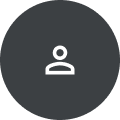
Rachel Fan
Research Areas
Authored Publications
Sort By
Google
Methods for Measuring Brand Lift of Online Ads
Tim Hesterberg
Ying Liu
Lu Zhang
Proceedings of the 2018 Joint Statistical Meetings, American Statistical Association
Brand Attitudes and Search Engine Queries
Jeffrey P. Dotson
Elea McDonnell Feit
Yi-Hsin Yeh
Journal of Interactive Marketing, 37 (2017), pp. 105-116 (to appear)