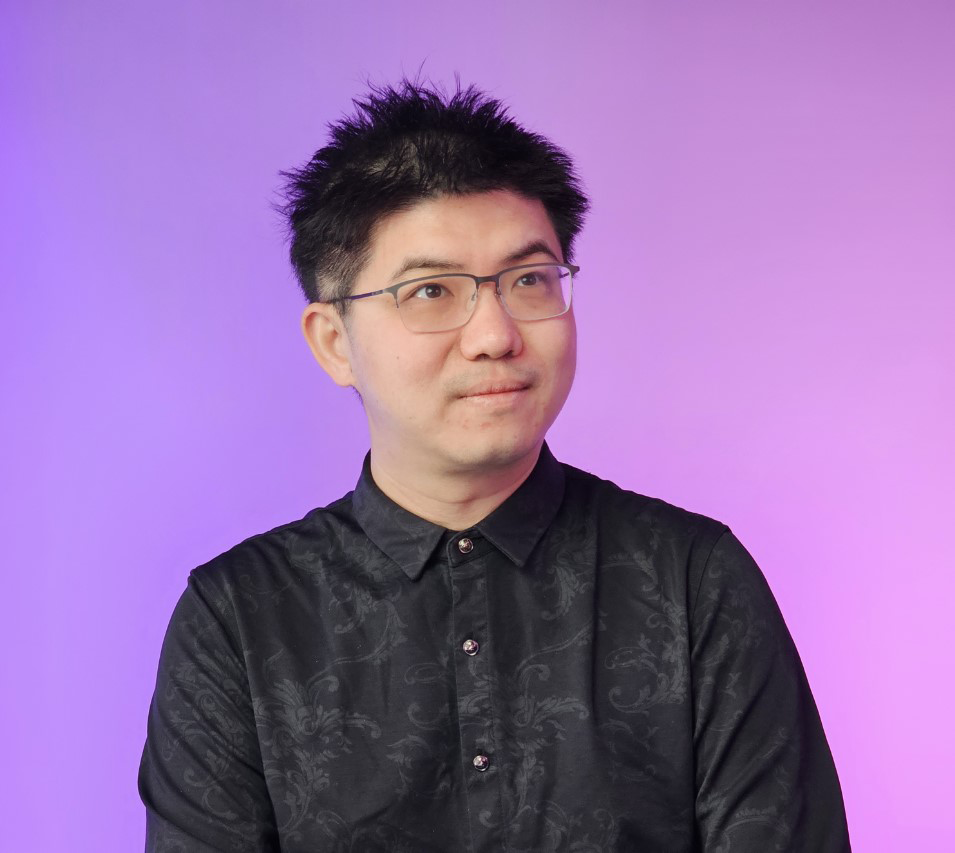
Ruofei Du
Ruofei Du serves as Interactive Perception & Graphics Lead / Manager at Google and devotes to creating novel interactive technologies for XR. As a Research Scientist, Ruofei's research covers a wide range of topics in technical HCI, Graphics, and Perception, including XR interactions, visual programming, augmented communication, XR social platforms, digital human, foveated rendering, accessibility, and deep learning in graphics. Du serves as an Associate Chair in program committee of CHI and UIST; an Associate Editor of IEEE TCSVT. He holds 3 US patents and has published over 30 peer-reviewed publications in top venues of HCI, Computer Graphics, and Computer Vision, including CHI, UIST, SIGGRAPH Asia, TVCG, CVPR, ICCV, ECCV, ISMAR, VR, and I3D. In his own words: I am passionate about inventing interactive technologies with graphics, perception, and HCI. See my research, artsy, projects, youtube, talks, github, and shadertoy demos for fun!
Personal Website
Google Scholar