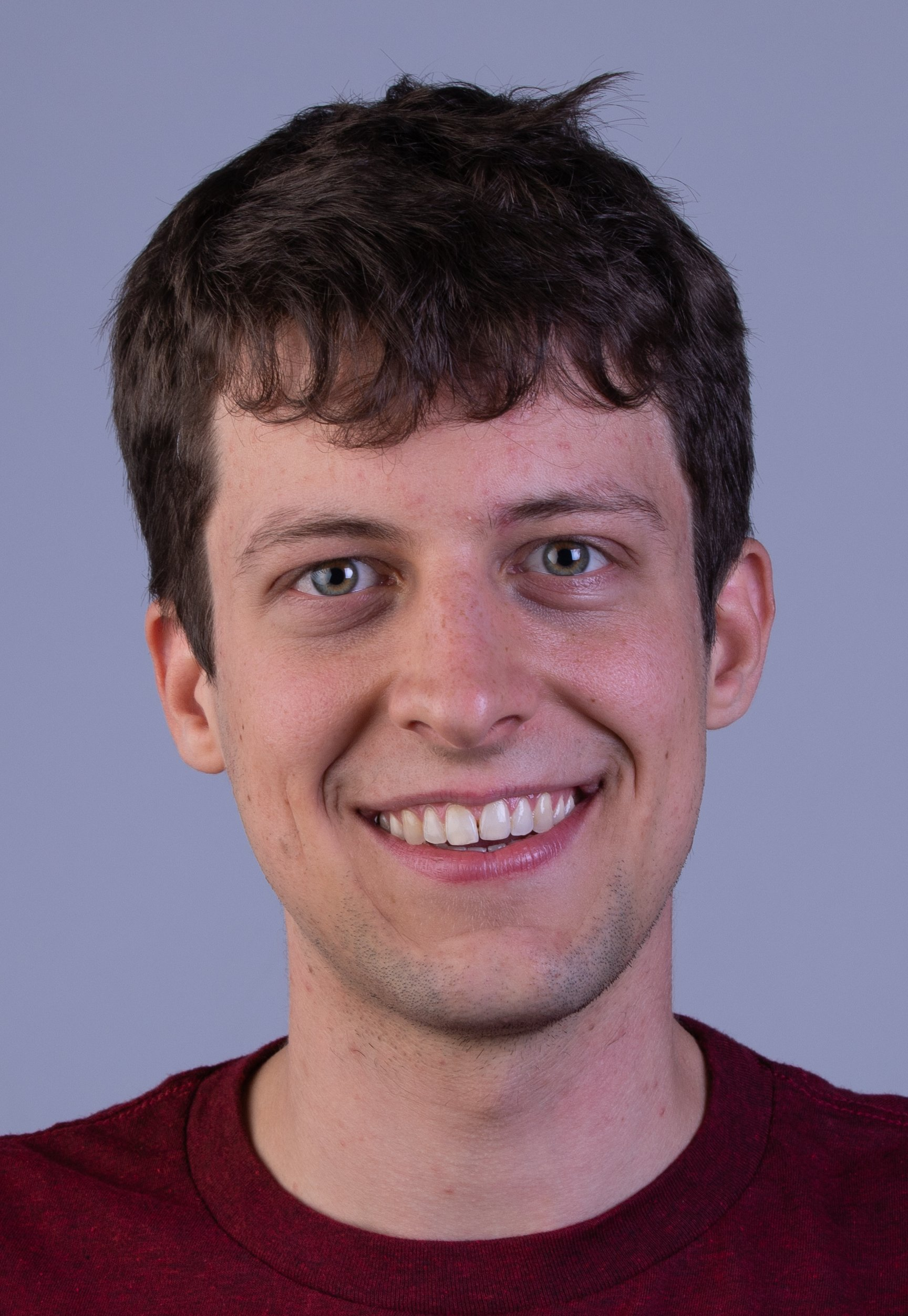
Paul Voigtlaender
I'm a research scientist at Google in Zurich, Switzerland. I'm currently working on the combination of language and vision for videos. Before that I did my PhD on "Video Object Segmentation and Tracking" at RWTH Aachen University, Germany.
Research Areas
Authored Publications
Sort By
Google
Connecting Vision and Language with Video Localized Narratives
Vittorio Ferrari
IEEE / CVF Computer Vision and Pattern Recognition Conference (CVPR) 2023 (to appear)
FEELVOS: Fast End-to-End Embedding Learning for Video Object Segmentation
Yuning Chai
Bastian Leibe
Liang-chieh Chen
International Conference on Computer Vision and Pattern Recognition (CVPR) (2019) (to appear)