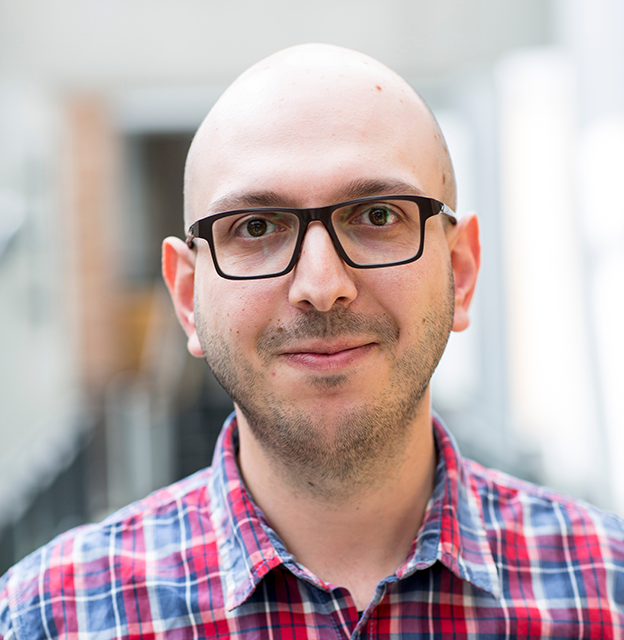
Konstantinos Rematas
I am a Research Scientist at Google Research, Zurich, working on computer vision and graphics. Previously, I was a research associate at University of Washington and I obtained my PhD from KU Leuven.
Research Areas
Authored Publications
Sort By
Preview abstract
We present a method for estimating neural scenes representations of objects given only a single image. The core of our method is the estimation of a geometric scaffold for the object and its use as a guide for the reconstruction of the underlying radiance field. Our formulation is based on a generative process that first maps a latent code to a voxelized shape, and then renders it to an image, with the object appearance being controlled by a second latent code. During inference, we optimize both the latent codes and the networks to fit an image of a new object.
The explicit disentanglement of shape and appearance, together with our effective pre-training, allows our model to be fine-tuned given a single image. We can then render new views in a geometrically consistent manner and they represent faithfully the input object. Additionally, our method is able to estimate the radiance field of images outside of the training domain (e.g. real photographs). Finally, the inferred geometric scaffold is itself an accurate estimate of the object's 3D shape.
We demonstrate in several experiments the effectiveness of our approach in both synthetic and real images.
View details
Preview abstract
We propose a method to detect and reconstruct multiple 3D objects from a single 2D image. The method is based on a key-point detector that localizes object centers in the image and then predicts all necessary properties for multi-object reconstruction: oriented 3D bounding
boxes, 3D shapes, and semantic class labels. By formulating 3D shape reconstruction as a classification problem, the method is agnostic
to specific shape representations. Specifically, the method uses CAD/mesh models, to reconstruct realistic and visually pleasing shapes (unlike e.g. voxel-based methods) and relies on point clouds and voxel representations to formulate the loss functions. Our method formulates 3D shape reconstruction as a classification problem, i.e. selecting among exemplar CAD models from the training set. This makes it agnostic to shape representations, and enables the reconstruction of realistic and visually-pleasing shapes (unlike e.g. voxel-based methods). At the same time, we also rely on point clouds and voxel representations derived from the CAD models to formulate the loss functions. In particular, a collision-loss penalizes intersecting objects, further increasing the realism of the reconstructed scenes. The method is a single-stage approach, thus it is orders-ofmagnitude faster than two-stage approaches, it is fully differentiable and end-to-end trainable.
View details
Preview abstract
We present a neural rendering framework that maps a
voxelized scene into a high quality image. Highly-textured
objects and scene element interactions are realistically rendered by our method, despite having a rough representation
as an input. Moreover, our approach allows controllable
rendering: geometric and appearance modifications in the
input are accurately propagated to the output. The user can
move, rotate and scale an object, change its appearance and
texture or modify a light’s position and all these edits are
represented in the final rendering. We demonstrate the effectiveness of our approach by rendering scenes with varying appearance, from single color per object to complex,
high-frequency textures. We show that our re-rendering network can generate very precise and detailed images that
capture the appearance of the input scene. Our experiments
also illustrate that our approach achieves more accurate
image synthesis results compared to alternatives and can
also handle low voxel grid resolutions. Finally, we show
how our neural rendering framework can be realistically
applied to real scenes with diverse set of objects.
View details