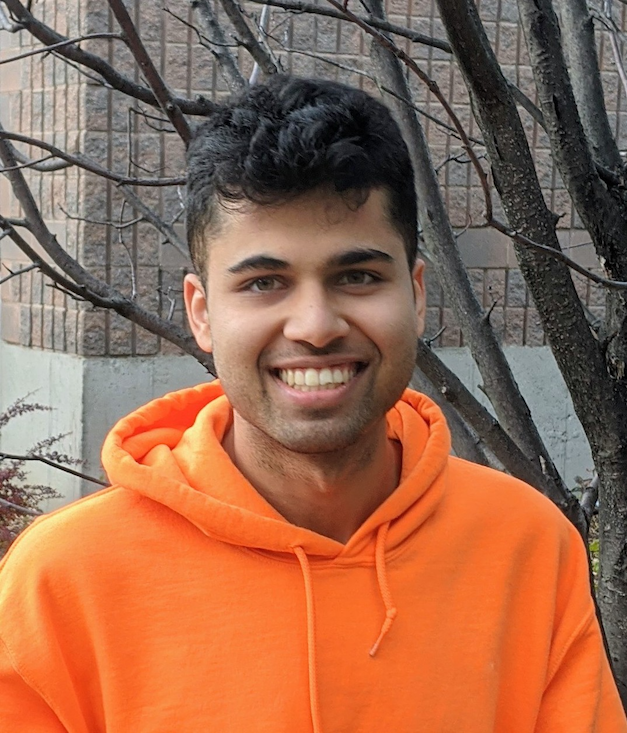
Rishabh Agarwal
I am a staff research scientist in the Google DeepMind team in Montréal. My research interests mainly revolve around Reinforcement Learning (RL), often with the goal of making RL methods suitable for real-world problems, and includes an outstanding paper award at NeurIPS. My personal website is at agarwl.github.io.
Research Areas
Authored Publications
Sort By
DistillSpec: Improving speculative decoding via knowledge distillation
Kaifeng Lyu
Aditya Menon
Yongchao Zhou
Jean-François Kagy
International Conference on Learning Representations (ICLR) (2024)
Preview abstract
Speculative decoding proves highly effective in expediting Large Language Model inference by employing a smaller draft model for token generation and a larger model for parallel token verification. Nonetheless, identifying an accurate and compact draft model aligned with the target model presents challenges. To address this, we propose leveraging white-box knowledge distillation, significantly improving draft model alignment with the larger target model, thereby enhancing speculative decoding. Our findings underscore the pivotal role of on-policy data generation and a suitable divergence function tailored to the task and decoding scheme for successful distillation. In practice, our refined distillation approach yields 20\% speedup over standard speculative decoding across five distinct tasks, using both greedy decoding and temperature sampling. Furthermore, we extend the concept of lossless speculative decoding to incorporate a lenience factor in the rejection sampling step, offering fine-grained control over the trade-off between quality and latency in lossy decoding. Finally, adopting a strategy of "distilling for performance first and distillation for speculative decoding second" enables a remarkable 8x reduction in latency with minimal performance compromise, compared to no distillation and speculative decoding baseline.
View details
Bigger, Better, Faster: Human-level Atari with human-level efficiency
Aaron Courville
Marc Bellemare
Johan Obando Ceron
Max Schwarzer
ICML (2023)
Preview abstract
We introduce a value-based RL agent, which we call BBF, that achieves super-human performance in the Atari 100K benchmark. BBF relies on scaling the neural networks used for value estimation, as well as a number of other design choices that enable this scaling in a sample-efficient manner. We conduct extensive analyses of these design choices and provide insights for future work. We end with a discussion about moving the goalpost for sample-efficient RL research on the ALE.
View details
Preview abstract
Offline reinforcement learning (RL) on large, heterogeneous datasets with highcapacity models can, in principle, lead to agents that generalize broadly, analogously to similar advances in vision and NLP. However, recent works argue
that offline RL methods encounter unique challenges to scaling up model capacity. Drawing on the learnings from these works, we re-examine previous design choices and find that with appropriate choices: wider ResNets, cross-entropy
based distributional backups, and feature normalization, offline Q-learning algorithms exhibit strong performance that scales with model capacity. Using multitask Atari as a test-bed for scaling and generalization, we train a single policy on
40 games with near-human performance using up-to 100M parameter networks,
finding that model performance scales favorably with capacity. In contrast to prior
work, we substantially extrapolate beyond dataset performance even when trained
entirely on a large (400M transitions) but highly suboptimal dataset (51% humannormalized score). Compared to supervised approaches, offline RL scales similarly with model capacity and has better performance, especially when the dataset
is suboptimal. Finally, we show that such offline Q-functions learn powerful representations that facilitate rapid transfer to novel games and fast online learning on
new variations of a training game, improving over existing representation learning
approaches.
View details
Proto-Value Networks: Scaling Representation Learning with Auxiliary Tasks
Marc Bellemare
Jesse Farebrother
Joshua Greaves
Charline Le Lan
International Conference on Learning Representations (ICLR) (2023)
Preview abstract
Auxiliary tasks improve the representations learned by deep reinforcement learning agents. Analytically, their effect is reasonably well-understood; in practice, how-ever, their primary use remains in support of a main learning objective, rather than as a method for learning representations. This is perhaps surprising given that many auxiliary tasks are defined procedurally, and hence can be treated as an essentially infinite source of information about the environment. Based on this observation, we study the effectiveness of auxiliary tasks for learning rich representations, focusing on the setting where the number of tasks and the size of the agent’s network are simultaneously increased. For this purpose, we derive a new family of auxiliary tasks based on the successor measure. These tasks are easy to implement and have appealing theoretical properties. Combined with a suitable off-policy learning rule, the result is a representation learning algorithm that can be understood as extending Mahadevan & Maggioni (2007)’s proto-value functions to deep reinforcement learning – accordingly, we call the resulting object proto-value networks. Through a series of experiments on the Arcade Learning Environment, we demonstrate that proto-value networks produce rich features that may be used to obtain performance comparable to established algorithms, using only linear approximation and a small number (~4M) of interactions with the environment’s reward function.
View details
Preview abstract
Offline model selection (OMS), that is, choosing the best policy from a set of many policies given only logged data, is crucial for applying offline RL in real-world settings. One idea that has been extensively explored is to select Q-functions based on their mean squared Bellman error (MSBE). However, previous work has struggled to obtain adequate OMS performance with Bellman errors, leading many researchers to abandon the idea. Through theoretical and empirical analyses, we elucidate why previous work has seen pessimistic results with Bellman errors and identify conditions under which OMS algorithms based on Bellman errors will perform well. Moreover, we develop a new OMS algorithm based on the MSBE that is more accurate than prior methods and obtains impressive performance on diverse discrete control tasks, including Atari games. We open-source our data and code to enable researchers to conduct OMS experiments more easily.
View details
Reincarnating Reinforcement Learning: Reusing Prior Computation to Accelerate Progress
Aaron Courville
Marc G. Bellemare
Max Allen Schwarzer
NeurIPS (2022)
Preview abstract
Learning tabula rasa, that is without any prior knowledge, is the prevalent workflow in reinforcement learning (RL) research. However, RL systems, when applied to large-scale settings, rarely operate tabula rasa. Such large-scale systems undergo multiple design or algorithmic changes during their development cycle and use ad hoc approaches for incorporating these changes without re-training from scratch, which would have been prohibitively expensive. Additionally, the inefficiency of deep RL typically excludes researchers without access to industrial-scale resources from tackling computationally-demanding problems. To address these issues, we present reincarnating RL as an alternative workflow or class of problem settings, where prior computational work (e.g., learned policies) is reused or transferred between design iterations of an RL agent, or from one RL agent to another. As a step towards enabling reincarnating RL from any agent to any other agent, we focus on the specific setting of efficiently transferring an existing sub-optimal policy to a standalone value-based RL agent. We find that existing approaches fail in this setting and propose a simple algorithm to address their limitations. Equipped with this algorithm, we demonstrate reincarnating RL's gains over tabula rasa RL on Atari 2600 games, a challenging locomotion task, and the real-world problem of navigating stratospheric balloons. Overall, this work argues for an alternative approach to RL research, which we believe could significantly improve real-world RL adoption and help democratize it further. Open-sourced code and trained agents at
View details
Preview abstract
In reinforcement learning, state representations are used to tractably deal with large problem spaces. State representations serve both to approximate the value function with few parameters, but also to generalize to newly encountered states. Their features may be learned implicitly (as part of a neural network) or explicitly (for example, the successor representation of Dayan (1993)). While the approximation properties of representations are reasonably well-understood, a precise characterization of how and when these representations generalize is lacking. In this work, we address this gap and provide an informative bound on the generalization error arising from a specific state representation. This bound is based on the notion of effective dimension which measures the degree to which knowing the value at one state informs the value at other states. Our bound applies to any state representation and quantifies the natural tension between representations that generalize well and those that approximate well. We complement our theoretical results with an empirical survey of classic representation learning methods from the literature and results on the Arcade Learning Environment, and find that the generalization behaviour of learned representations is well-explained by their effective dimension.
View details
Preview abstract
Despite overparameterization, deep networks trained via supervised learning are easy to optimize and exhibit excellent generalization. One hypothesis to explain this is that overparameterized deep networks enjoy the benefits of implicit regularization induced by stochastic gradient descent, which favors parsimonious solutions that generalize well on test inputs. It is reasonable to surmise that deep reinforcement learning (RL) methods could also benefit from this effect. In this paper, we discuss how the implicit regularization effect of SGD seen in supervised learning could in fact be harmful in the offline deep RL setting, leading to poor generalization and degenerate feature representations. Our theoretical analysis shows that when existing models of implicit regularization are applied to temporal difference learning, the resulting derived regularizer favors degenerate solutions with excessive "aliasing", in stark contrast to the supervised learning case. We back up these findings empirically, showing that feature representations learned by a deep network value function trained via bootstrapping can indeed become degenerate, aliasing the representations for state-action pairs that appear on either side of the Bellman backup. To address this issue, we derive the form of this implicit regularizer and, inspired by this derivation, propose a simple and effective explicit regularizer, called DR3, that counteracts the undesirable effects of this implicit regularizer. When combined with existing offline RL methods, DR3 substantially improves performance and stability, alleviating unlearning in Atari 2600 games, D4RL domains and robotic manipulation from images.
View details
Deep Reinforcement Learning at the Edge of the Statistical Precipice
Aaron Courville
Marc G. Bellemare
Max Schwarzer
Advances in Neural Information Processing Systems (2021)
Preview abstract
Deep reinforcement learning (RL) algorithms are predominantly evaluated by comparing their relative performance on a large suite of tasks. Most published results on deep RL benchmarks compare point estimates of aggregate performance such as mean and median scores across tasks, ignoring the statistical uncertainty implied by the use of a finite number of training runs. Beginning with the Arcade Learning Environment (ALE), the shift towards computationally-demanding benchmarks has led to the practice of evaluating only a small number of runs per task, exacerbating the statistical uncertainty in point estimates. In this paper, we argue that reliable evaluation in the few run deep RL regime cannot ignore the uncertainty in results without running the risk of slowing down progress in the field. We illustrate this point using a case study on the Atari 100k benchmark, where we find substantial discrepancies between conclusions drawn from point estimates alone versus a more thorough statistical analysis. With the aim of increasing the field's confidence in reported results with a handful of runs, we advocate for reporting interval estimates of aggregate performance and propose performance profiles to account for the variability in results, as well as present more robust and efficient aggregate metrics, such as interquartile mean scores, to achieve small uncertainty in results. Using such statistical tools, we scrutinize performance evaluations of existing algorithms on other widely used RL benchmarks including the ALE, Procgen, and the DeepMind Control Suite, again revealing discrepancies in prior comparisons. Our findings call for a change in how we evaluate performance in deep RL, for which we present a more rigorous evaluation methodology, accompanied with an open-source library rliable, to prevent unreliable results from stagnating the field. See agarwl.github.io/rliable for more details.
View details
Implicit Under-Parameterization Inhibits Data-Efficient Deep Reinforcement Learning
Dibya Ghosh
Sergey Levine
Aviral Kumar*
International Conference on Learning Representations (2021)
Preview abstract
We identify an implicit under-parameterization phenomenon in value-based deep RL methods that use bootstrapping: when value functions, approximated using deep neural networks, are trained with gradient descent using iterated regression onto target values generated by previous instances of the value network, more gradient updates decrease the expressivity of the current value network. We characterize this loss of expressivity in terms of a drop in the rank of the learned value network features, and show that this corresponds to a drop in performance. We demonstrate this phenomenon on widely studies domains, including Atari and Gym benchmarks, in both offline and online RL settings. We formally analyze this phenomenon and show that it results from a pathological interaction between bootstrapping and gradient-based optimization. We further show that mitigating implicit under-parameterization by controlling rank collapse improves performance. More details can be found at agarwl.github.io/iup .
View details