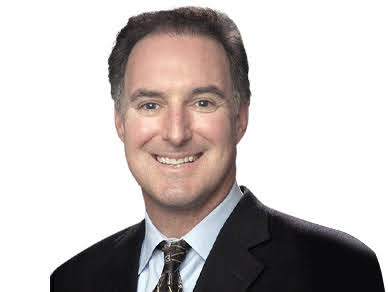
John Hernandez
Director, Head of Clinical Research Center of Excellence and Health Impact team
Authored Publications
Sort By
Evaluating PEARL - a Personalized Exercise Assistant using Reinforcement Learning (Walkmate Study)
Hulya Emir-Farinas
Martin Seneviratne
Amy Lee
Jim Taylor
Sriram Lakshminarasimhan
Emily Rosenzweig
OSF Registries (2024)
Preview abstract
Study protocol for PEARL Study: To evaluate the impact of two personalized nudging strategies delivered as pop-up notifications via the Fitbit app on user step count. Specifically, to personalize the following parameters of the pop-up notification system: message content, and timing (hr of the day)
View details
Analysis of objective and subjective sleep metrics and smartphone usage patterns
Conor Heneghan
Daniel McDuff
Ari Winbush
Nicholas Allen
Allen Jiang
Andrew Barakat
Logan Schneider
Benjamin Nelson
Ben Yetton
2024
Preview abstract
Analysis of objective and subjective sleep metrics and smartphone usage patterns
Conor Heneghan, , Daniel McDuff, Ari Winbush, Nicholas Allen, John Hernandez, Allen Jiang,, Andrew Barakat, Logan Schneider, Benjamin Nelson, Ben Yetton
Consumer Health Research Team, Google Inc.
Department of Psychology, University of Oregon
Verily Life Sciences
Department of Psychiatry, Harvard Medical School and Beth Israel Deaconess Medical Center
Introduction: The Digital Wellbeing Study is an IRB approved joint study between the University of Oregon and Google to investigate how smartphone usage interacts with objective and
subjective parameters of well-being such as sleep, exercise and stress. The study recruited a demographically diverse population who each wore a smartwatch and installed a smartphone app linked to the study. Participants completed demographic and health questionnaires including the PROMIS Sleep Disturbance (SD) Short Form. Aims of the study included (a) whether objective sleep duration was correlated with smartphone use, and (b) whether smartphone usage could predict the subjective self reported sleep instrument.
Methods: There was sufficient data from 7,499 users to conduct a population modeling analysis. An Ordinary Least Squares linear model was used as a predictor of each subject’s average total sleep time (TST) and their SD t-score. The inputs to the model included demographics, and population z-scored activity measures (steps, sedentary time, time driving, time at work, home and other locations, phone screen time, frequency of phone unlocks)
over seven days prior to the survey.
Results: The activity measures and baseline demographics could only explain a small amount of the overall variance in TST and SD (R^2=0.04 for TST and R^2=0.05 for SD). Phone screen
time was a statistically significant predictor of both TST (-8.19 mins, p< 0.001) and self-reported sleep disruption (0.611 t-score units, p< 0.001). The number of phone unlocks was a predictor of variability in TST (-3.33 mins, p< 0.001) suggesting that longer session times are correlated with greater TST variability. The effects are minimal (e.g., a subject who has one standard
deviation greater phone screen time than average would be predicted to only see a 2% reduction in TST, and a 0.6% increase in perceived sleep disturbance). Time driving and step count were
also minor predictors of SD and TST.
Conclusion: At a population level, average activity measures from wearables and smartphones such as steps, smartphone usage time, sedentary activity etc. are limited predictors of
objective sleep metrics such as Total Sleep Time, and subjective sleep metrics such as the PROMIS Sleep Disturbance t-score.
Support (if any): This research was funded by Google Inc.
View details
Evidence of Differences in Diurnal Electrodermal Patterns by Mental Health Status in Free-Living Data
Daniel McDuff
Isaac Galatzer-Levy
Seamus Thomson
Andrew Barakat
Conor Heneghan
Samy Abdel-Ghaffar
Jake Sunshine
Lindsey Sunden
Allen Jiang
Ari Winbush
Benjamin Nelson
Nicholas Allen
medRxiv (2024)
Preview abstract
Electrodermal activity (EDA) is a standardized measure of sympathetic arousal that has been linked to depression in laboratory experiments. However, the inability to measure EDA passively over time and in the real-world has limited conclusions that can be drawn about EDA as an indicator of mental health status outside of a controlled setting. Recent smartwatches have begun to incorporate wrist-worn continuous EDA sensors that enable longitudinal measurement in every-day life. This work presents the first example of passively collected, diurnal variations in EDA present in people with depression, anxiety and perceived stress. Subjects who were depressed had higher tonic EDA and heart rate, despite not engaging in greater physical activity, compared to those that were not depressed. EDA measurements showed differences between groups that were most prominent during the early morning. We did not observe amplitude or phase differences in the diurnal patterns.
View details
What Are The Odds? Language Models are Capable of Probabilistic Reasoning
Akshay Paruchuri
Shun Liao
Jake Sunshine
Tim Althoff
Daniel McDuff
arXiv (2024)
Preview abstract
Language models (LM) are capable of remarkably complex linguistic tasks; however, numerical reasoning is an area in which they frequently struggle. An important but rarely evaluated form of reasoning is understanding probability distributions. In this paper we focus on evaluating the probabilistic reasoning capabilities of LMs using idealized and real-world statistical distributions. We perform a systematic evaluation of state-of-the-art LMs on three tasks: estimating percentiles, drawing samples, and calculating probabilities. We find that zero-shot performance varies dramatically across different families of distributions and that performance can be improved significantly by using anchoring examples (shots) from within a distribution, or to a lesser extent across distributions within the same family. For real-world distributions, the absence of in-context examples can be substituted with context from which the LM can retrieve some statistics. Finally, we show that simply providing the mean and standard deviation of real-world distributions improves performance. To conduct this work, we developed a comprehensive benchmark distribution dataset with associated question-answer pairs that we release publicly, including questions about population health, climate, and finance.
View details
Sleep patterns and risk of chronic disease as measured by long-term monitoring with commercial wearable devices in the All of Us Research Program
Neil S. Zheng
Jeffrey Annis
Hiral Master
Lide Han
Karla Gleichauf
Melody Nasser
Peyton Coleman
Stacy Desine
Douglas M. Ruderfer
Logan D. Schneider
Evan L. Brittain
Nature Medicine (2024)
Preview abstract
Poor sleep health is associated with increased all-cause mortality and incidence of many chronic conditions. Previous studies have relied on cross-sectional and self-reported survey data or polysomnograms, which have limitations with respect to data granularity, sample size and longitudinal information. Here, using objectively measured, longitudinal sleep data from commercial wearable devices linked to electronic health record data from the All of Us Research Program, we show that sleep patterns, including sleep stages, duration and regularity, are associated with chronic disease incidence. Of the 6,785 participants included in this study, 71% were female, 84% self-identified as white and 71% had a college degree; the median age was 50.2 years (interquartile range = 35.7, 61.5) and the median sleep monitoring period was 4.5 years (2.5, 6.5). We found that rapid eye movement sleep and deep sleep were inversely associated with the odds of incident atrial fibrillation and that increased sleep irregularity was associated with increased odds of incident obesity, hyperlipidemia, hypertension, major depressive disorder and generalized anxiety disorder. Moreover, J-shaped associations were observed between average daily sleep duration and hypertension, major depressive disorder and generalized anxiety disorder. These findings show that sleep stages, duration and regularity are all important factors associated with chronic disease development and may inform evidence-based recommendations on healthy sleeping habits.
View details
Predicting subjective sleep impairment and disturbance from wearable sleep data
Conor Heneghan
Ben Yetton
Daniel McDuff
Nicholas Allen
Andrew Barakat
Allen Jiang
Logan Schneider
Benjamin Nelson
Ari Winbush
2024
Preview abstract
Introduction:
Wearables offer a scalable, passive and objective measure of sleep health. However, prior reported correlations (spearman) between subjective and wearable derived sleep measures have been modest (rS=0.3-0.46). We set out to determine if wearables adequately capture subjective feelings of sleep disturbance and impairment in a large, diverse ecologically valid sleep study.
Methods:
Subject data (n=2922, mean age= 45.4 (12.6), 74% female) came from the Digital Wellbeing Study: a joint study between the University of Oregon and Google to investigate how smartphone usage impacts well-being. Wearable (Fitbit) derived sleep metrics were summarized across the week prior to the administration of the PROMIS Sleep Disturbance (SD) and Sleep Related Impairment (SR) Short Form surveys. A series of stepwise OLS regressions were used to test the predictive power of each sleep metric over a baseline model of age and sex.
Results:
Sleep variables of total sleep time, resting heart rate, and the variability in total sleep time and restlessness (accelerometer based metric) improved both SI and SD above a baseline model (SIBaseline adjR2=0.087, SDBaseline adjR2=0.024). Deep (e.g. N3) minutes uniquely improved SI model fit, while longest wake length and total wake minutes improved SD fit. REM percent and normalized nightly heart rate did not improve model fit. The final model explained 12.9% of the variance of SI, and 8.4% of the variance of SD. The most predictive single sleep metric was the variability in total sleep time (adjR2=0.104) for SI, and total sleep time for SD (age & sex included). Fitbit’s composite “Sleep Score” was the single best predictor of SD when included in analysis (age and sex excluded).
Conclusion: As demonstrated in previous studies, wearable derived sleep metrics are modest predictors of perceived sleep disturbance or sleep related impairment. Composite metrics that include measures of sleep variability are recommended.
Support: This research was funded by Google Inc.
View details
Preview abstract
Background: Physical activity levels worldwide have declined over recent decades, with the average number of daily steps decreasing steadily since 1995. Given that physical inactivity is a major modifiable risk factor for chronic disease and mortality, increasing the level of physical activity is a clear opportunity to improve population health on a broad scale. The current study aims to assess the cost-effectiveness and budget impact of a Fitbit-based intervention among healthy, but insufficiently active, adults to quantify the potential clinical and economic value for a commercially insured population in the U.S. Methods: An economic model was developed to compare physical activity, health outcomes, costs, and quality-adjusted life-years (QALYs) associated with usual care and a Fitbit-based intervention that consists of a consumer wearable device alongside goal setting and feedback features provided in a companion software application. Improvement in physical activity was measured in terms of mean daily step count. The effects of increased daily step count were characterized as reduced short-term healthcare costs and decreased incidence of chronic diseases with corresponding improvement in health utility and reduced disease costs. Published literature, standardized costing resources, and data from a National Institutes of Health-funded research program were utilized. Cost-effectiveness and budget impact analyses were performed for a hypothetical cohort of middle-aged adults. Results: The base case cost-effectiveness results found the Fitbit intervention to be dominant (less costly and more effective) compared to usual care. Discounted 15-year incremental costs and QALYs were -$1,257 and 0.011, respectively. In probabilistic analyses, the Fitbit intervention was dominant in 93% of simulations and either dominant or cost-effective (defined as less than $150,000/QALY gained) in 99.4% of simulations. For budget impact analyses conducted from the perspective of a U.S. Commercial payer, the Fitbit intervention was estimated to save approximately $6.5-million dollars over 2 years and $8.5-million dollars over 5 years for a cohort of 8,000 participants. Although the economic analysis results were very robust, the short-term healthcare cost savings were the most uncertain in this population and warrant further research. Conclusions: There is abundant evidence documenting the benefits of wearable activity trackers when used to increase physical activity as measured by daily step counts. Our research provides additional health economic evidence supporting implementation of wearable-based interventions to improve population health and offers compelling support for payers to consider including wearable-based physical activity interventions as part of a comprehensive portfolio of preventive health offerings for their insured populations.
View details
Towards a Personal Health Large Language Model
Anastasiya Belyaeva
Nick Furlotte
Zhun Yang
Chace Lee
Erik Schenck
Yojan Patel
Jian Cui
Logan Schneider
Robby Bryant
Ryan Gomes
Allen Jiang
Roy Lee
Javier Perez
Jamie Rogers
Cathy Speed
Shyam Tailor
Megan Walker
Jeffrey Yu
Tim Althoff
Conor Heneghan
Mark Malhotra
Shwetak Patel
Shravya Shetty
Jiening Zhan
Yeswanth Subramanian
Daniel McDuff
arXiv (2024)
Preview abstract
Large language models (LLMs) can retrieve, reason over, and make inferences about a wide range of information. In health, most LLM efforts to date have focused on clinical tasks. However, mobile and wearable devices, which are rarely integrated into clinical tasks, provide a rich, continuous, and longitudinal source of data relevant for personal health monitoring. Here we present a new model, Personal Health Large Language Model (PH-LLM), a version of Gemini fine-tuned for text understanding and reasoning over numerical time-series personal health data for applications in sleep and fitness. To systematically evaluate PH-LLM, we created and curated three novel benchmark datasets that test 1) production of personalized insights and recommendations from measured sleep patterns, physical activity, and physiological responses, 2) expert domain knowledge, and 3) prediction of self-reported sleep quality outcomes. For the insights and recommendations tasks we created 857 case studies in sleep and fitness. These case studies, designed in collaboration with domain experts, represent real-world scenarios and highlight the model’s capabilities in understanding and coaching. Through comprehensive human and automatic evaluation of domain-specific rubrics, we observed that both Gemini Ultra 1.0 and PH-LLM are not statistically different from expert performance in fitness and, while experts remain superior for sleep, fine-tuning PH-LLM provided significant improvements in using relevant domain knowledge and personalizing information for sleep insights. To further assess expert domain knowledge, we evaluated PH-LLM performance on multiple choice question examinations in sleep medicine and fitness. PH-LLM achieved 79% on sleep (N=629 questions) and 88% on fitness (N=99 questions), both of which exceed average scores from a sample of human experts as well as benchmarks for receiving continuing credit in those domains. To enable PH-LLM to predict self-reported assessments of sleep quality, we trained the model to predict self-reported sleep disruption and sleep impairment outcomes from textual and multimodal encoding representations of wearable sensor data. We demonstrate that multimodal encoding is both necessary and sufficient to match performance of a suite of discriminative models to predict these outcomes. Although further development and evaluation are necessary in the safety-critical personal health domain, these results demonstrate both the broad knowledge base and capabilities of Gemini models and the benefit of contextualizing physiological data for personal health applications as done with PH-LLM.
View details
Preview abstract
This Op-ed is by leaders from the American Heart Association, Digital Medicine Society and Google involved in a Digital Medicine Society-sponsored project on digital measures for physical activity. The Op-ed summarizes evidence that the technology exists today to digitally measure physical activity in the broad population – and, by measuring it the right way, we can embrace it as the ‘6th vital sign’ and enter a new era of healthcare centered on proactive patient care.
View details
Cost-utility analysis of deep learning and trained human graders for diabetic retinopathy screening in a nationwide program
Attasit Srisubat
Kankamon Kittrongsiri
Sermsiri Sangroongruangsri
Chalida Khemvaranan
Jacqueline Shreibati
Fred Hersch
Prut Hanutsaha
Varis Ruamviboonsuk
Saowalak Turongkaravee
Rajiv Raman
Dr. Paisan Raumviboonsuk
Ophthalmology (2023)
Preview abstract
Introduction
Deep learning (DL) for screening diabetic retinopathy (DR) has the potential to address limited healthcare resources by enabling expanded access to healthcare. However, there is still limited health economic evaluation, particularly in low- and middle-income countries, on this subject to aid decision-making for DL adoption.
Methods
In the context of a middle-income country (MIC), using Thailand as a model, we constructed a decision tree-Markov hybrid model to estimate lifetime costs and outcomes of Thailand’s national DR screening program via DL and trained human graders (HG). We calculated the incremental cost-effectiveness ratio (ICER) between the two strategies. Sensitivity analyses were performed to probe the influence of modeling parameters.
Results
From a societal perspective, screening with DL was associated with a reduction in costs of ~ US$ 2.70, similar quality-adjusted life-years (QALY) of + 0.0043, and an incremental net monetary benefit of ~ US$ 24.10 in the base case. In sensitivity analysis, DL remained cost-effective even with a price increase from US$ 1.00 to US$ 4.00 per patient at a Thai willingness-to-pay threshold of ~ US$ 4.997 per QALY gained. When further incorporating recent findings suggesting improved compliance to treatment referral with DL, our analysis models effectiveness benefits of ~ US$ 20 to US$ 50 depending on compliance.
Conclusion
DR screening using DL in an MIC using Thailand as a model may result in societal cost-savings and similar health outcomes compared with HG. This study may provide an economic rationale to expand DL-based DR screening in MICs as an alternative solution for limited availability of skilled human resources for primary screening, particularly in MICs with similar prevalence of diabetes and low compliance to referrals for treatment.
View details