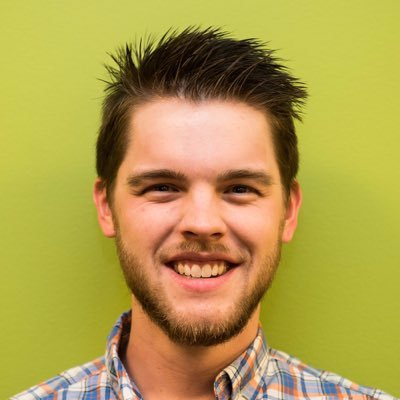
Mike Dusenberry
Mike Dusenberry is a Research Software Engineer at Google Cloud AI Research. Previously, he was AI Resident at Google Brain and Google Health Research. Before joining Google, Mike obtained a B.S. in Computer Science, studied in medical school as an M.D. candidate for two years prior to leaving to focus on machine learning, and worked as a machine learning engineer at IBM focused on research and open-source software for distributed systems and healthcare. Mike's work is focused on research in Bayesian deep learning (neural nets, probability, decisions, uncertainty), and applications to medicine and other high-stakes problems where uncertainty, reliability, and robustness matter.
Research Areas
Authored Publications
Sort By
Preview abstract
Accurate estimation of output quantiles is crucial in many use cases, where it is desired to model the range of possibility. Modeling target distribution at arbitrary quantile levels and at arbitrary input attribute levels are important to offer a comprehensive picture of the data, and requires the quantile function to be expressive enough. The quantile function describing the target distribution using quantile levels is critical for quantile regression. Althought various parametric forms for the distributions (that the quantile function specifies) can be adopted, an everlasting problem is selecting the most appropriate one that can properly approximate the data distributions. In this paper, we propose a non-parametric and data-driven approach, Neural Spline Search (NSS), to represent the observed data distribution without parametric assumptions. NSS is flexible and expressive for modeling data distributions by transforming the inputs with a series of monotonic spline regressions guided by symbolic operators. We demonstrate that NSS outperforms previous methods on synthetic, real-world regression and time-series forecasting tasks.
View details
Morse Neural Networks for Uncertainty Quantification
Clara Huiyi Hu
ICML 2023 Workshop on Structured Probabilistic Inference & Generative Modeling (2023)
Preview abstract
We introduce a new deep generative model useful for uncertainty quantification: the Morse neural network, which generalizes the unnormalized Gaussian densities to have modes of high-dimensional submanifolds instead of just discrete points. Fitting the Morse neural network via a KL-divergence loss yields 1) a (unnormalized) generative density, 2) an OOD detector, 3) a calibration temperature, 4) a generative sampler, along with in the supervised case 6) a distance aware-classifier. The Morse network can be used on top of a pre-trained network to bring distance-aware calibration w.r.t the training data. Because of its versatility, the Morse neural networks unifies many techniques: e.g., the Entropic Out-of-Distribution Detector of (Macêdo et al., 2021) inOOD detection, the one class Deep Support Vector Description method of (Ruff et al., 2018) in anomaly detection, or the Contrastive One Class classifier in continuous learning (Sun et al., 2021).The Morse neural network has connections to sup-port vector machines, kernel methods, and Morse theory in topology.
View details
Plex: Towards Reliability using Pretrained Large Model Extensions
Yarin Gal
Tim G. J. Rudner
Joost van Amersfoort
Neil Band
Honglin Yuan
Zelda Mariet
D. Sculley
Karan Singhal
Zi Wang
Kelly Buchanan
Mark Patrick Collier
Du Phan
Clara Huiyi Hu
Andreas Christian Kirsch
Rodolphe Jenatton
ICML 2022 Pre-training Workshop (2022)
Preview abstract
A recent trend in artificial intelligence (AI) is the use of pretrained models for language and vision tasks, which has achieved extraordinary performance but also puzzling failures. Examining tasks that probe the model’s abilities in diverse ways is therefore critical to the field. In this paper, we explore the \emph{reliability} of models, where we define a reliable model as one that not only achieves strong predictive performance but also performs well consistently over many decision-making tasks such as uncertainty (e.g., selective prediction, open set recognition), robust generalization (e.g., accuracy and scoring rules such as log-likelihood on in- and out-of-distribution datasets), and adaptation (e.g., active learning, few-shot learning). We devise 11 types of tasks over 36 datasets in order to evaluate different aspects of reliability on both vision and language domains. To improve reliability, we developed ViT-Plex and T5-Plex, \emph{p}retrained \emph{l}arge-model \emph{ex}tensions (henceforth abbreviated as \emph{plex}) for vision and language modalities. Plex greatly improves the state-of-the-art across tasks, and as a pretrained model Plex unifies the traditional protocol of designing and tuning one model for each reliability task. We demonstrate scaling effects over model sizes and pretraining dataset sizes up to 4 billion examples. We also demonstrate Plex’s capabilities on new tasks including zero-shot open set recognition, few-shot uncertainty, and uncertainty in conversational language understanding.
View details
Combining Ensembles and Data Augmentation Can Harm Your Calibration
Rafael Rios Müller
Yeming Wen
Ghassen Jerfel
International Conference on Learning Representations (2021)
Preview abstract
Ensemble methods which average over multiple neural network predictions are a simple approach to improve a model’s calibration and robustness. Similarly, data augmentation techniques, which encode prior information in the form of invariant feature transformations, are effective for improving calibration and robustness. In this paper, we show a surprising pathology: combining ensembles and data augmentation can harm model calibration. This leads to a trade-off in practice, whereby improved accuracy by combining the two techniques comes at the expense of calibration. On the other hand, selecting only one of the techniques ensures good uncertainty estimates at the expense of accuracy. We investigate this pathology and identify a compounding under-confidence among methods which marginalize over sets of weights and data augmentation techniques which soften labels. Finally, we propose a simple correction, achieving the best of both worlds with significant accuracy and calibration gains over using only ensembles or data augmentation individually. Applying the correction produces new state-of-the art in uncertainty calibration and robustness across CIFAR-10, CIFAR-100, and ImageNet.
View details
A prospective evaluation of AI-augmented epidemiology to forecast COVID-19 in the USA and Japan
Daisuke Yoneoka
Shashank Singh
Thomas Tsai
Shuhei Nomura
Hiroaki Miyata
Joel Shor
Ashwin Sura Ravi
Chun-Liang Li
Nate Yoder
Kris Popendorf
Dario Sava
Arkady Epshteyn
Beth Luan
Hiroki Kayama
Joe Mckenna
Johan Euphrosine
Isaac Jones
npj Digital Medicine (2021)
Preview abstract
The COVID-19 pandemic has highlighted the global need for reliable models of disease spread. We evaluate an AI-improved forecasting approach that provides daily predictions of the expected number of confirmed COVID-19 deaths, cases and hospitalizations during the following 28 days. We present an international, prospective evaluation of model performance across all states and counties in the USA and prefectures in Japan. National mean absolute percentage error (MAPE) for predicting COVID-19 associated deaths before and after prospective deployment remained consistently <3% (US) and <10% (Japan). Average statewide (US) and prefecture wide (Japan) MAPE was 6% and 20% respectively (14% when looking at prefectures with more than 10 deaths).We show our model performs well even during periods of considerable change in population behavior, and that it is robust to demographic differences across different geographic locations.We further demonstrate the model provides meaningful explanatory insights, finding that the model appropriately responds to local and national policy interventions. Our model enables counterfactual simulations, which indicate continuing NPIs alongside vaccinations is essential for more rapidly recovering from the pandemic, delaying the application of interventions has a detrimental effect, and allow exploration of the consequences of different vaccination strategies. The COVID-19 pandemic remains a global emergency. In the face of substantial challenges ahead, the approach presented here has the potential to inform critical decisions.
View details
Analyzing the Role of Model Uncertainty for Electronic Health Records
Jeremy Nixon
Edward Choi
Ghassen Jerfel
ACM Conference on Health, Inference, and Learning (ACM CHIL) (2020)
Preview abstract
In medicine, both ethical and monetary costs of incorrect predictions can be significant, and the complexity of the problems often necessitates increasingly complex models. Recent work has shown that changing just the random seed is enough for otherwise well-tuned deep neural networks to vary in their individual predicted probabilities. In light of this, we investigate the role of model uncertainty methods in the medical domain. Using RNN ensembles and various Bayesian RNNs, we show that population-level metrics, such as AUC-PR, AUC-ROC, log-likelihood, and calibration error, do not capture model uncertainty. Meanwhile, the presence of significant variability in patient-specific predictions and optimal decisions motivates the need for capturing model uncertainty. Understanding the uncertainty for individual patients is an area with clear clinical impact, such as determining when a model decision is likely to be brittle. We further show that RNNs with only Bayesian embeddings can be a more efficient way to capture model uncertainty compared to ensembles, and we analyze how model uncertainty is impacted across individual input features and patient subgroups.
View details
Learning the Graphical Structure of Electronic Health Records with Graph Convolutional Transformer
Gerardo Flores
Zhen Xu
Edward Choi
Yujia Li
Association for the Advancement of Artificial Intelligence (AAAI) (2020)
Preview abstract
Effective modeling of electronic health records (EHR) is rapidly becoming an important topic in both academia and industry. A recent study showed that using the graphical structure underlying EHR data (e.g. relationship between diagnoses and treatments) improves the performance of prediction tasks such as heart failure prediction. However, EHR data do not always contain complete structure information. Moreover, when it comes to claims data, structure information is completely unavailable to begin with. Under such circumstances, can we still do better than just treating EHR data as a flat-structured bag-of-features? In this paper, we study the possibility of jointly learning the hidden structure of EHR while performing supervised prediction tasks on EHR data. Specifically, we discuss that Transformer is a suitable basis model to learn the hidden EHR structure, and propose Graph Convolutional Transformer, which uses data statistics to guide the structure learning process. The proposed model consistently outperformed previous approaches empirically, on both synthetic data and publicly available EHR data, for various prediction tasks such as graph reconstruction and readmission prediction, indicating that it can serve as an effective general-purpose representation learning algorithm for EHR data.
View details
Efficient and Scalable Bayesian Neural Nets with Rank-1 Factors
Yeming Wen
Ghassen Jerfel
Yian Ma
International Conference on Machine Learning (ICML) (2020)
Preview abstract
Bayesian neural networks (BNNs) demonstrate promising success in improving the robustness and uncertainty quantification of modern neural networks. However, they generally struggle with underfitting at scale and parameter efficiency. On the other hand, deep ensembles have emerged as an alternative for uncertainty quantification that, while outperforming BNNs on certain problems, also suffers from efficiency issues. It remains unclear how to combine the strengths of these two approaches and remediate their common issues. To tackle this challenge, we propose a rank-1 parameterization of BNNs, where each weight matrix involves only a distribution on a rank-1 subspace. We also revisit the use of mixture approximate posteriors to capture multiple modes where unlike typical mixtures, this approach admits a significantly smaller memory increase (e.g., only a 0.4% increase for a ResNet-50 mixture of size 10). We perform a systematic empirical study on the choices of prior, variational posterior, and methods to improve training. For ResNet-50 on ImageNet and Wide ResNet 28-10 on CIFAR-10/100, rank-1 BNNs outperform baselines across log-likelihood, accuracy, and calibration on the test set and out-of-distribution variants.
View details
Bayesian Layers: A Module for Neural Network Uncertainty
Mark van der Wilk
Danijar Hafner
Neural Information Processing Systems (NeurIPS) (2019)
Preview abstract
We describe Bayesian Layers, a module designed for fast experimentation with neural network uncertainty. It extends neural network libraries with drop-in replacements for common layers. This enables composition via a unified abstraction over deterministic and stochastic functions and allows for scalability via the underlying system. These layers capture uncertainty over weights (Bayesian neural nets), pre-activation units (dropout), activations ("stochastic output layers"), or the function itself (Gaussian processes). They can also be reversible to propagate uncertainty from input to output. We include code examples for common architectures such as Bayesian LSTMs, deep GPs, and flow-based models. As demonstration, we fit a 5-billion parameter "Bayesian Transformer" on 512 TPUv2 cores for uncertainty in machine translation and a Bayesian dynamics model for model-based planning. Finally, we show how Bayesian Layers can be used within the Edward2 probabilistic programming language for probabilistic programs with stochastic processes.
View details