People
Our researchers drive advancements in computer science through both fundamental and applied research.
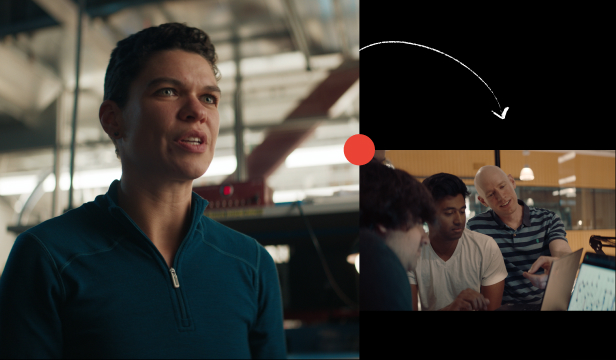
Our researchers drive advancements in computer science through both fundamental and applied research.
1 - 30 of 2267 researchers
-
Martín Abadi
- Algorithms and Theory
- Distributed Systems and Parallel Computing
- Machine Intelligence
-
Pendo Abbo
- Machine Learning
-
Ahmed Abdelkader
- Algorithms and Theory
- Machine Learning
-
Douglas Aberdeen
- Machine Intelligence
-
Jacob Abernethy
- Algorithms and Theory
- Economics and Electronic Commerce
- Machine Intelligence
-
Sami Abu-El-Haija
- Algorithms and Theory
- Machine Perception
-
Moheeb Abu Rajab
- Security, Privacy and Abuse Prevention
-
Mustafa Emre Acer
- Security, Privacy and Abuse Prevention
-
Hartwig Adam
- Data Mining and Modeling
- Machine Perception
-
Balu Adsumilli
- Algorithms and Theory
- Audio Processing
- Machine Intelligence
-
Mohit Agarwal
- Human-Computer Interaction and Visualization
- Machine Intelligence
- Machine Learning
-
Sameer Agarwal
- Algorithms and Theory
- Machine Perception
-
Alekh Agarwal
- Algorithms and Theory
- Machine Intelligence
-
Shashank Agarwal
- Algorithms and Theory
- Data Mining and Modeling
- Education Innovation
-
Anmol Agarwal
- Natural Language Processing
-
Gagan Aggarwal
- Algorithms and Theory
- Data Mining and Modeling
- Economics and Electronic Commerce
-
Ankur Agiwal
- Data Management
- Distributed Systems and Parallel Computing
-
Shreya Agrawal
- General Science
- Machine Intelligence
-
Priyanka Agrawal
- Machine Intelligence
- Natural Language Processing
-
Blaise Aguera y Arcas
- Human-Computer Interaction and Visualization
- Machine Intelligence
- Machine Perception
-
Eirikur Agustsson
- Machine Intelligence
- Machine Perception
-
Roee Aharoni
- Machine Translation
- Natural Language Processing
-
Sara Ahmadian
- Algorithms and Theory
- Data Mining and Modeling
- Distributed Systems and Parallel Computing
-
Zafarali Ahmed
- Machine Intelligence
-
Amr Ahmed
- Data Mining and Modeling
- Human-Computer Interaction and Visualization
- Information Retrieval and the Web
-
Faruk Ahmed
- Machine Learning
- Health & Bioscience
-
Mustaq Ahmed
- Algorithms and Theory
- Networking
-
Junwhan Ahn
- Distributed Systems and Parallel Computing
- Hardware and Architecture
- Software Systems
-
Dror Aiger
- Algorithms and Theory
- Information Retrieval and the Web
- Machine Perception
-
Alex Ainslie
- Human-Computer Interaction and Visualization
- Security, Privacy and Abuse Prevention