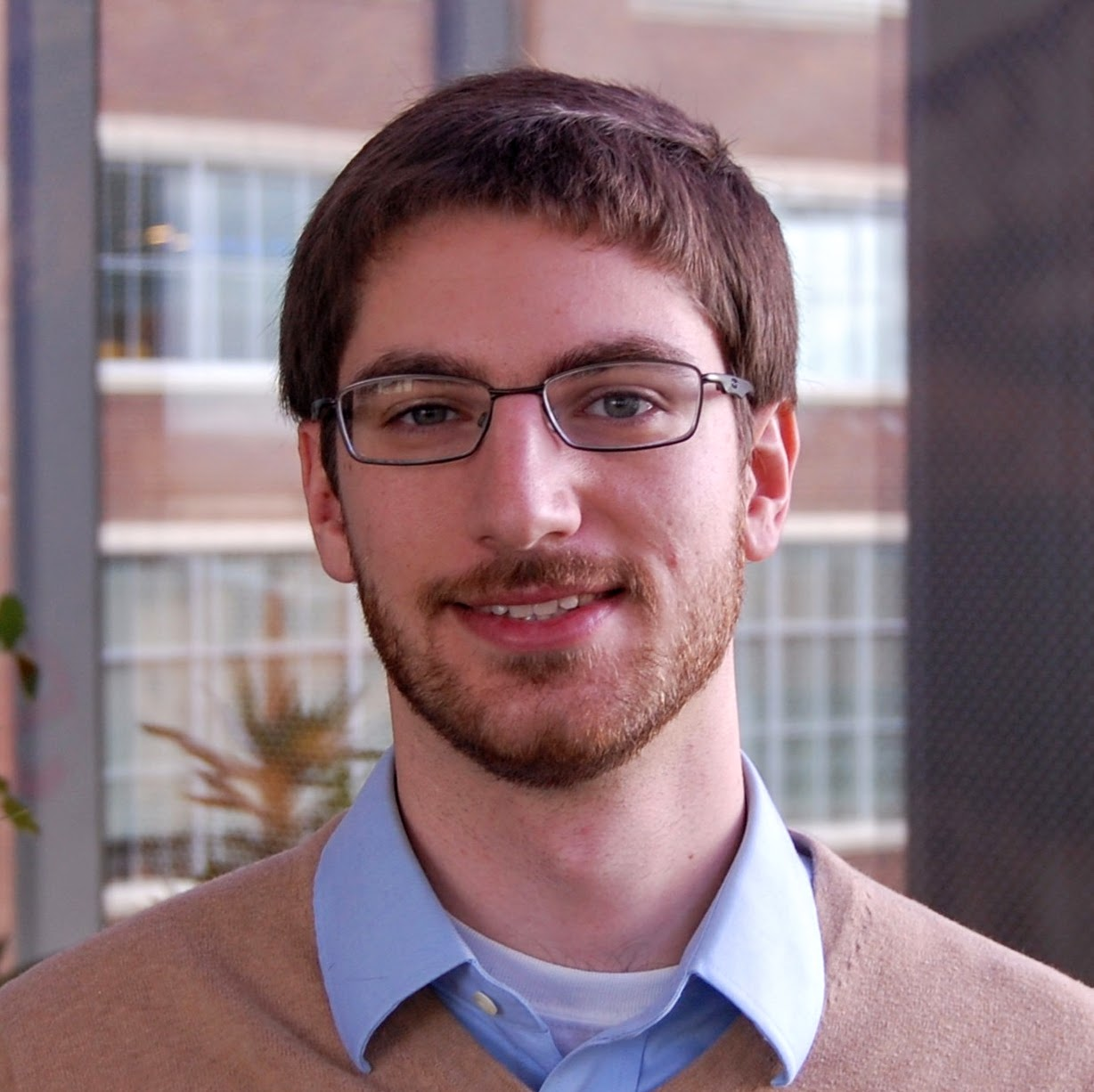
Joseph Roth
Research Areas
Authored Publications
Sort By
Google
AVA-ActiveSpeaker: An Audio-Visual Dataset for Active Speaker Detection
Ondrej Klejch
Radhika Marvin
Liat Kaver
Sharadh Ramaswamy
Arkadiusz Stopczynski
ICASSP, IEEE (2020)
Modeling Uncertainty with Hedged Instance Embedding
Seong Joon Oh
Jiyan Pan
ICLR 2019 (2019)
AVA-Speech: A Densely Labeled Dataset of Speech Activity in Movies
Liat Kaver
Radhika Marvin
Nathan Christopher Reale
Loretta Guarino Reid
Proceedings of Interspeech, 2018