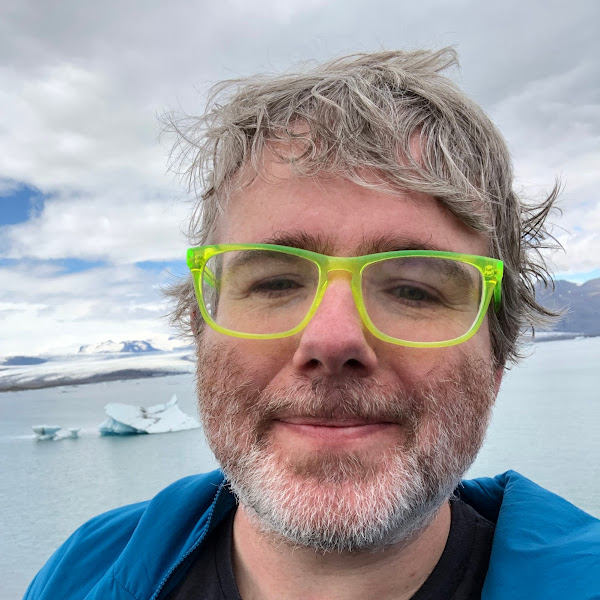
Shaun Kane
Research Areas
Authored Publications
Sort By
Google
From Provenance to Aberrations: Image Creator and Screen Reader User Perspectives on Alt Text for AI-Generated Images
Maitraye Das
Alexander J. Fiannaca
CHI Conference on Human Factors in Computing Systems (2024)
“They only care to show us the wheelchair”: disability representation in text-to-image AI models
Avery Mack
Rida Qadri
CHI Conference on Human-Computer Interaction (2024)
Using large language models to accelerate communication for eye gaze typing users with ALS
Subhashini Venugopalan
Katie Seaver
Xiang Xiao
Katrin Tomanek
Sri Jalasutram
Ajit Narayanan
Bob MacDonald
Emily Kornman
Daniel Vance
Blair Casey
Steve Gleason
(2024)
Designing Responsible AI: Adaptations of UX Practice to Meet Responsible AI Challenges
Qiaosi Wang
Michael Adam Madaio
Shivani Kapania
Lauren Wilcox
ACM Conference on Human Factors in Computing Systems (ACM CHI) 2023, ACM (2023)
SpeakFaster Observer: Long-Term Instrumentation of Eye-Gaze Typing for Measuring AAC Communication
Katrin Tomanek
Richard Jonathan Noel Cave
Bob MacDonald
Jon Campbell
Blair Casey
Emily Kornman
Daniel Vance
Jay Beavers
CHI23 Case Studies of HCI in Practice (2023) (to appear)
Practical Challenges for Investigating Abbreviation Strategies
Elisa Kreiss
CHI 2023 Workshop on Assistive Writing, ACM (2023) (to appear)
“The less I type, the better”: How AI Language Models can Enhance or Impede Communication for AAC Users
Stephanie Valencia
Richard Cave
Krystal Kallarackal
Katie Seaver
ACM Conference on Human Factors in Computing Systems (ACM CHI) 2023, ACM (2023) (to appear)
"I wouldn’t say offensive but...": Disability-Centered Perspectives on Large Language Models
Vinitha Gadiraju
Alex Taylor
Robin Brewer
Proceedings of FAccT 2023 (2023) (to appear)