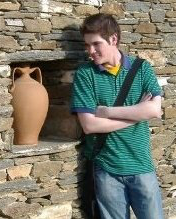
Michael Tseng
Authored Publications
Sort By
A Chain-of-Thought Is as Strong as Its Weakest Link: A Benchmark for Verifiers of Reasoning Chains
Alon Jacovi
Or Honovich
Proceedings of the 62nd Annual Meeting of the Association for Computational Linguistics (2024), pp. 4615–4634
Preview abstract
Prompting language models to provide step-by-step answers (e.g., “Chain-of-Thought”) is the prominent approach for complex reasoning tasks, where more accurate reasoning chains typically improve downstream task performance. Recent literature discusses automatic methods to verify reasoning to evaluate and improve their correctness. However, no fine-grained step-level datasets are available to enable thorough evaluation of such verification methods, hindering progress in this direction. We introduce REVEAL: Reasoning Verification Evaluation, a dataset to benchmark automatic verifiers of complex Chain-of-Thought reasoning in open-domain question-answering settings. REVEAL includes comprehensive labels for the relevance, attribution to evidence passages, and logical correctness of each reasoning step in a language model’s answer, across a variety of datasets and state-of-the-art language models. Evaluation on REVEAL shows that verifiers struggle at verifying reasoning chains — in particular, verifying logical correctness and detecting contradictions. Available at https://reveal-dataset.github.io/.
View details
A Case for a Range of Acceptable Annotations
Olivia Rhinehart
Workshop on Subjectivity, Ambiguity and Disagreement in Crowdsourcing, AAAI (HCOMP 2018) (2018)
Preview abstract
Multi-way annotation is often used to ensure data quality in crowdsourced annotation tasks. Each item is annotated redundantly and the contributors’ judgments are converted into a single “ground truth” label or more complex annotation through a resolution technique (e.g., on the basis of majority or plurality). Recent crowdsourcing research has argued against the notion of a single “ground truth” annotation for items in semantically oriented tasks—that is, we should accept the aggregated judgments of a large pool of crowd contributors as “crowd truth.” While we agree that many semantically oriented tasks are inherently subjective, we do not go so far as to trust the judgments of the crowd in all cases. We recognize that there may be items for which there is truly only one acceptable response, and that there may be divergent annotations that are truly of unacceptable quality. We propose that there exists a class of annotations between these two categories that exhibit acceptable variation, which we define as the range of annotations for a given item that meet the standard of quality for a task. We illustrate acceptable variation within existing annotated data sets, including a labeled sound corpus and a medical relation extraction corpus. Finally, we explore the implications of acceptable variation on annotation task design and annotation quality evaluation.
View details
Community-Driven Crowdsourcing: Data Collection with Local Developers
Christina Funk
Ravindran Rajakumar
Linne Ha
Proceedings of the Eleventh International Conference on Language Resources and Evaluation (LREC 2018), European Language Resources Association (ELRA), Miyazaki, Japan, pp. 1606-1609
Preview abstract
We tested the viability of partnering with local developers to create custom annotation applications and to recruit and motivate crowd contributors from their communities to perform an annotation task consisting of the assignment of toxicity ratings to Wikipedia comments. We discuss the background of the project, the design of the community-driven approach, the developers’ execution of their applications and crowdsourcing programs, and the quantity, quality, and cost of judgments, as well as the influence of each application’s design on the outcomes. The community-driven approach resulted in local developers successfully creating four unique tools and collecting labeled data of sufficiently high quantity and quality. The creative approaches to the task presentation and crowdsourcing program design drew upon developers’ local knowledge of their own social networks, who also reported interest in the underlying problem that the data collection addresses. We consider the lessons that may be drawn from this project for future iterations of the community-driven approach.
View details
Points, Paths, and Playscapes: Large-scale Spatial Language Understanding Tasks Set in the Real World
Daphne Luong
Proceedings of the First International Workshop on Spatial Language Understanding, Association for Computational Linguistics, New Orleans, Louisiana, USA (2018), pp. 46-52
Preview abstract
Spatial language understanding is important for practical applications and as a building block for better abstract language understanding. Much progress has been made through work on understanding spatial relations and values in images and texts as well as on giving and following navigation instructions in restricted domains. We argue that the next big advances in spatial language understanding can be best supported by creating large-scale datasets that focus on points and paths based in the real world, and then extending these to create online, persistent playscapes that mix human and bot players. The bot players can begin play having undergone a prior training regime, but then must learn, evolve, and survive according to their depth of understanding of scenes, navigation, and interactions.
View details
Linguistic Wisdom from the Crowd
Nancy Chang
Russell Lee-Goldman
Crowdsourcing Breakthroughs for Language Technology Applications, AAAI Technical Report WS-15-24 (2016)
Preview abstract
Crowdsourcing for linguistic data typically aims to replicate expert annotations using simplified tasks. But an alternative goal—one that is especially relevant for research in the domains of language meaning and use—is to tap into people's rich experience as everyday users of language. Research in these areas has the potential to tell us a great deal about how language works, but designing annotation frameworks for crowdsourcing of this kind poses special challenges. In this paper we define and exemplify two approaches to linguistic data collection corresponding to these differing goals (model-driven and user-driven) and discuss some hybrid cases in which they overlap. We also describe some design principles and resolution techniques helpful for eliciting linguistic wisdom from the crowd.
View details