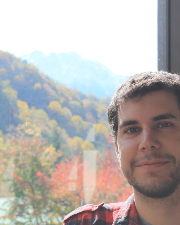
Philippe Hamel
Research Areas
Authored Publications
Sort By
Google
To Have a Tiger by the Tail: Improving Music Recommendation for International Users
Preview
Machine Learning for Music Discovery Workshop, ICML 2015
Transfer Learning In MIR: Sharing Learned Latent Representations For Music Audio Classification And Similarity
Matthew E. P. Davies
Kazuyoshi Yoshii
Masataka Goto
14th International Conference on Music Information Retrieval (ISMIR '13) (2013)
Building Musically-relevant Audio Features through Multiple Timescale Representations
Preview
Yoshua Bengio
Proceedings of the 13th International Society for Music Information Retrieval Conference, Porto, Portugal (2012)
Temporal pooling and multiscale learning for automatic annotation and ranking of music audio
Preview
Simon Lemieux
Yoshua Bengio
International Society for Music Information Retrieval (ISMIR 2011)