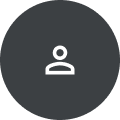
Pierre-Antoine Manzagol
I'm a Software Developer at Google Research, Brain Team in Montreal. I'm currently interested in machine learning methods for understanding and generating programs.
Research Areas
Authored Publications
Sort By
Resolving Code Review Comments with Machine Learning
Alexander Frömmgen
Elena Khrapko
Marcus Revaj
Peter Choy
2024 IEEE/ACM 46th International Conference on Software Engineering: Software Engineering in Practice (ICSE-SEIP) (to appear)
Preview abstract
Code reviews are a critical part of the software development process, taking a significant amount of the code authors’ and the code reviewers’ time. As part of this process, the reviewer inspects the proposed code and asks the author for code changes through comments written in natural language. At Google, we see millions of reviewer comments per year, and authors require an average of ∼60 minutes active shepherding time between sending changes for review and finally submitting the change. In our measurements, the required active work time that the code author must devote to address reviewer comments grows almost linearly with the number of comments. However, with machine learning (ML), we have an opportunity to automate and streamline the code-review process, e.g., by proposing code changes based on a comment’s text.
We describe our application of recent advances in large sequence models in a real-world setting to automatically resolve code-review comments in the day-to-day development workflow at Google. We present the evolution of this feature from an asynchronous generation of suggested edits after the reviewer sends feedback, to an interactive experience that suggests code edits to the reviewer at review time. In deployment, code-change authors at Google address 7.5% of all reviewer comments by applying an ML-suggested edit. The impact of this will be to reduce the time spent on code reviews by hundreds of thousands of engineer hours annually at Google scale. Unsolicited, very positive feedback highlights that the impact of ML-suggested code edits increases Googlers’ productivity and allows them to focus on more creative and complex tasks.
View details
PLUR: A Unifying, Graph-Based View of Program Learning, Understanding, and Repair
Zimin Chen
Vincent J Hellendoorn
Subhodeep Moitra
Thirty-fifth Conference on Neural Information Processing Systems (NeurIPS 2021) (2021)
Preview abstract
Machine learning for understanding and editing source code has recently attracted significant interest, with many developments in new models,
new code representations, and new tasks.
This proliferation can appear disparate and disconnected, making each approach seemingly unique and incompatible, thus obscuring the core
machine learning challenges and contributions.
In this work, we demonstrate that the landscape can be significantly simplified by taking a general approach of mapping a graph to a sequence of tokens and pointers.
Our main result is to show that 16 recently published tasks of different shapes can be cast in this form, based on which a single model architecture achieves near or above state-of-the-art results on nearly all tasks, outperforming custom models like code2seq and alternative generic models like Transformers.
This unification further enables multi-task learning and a series of cross-cutting experiments about the importance of different modeling choices for code understanding and repair tasks.
The full framework, called PLUR, is easily extensible to more tasks, and will be open-sourced (link).
View details
Meta-Dataset: A Dataset of Datasets for Learning to Learn from Few Examples
Eleni Triantafillou
Tyler Zhu
Carles Gelada
Kelvin Xu
International Conference on Learning Representations (submission) (2020)
Preview abstract
Few-shot classification refers to learning a classifier for new classes given only a few examples. While a plethora of models have emerged to tackle this recently, we find the current procedure and datasets that are used to systematically assess progress in this setting lacking. To address this, we propose META-DATASET: a new benchmark for training and evaluating few-shot classifiers that is large-scale, consists of multiple datasets, and presents more natural and realistic tasks. The aim is to measure the ability of state-of the-art models to leverage diverse sources of data to achieve higher generalization, and to evaluate that generalization ability in a more challenging setting. We additionally measure robustness of current methods to variations in the number of available examples and the number of classes. Finally our extensive empirical evaluation leads us to identify weaknesses in Prototypical Networks and MAML, two popular few-shot classification methods, and to propose a new method, ProtoMAML, which achieves improved performance on our benchmark.
View details
On the interplay between noise and curvature and its effect on optimization and generalization
Yoshua Bengio
Valentin Thomas
Nicolas Le Roux
Bart van Merriënboer
Proceedings of the 23rdInternational Conference on Artificial Intelligence and Statistics (AISTATS) (2020)
Preview abstract
This work revisits the notion of \textit{information criterion} to characterize generalization for modern deep learning models. In particular, we empirically demonstrate the effectiveness of the Takeuchi Information Criterion, an extension of the Akaike Information Criterion for misspecified models, in estimating the generalization gap, shedding light on why quantities such as the number of parameters cannot quantify generalization. The TIC depends on both the Hessian of the loss $\rmH$ and the covariance matrix of the gradients $\rmSS$. By exploring the semantic and numerical similarities and differences between these two matrices as well as the Fisher information matrix $\rmF$, we bring further evidence that flatness cannot in itself predict generalization. We also address the question of when is $\rmSS$ a reasonable approximation to $\rmF$, as commonly assumed.
View details
Reducing the variance in online optimization by transporting past gradients
Sebastien Arnold
Reza Babanezhad
Ioannis Mitliagkas
Nicolas Le Roux
NeurIPS 2019 (2019) (to appear)
Preview abstract
Most stochastic optimization methods use gradients once before discarding them. While variance reduction methods have shown that reusing past gradients can be beneficial when there is a finite number of datapoints, they do not easily extend to the online setting. One issue is the staleness due to using past gradients. We propose to correct this staleness using the idea of {\em implicit gradient transport} (IGT) which transforms gradients computed at previous iterates into gradients evaluated at the current iterate without using the Hessian explicitly. In addition to reducing the variance and bias of our updates over time, IGT can be used as a drop-in replacement for the gradient estimate in a number of well-understood methods such as heavy ball or Adam. We show experimentally that it achieves state-of-the-art results on a wide range of architectures and benchmarks. Additionally, the IGT gradient estimator yields the optimal asymptotic convergence rate for online stochastic optimization in the restricted setting where the Hessians of all component functions are equal.
View details