implementation, and we find that it significantly outperforms competing non-adaptive projection methods. In our analysis, we also derive the general solution of (Bregman) projections onto the (n, k)-simplex, a result which may be of independent interest.
View details
Efficient Training on Very Large Corpora via Gramian Estimation
Walid Krichene
Nicolas Mayoraz
Li Zhang
Lichan Hong
John Anderson
ICLR 2019 (to appear)
Preview abstract
We study the problem of learning similarity functions over very large corpora using neural network embedding models. These models are typically trained using SGD with random sampling of unobserved pairs, with a sample size that grows quadratically with the corpus size, making it expensive to scale.
We propose new efficient methods to train these models without having to sample unobserved pairs. Inspired by matrix factorization, our approach relies on adding a global quadratic penalty and expressing this term as the inner-product of two generalized Gramians. We show that the gradient of this term can be efficiently computed by maintaining estimates of the Gramians, and develop variance reduction schemes to improve the quality of the estimates. We conduct large-scale experiments that show a significant improvement both in training time and generalization performance compared to sampling methods.
View details