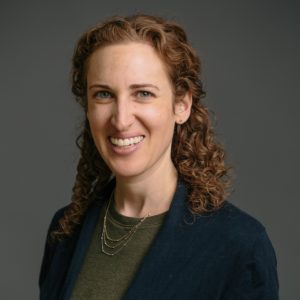
Meredith Ringel Morris
Meredith Ringel Morris is Director and Principal Scientist for Human-AI Interaction in Google DeepMind (formerly in Google Brain), conducting foundational research on Human-AI interaction and Human-Centered AI. Previously, she was Director of People + AI Research in Google Research's Responsible AI organization. She is also an Affiliate Professor at the University of Washington in The Paul G. Allen School of Computer Science & Engineering and in The Information School. Prior to joining Google Research, Dr. Morris was Research Area Manager for Interaction, Accessibility, and Mixed Reality at Microsoft Research, where she founded Microsoft’s Ability research group. Dr. Morris is an ACM Fellow and a member of the ACM SIGCHI Academy. Dr. Morris earned her Sc.B. in Computer Science from Brown University and her M.S. and Ph.D. in Computer Science from Stanford University.
Authored Publications
Sort By
Using large language models to accelerate communication for eye gaze typing users with ALS
Subhashini Venugopalan
Katie Seaver
Xiang Xiao
Katrin Tomanek
Sri Jalasutram
Ajit Narayanan
Bob MacDonald
Emily Kornman
Daniel Vance
Blair Casey
Steve Gleason
(2024)
From Provenance to Aberrations: Image Creator and Screen Reader User Perspectives on Alt Text for AI-Generated Images
Maitraye Das
Alexander J. Fiannaca
CHI Conference on Human Factors in Computing Systems (2024)
Help and The Social Construction of Access: A Case-Study from India
Vaishnav Kameswaran
Jerry Young Robinson
Nithya Sambasivan
Gaurav Aggarwal
Proceedings of ASSETS 2024, ACM (2024)
Characterizing Image Accessibility on Wikipedia across Languages
Elisa Kreiss
Krishna Srinivasan
Tiziano Piccardi
Jesus Adolfo Hermosillo
Michael S. Bernstein
Christopher Potts
Wiki Workshop 2023 (to appear)
Towards Semantically-Aware UI Design Tools: Design, Implementation, and Evaluation of Semantic Grouping Guidelines
Peitong Duan
Bjoern Hartmann
Karina Nguyen
Marti Hearst
ICML 2023 Workshop on Artificial Intelligence and Human-Computer Interaction (2023)
Practical Challenges for Investigating Abbreviation Strategies
Elisa Kreiss
CHI 2023 Workshop on Assistive Writing, ACM (2023) (to appear)
Generative Agents: Interactive Simulacra of Human Behavior
Joon Sung Park
Joseph C. O'Brien
Percy Liang
Michael Bernstein
Proceedings of UIST 2023, ACM (2023)